Performance of GAN-based augmentation for deep learning COVID-19 image classification
INTERNATIONAL WORKSHOP ON MACHINE LEARNING AND QUANTUM COMPUTING APPLICATIONS IN MEDICINE AND PHYSICS: WMLQ2022 AIP Conference Proceedings(2023)
摘要
The biggest challenge in the application of deep learning to the medical
domain is the availability of training data. Data augmentation is a typical
methodology used in machine learning when confronted with a limited data set.
In a classical approach image transformations i.e. rotations, cropping and
brightness changes are used. In this work, a StyleGAN2-ADA model of Generative
Adversarial Networks is trained on the limited COVID-19 chest X-ray image set.
After assessing the quality of generated images they are used to increase the
training data set improving its balance between classes. We consider the
multi-class classification problem of chest X-ray images including the COVID-19
positive class that hasn't been yet thoroughly explored in the literature.
Results of transfer learning-based classification of COVID-19 chest X-ray
images are presented. The performance of several deep convolutional neural
network models is compared. The impact on the detection performance of
classical image augmentations i.e. rotations, cropping, and brightness changes
are studied. Furthermore, classical image augmentation is compared with
GAN-based augmentation. The most accurate model is an EfficientNet-B0 with an
accuracy of 90.2 percent, trained on a dataset with a simple class balancing.
The GAN augmentation approach is found to be subpar to classical methods for
the considered dataset.
更多查看译文
AI 理解论文
溯源树
样例
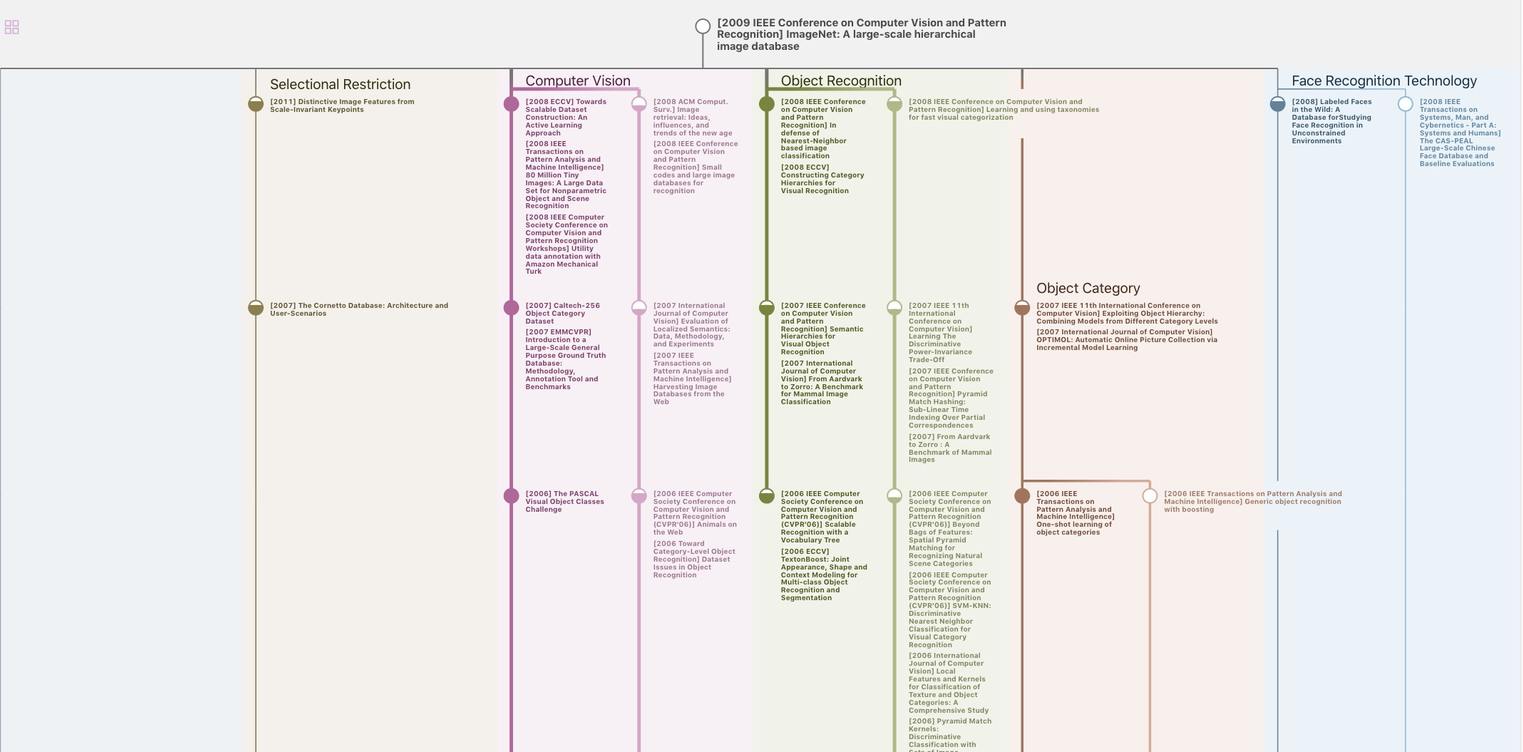
生成溯源树,研究论文发展脉络
Chat Paper
正在生成论文摘要