A Hyper-Network Based End-To-End Visual Servoing with Arbitrary Desired Poses
ICRA 2024(2024)
Abstract
Recently, several works achieve end-to-end visual servoing (VS) for robotic manipulation by replacing traditional controller with differentiable neural networks, but lose the ability to servo arbitrary desired poses. This letter proposes a differentiable architecture for arbitrary pose servoing: a hyper-network based neural controller (HPN-NC). To achieve this, HPN-NC consists of a hyper net and a low-level controller, where the hyper net learns to generate the parameters of the low-level controller and the controller uses the 2D keypoints error for control like traditional image-based visual servoing (IBVS). HPN-NC can complete 6 degree of freedom visual servoing with large initial offset. Taking advantage of the fully differentiable nature of HPN-NC, we provide a three-stage training procedure to servo real world objects. With self-supervised end-to-end training, the performance of the integrated model can be further improved in unseen scenes and the amount of manual annotations can be significantly reduced.
MoreTranslated text
Key words
Deep Learning in Grasping and Manipulation,Transfer Learning,Visual Servoing
AI Read Science
Must-Reading Tree
Example
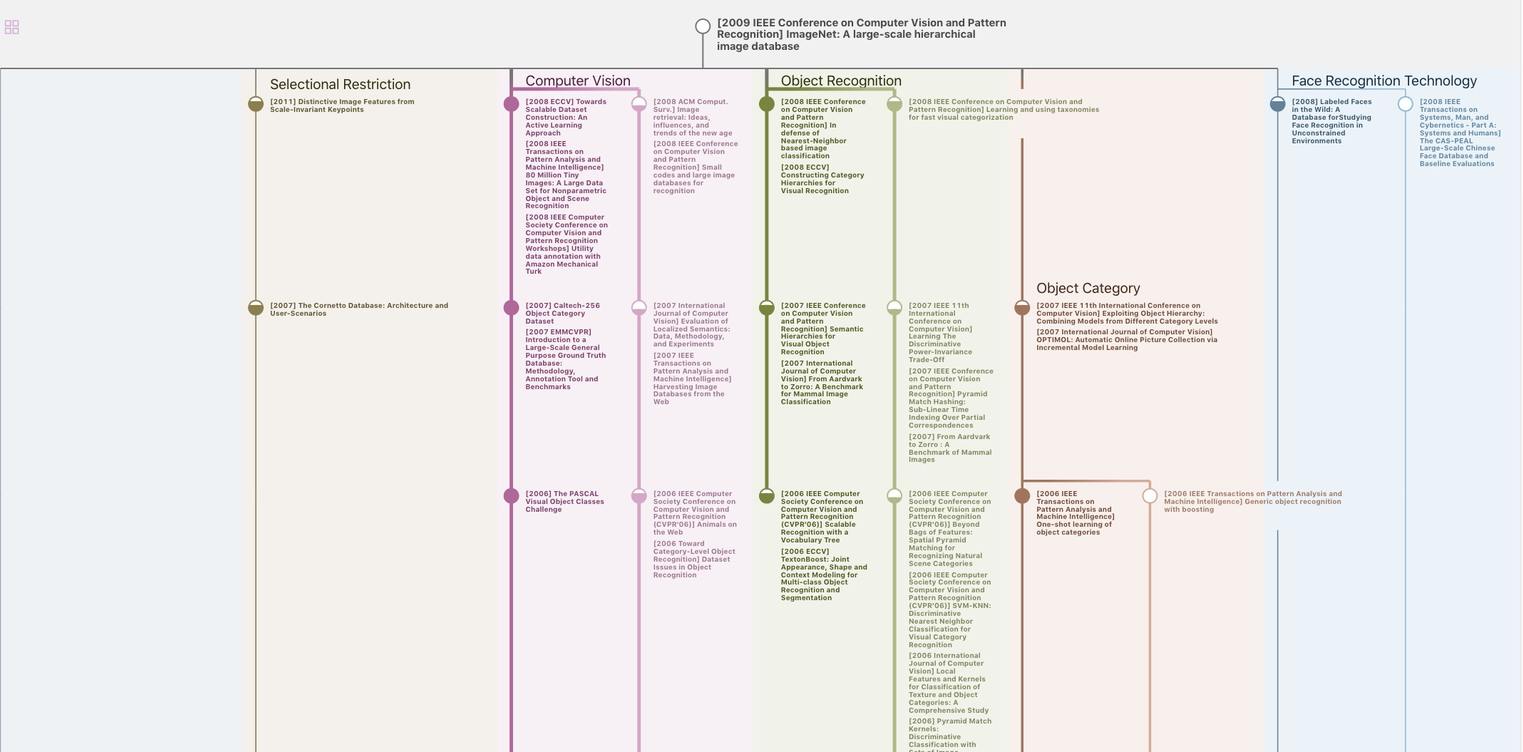
Generate MRT to find the research sequence of this paper
Chat Paper
Summary is being generated by the instructions you defined