Identification of Extended Emission Gamma-Ray-Bursts Candidates using Machine Learning
ASTROPHYSICAL JOURNAL(2023)
摘要
Gamma-ray bursts (GRBs) have been traditionally classified based on their duration. The increasing number of extended emission (EE) GRBs, lasting typically more than 2 seconds but with properties similar to those of a short GRBs, challenges the traditional classification criteria. In this work, we use the t-Distributed Stochastic Neighbor Embedding (t-SNE), a machine learning technique, to classify GRBs. We present the results for GRBs observed until July 2022 by the {\itshape Swift}/BAT instrument in all its energy bands. We show the effects of varying the learning rate and perplexity parameters as well as the benefit of pre-processing the data by a non-parametric noise reduction technique. % named {\sc FABADA}. Consistently with previous works, we show that the t-SNE method separates GRBs in two subgroups. We also show that EE GRBs reported by various authors under different criteria tend to cluster in a few regions of our t-SNE maps, and identify seven new EE GRB candidates by using the gamma-ray data provided by the automatic pipeline of {\itshape Swift}/BAT and the proximity with previously identified EE GRBs.
更多查看译文
关键词
machine learning,gamma-ray
AI 理解论文
溯源树
样例
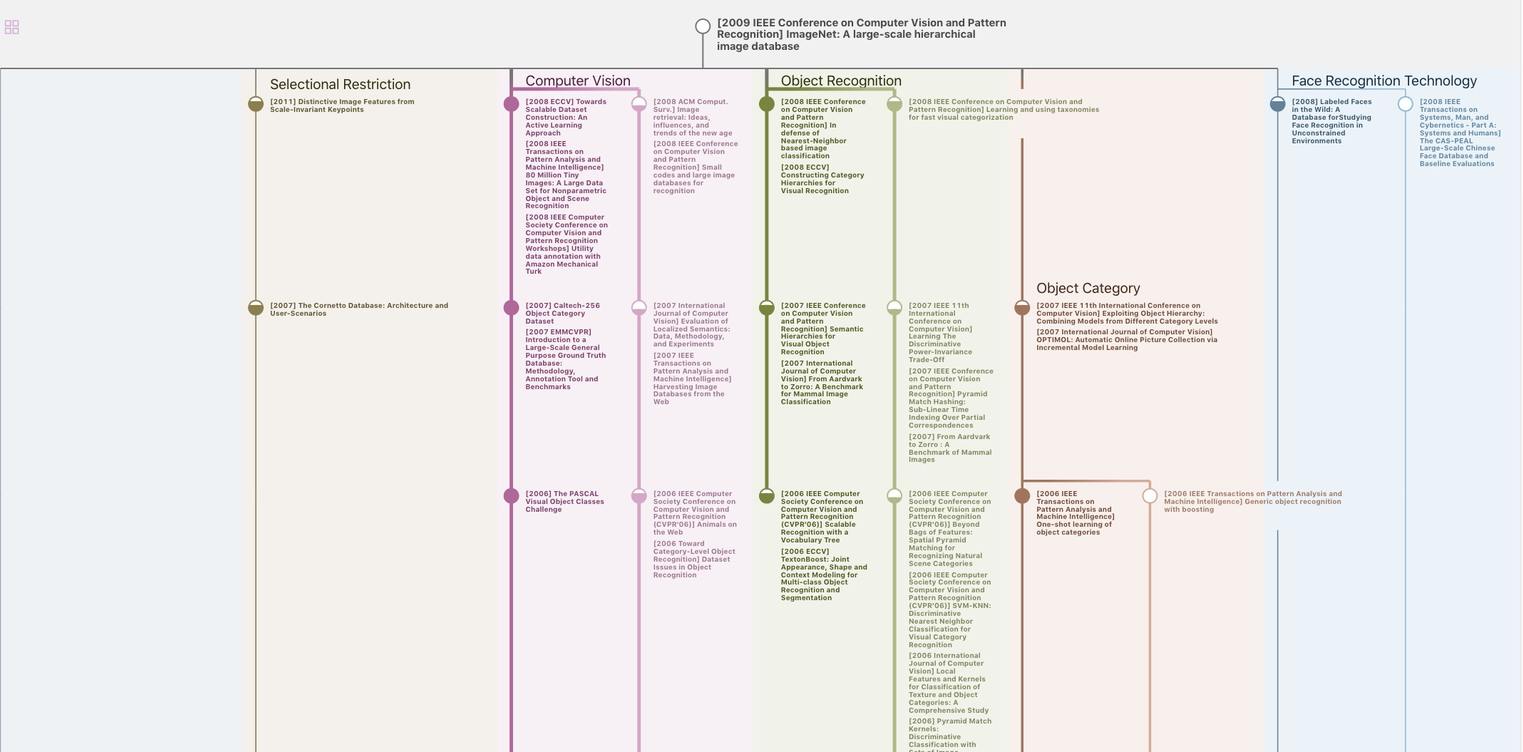
生成溯源树,研究论文发展脉络
Chat Paper
正在生成论文摘要