Hidden convexity, optimization, and algorithms on rotation matrices
arXiv (Cornell University)(2023)
摘要
This paper studies hidden convexity properties associated with constrained optimization problems over the set of rotation matrices $\text{SO}(n)$. Such problems are nonconvex due to the constraint $X \in \text{SO}(n)$. Nonetheless, we show that certain linear images of $\text{SO}(n)$ are convex, opening up the possibility for convex optimization algorithms with provable guarantees for these problems. Our main technical contributions show that any two-dimensional image of $\text{SO}(n)$ is convex and that the projection of $\text{SO}(n)$ onto its strict upper triangular entries is convex. These results allow us to construct exact convex reformulations for constrained optimization problems over $\text{SO}(n)$ with a single constraint or with constraints defined by low-rank matrices. Both of these results are optimal in a formal sense.
更多查看译文
关键词
rotation matrices,convexity,optimization,algorithms
AI 理解论文
溯源树
样例
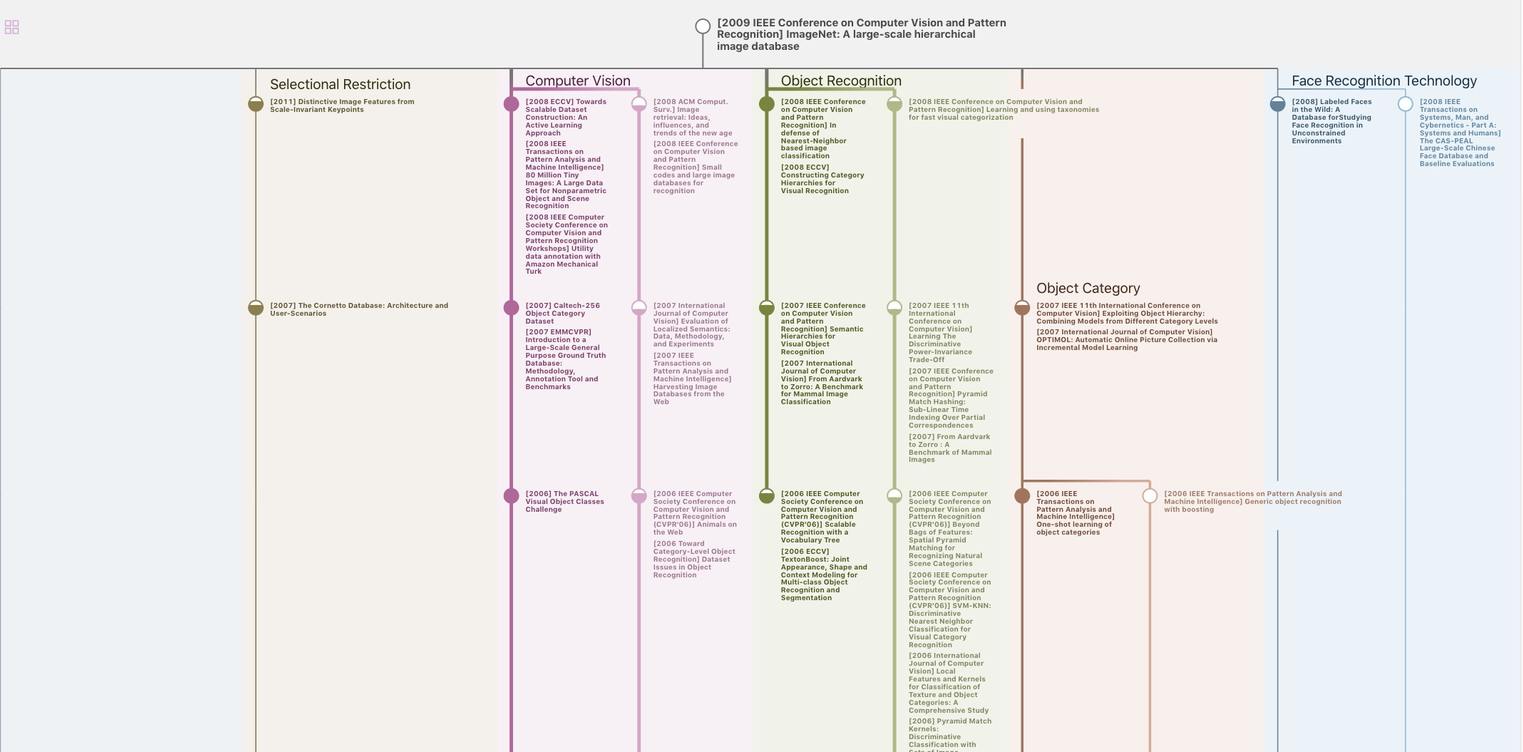
生成溯源树,研究论文发展脉络
Chat Paper
正在生成论文摘要