Attribute network models, stochastic approximation, and network sampling and ranking algorithms
arXiv (Cornell University)(2023)
摘要
We analyze dynamic random network models where younger vertices connect to older ones with probabilities proportional to their degrees as well as a propensity kernel governed by their attribute types. Using stochastic approximation techniques we show that, in the large network limit, such networks converge in the local weak sense to randomly stopped multitype branching processes whose explicit description allows for the derivation of asymptotics for a wide class of network functionals. These asymptotics imply that while degree distribution tail exponents depend on the attribute type (already derived by Jordan (2013)), Page-rank centrality scores have the \emph{same} tail exponent across attributes. Moreover, the mean behavior of the limiting Page-rank score distribution can be explicitly described and shown to depend on the attribute type. The limit results also give explicit formulae for the performance of various network sampling mechanisms. One surprising consequence is the efficacy of Page-rank and walk based network sampling schemes for directed networks in the setting of rare minorities. The results also allow one to evaluate the impact of various proposed mechanisms to increase degree centrality of minority attributes in the network, and to quantify the bias in inferring about the network from an observed sample. Further, we formalize the notion of resolvability of such models where, owing to propagation of chaos type phenomenon in the evolution dynamics for such models, one can set up a correspondence to models driven by continuous time branching process dynamics.
更多查看译文
关键词
network models,algorithms,stochastic approximation
AI 理解论文
溯源树
样例
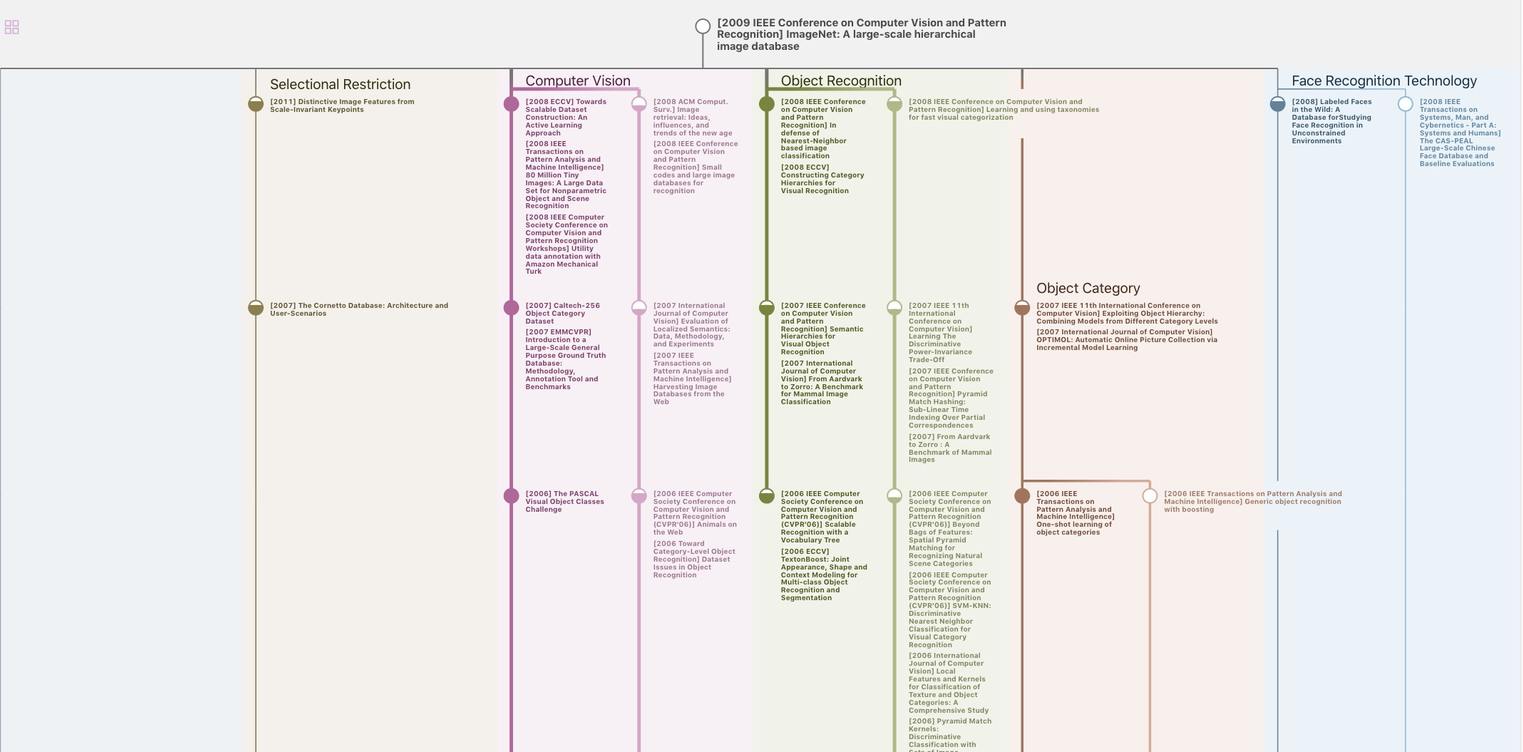
生成溯源树,研究论文发展脉络
Chat Paper
正在生成论文摘要