Bayesian averaging for ground state masses of atomic nuclei in a Machine Learning approach
FRONTIERS IN PHYSICS(2023)
摘要
We present global predictions of the ground state mass of atomic nuclei based on a novel Machine Learning (ML) algorithm. We combine precision nuclear experimental measurements together with theoretical predictions of unmeasured nuclei. This hybrid data set is used to train a probabilistic neural network. In addition to training on this data, a physics-based loss function is employed to help refine the solutions. The resultant Bayesian averaged predictions have excellent performance compared to the testing set and come with well-quantified uncertainties which are critical for contemporary scientific applications. We assess extrapolations of the model's predictions and estimate the growth of uncertainties in the region far from measurements.
更多查看译文
关键词
atomic nuclei, Bayesian averaging, binding energies and masses, Machine Learning-ML, nuclear physics, computational physics
AI 理解论文
溯源树
样例
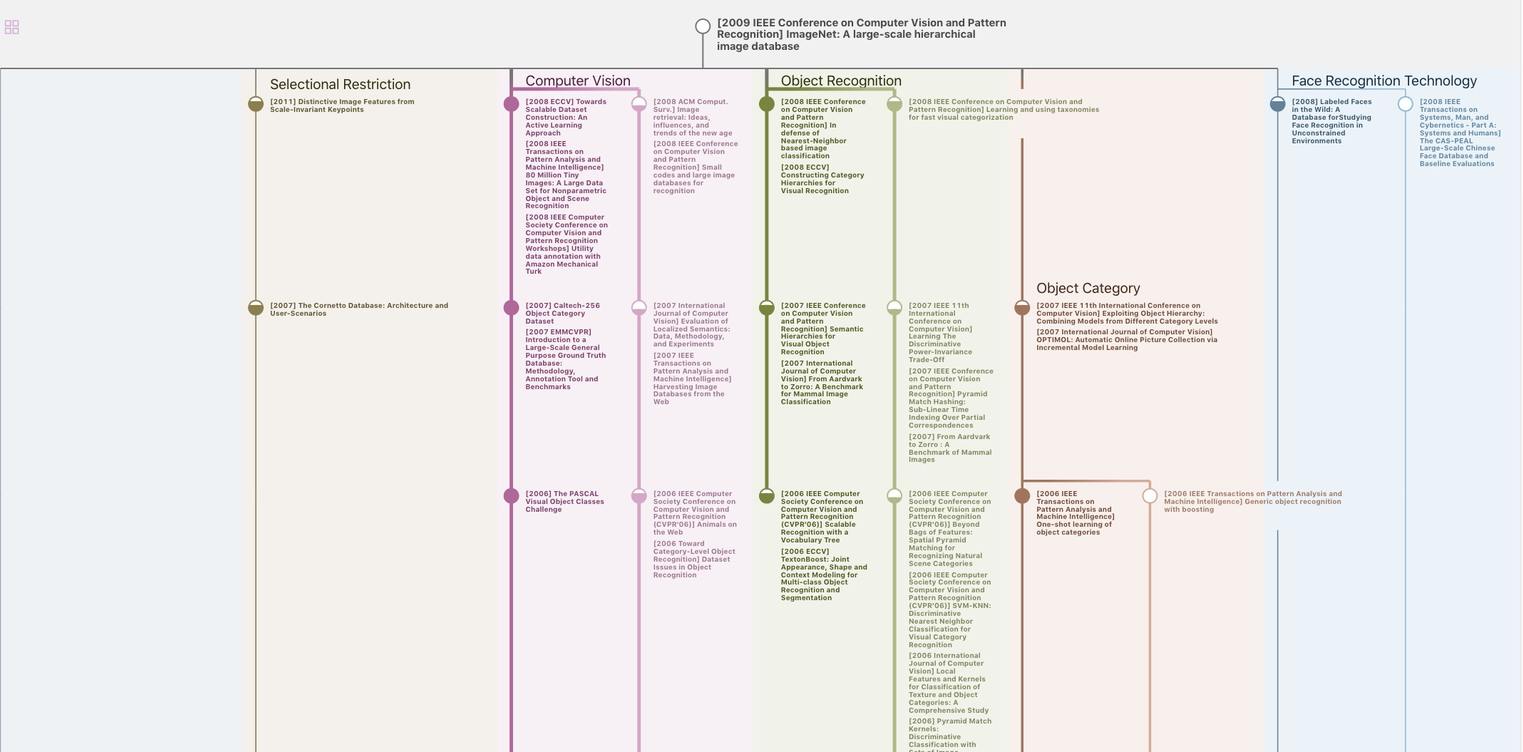
生成溯源树,研究论文发展脉络
Chat Paper
正在生成论文摘要