How can machine learning help in understanding the impact of climate change on crop yields?
Environmental Research Letters(2023)
摘要
Ordinary least squares linear regression (LR) has long been a popular choice among researchers interested in using historical data for estimating crop yield response to climate change. Today, the rapidly growing field of machine learning (ML) offers a wide range of advanced statistical tools that are increasingly being used for more accurate estimates of this relationship. This study compares LR to a popular ML technique called boosted regression trees (BRTs). We find that BRTs provide a significantly better prediction accuracy compared to various LR specifications, including those fitting quadratic and piece-wise linear functions. BRTs are also able to identify break points where the relationship between climate and yield undergoes significant shifts (for example, increasing yields with precipitation followed by a plateauing of the relationship beyond a certain point). Tests we performed with synthetically simulated climate and crop yield data showed that BRTs can automatically account for not only spatial variation in climate-yield relationships, but also interactions between different variables that affect crop yields. We then used both statistical techniques to estimate the influence of historical climate change on rice, wheat, and pearl millet in India. BRTs predicted a considerably smaller negative impact compared to LR. This may be an artifact of BRTs conflating time and climate variables, signaling a potential weakness of models with excessively flexible functional forms for inferring climate impacts on agriculture. Our findings thus suggest caution while interpreting the results from single-model analyses, especially in regions with highly varied climate and agricultural practices.
更多查看译文
关键词
statistical crop models,machine learning,boosted regression trees,crop yield,climate change,India
AI 理解论文
溯源树
样例
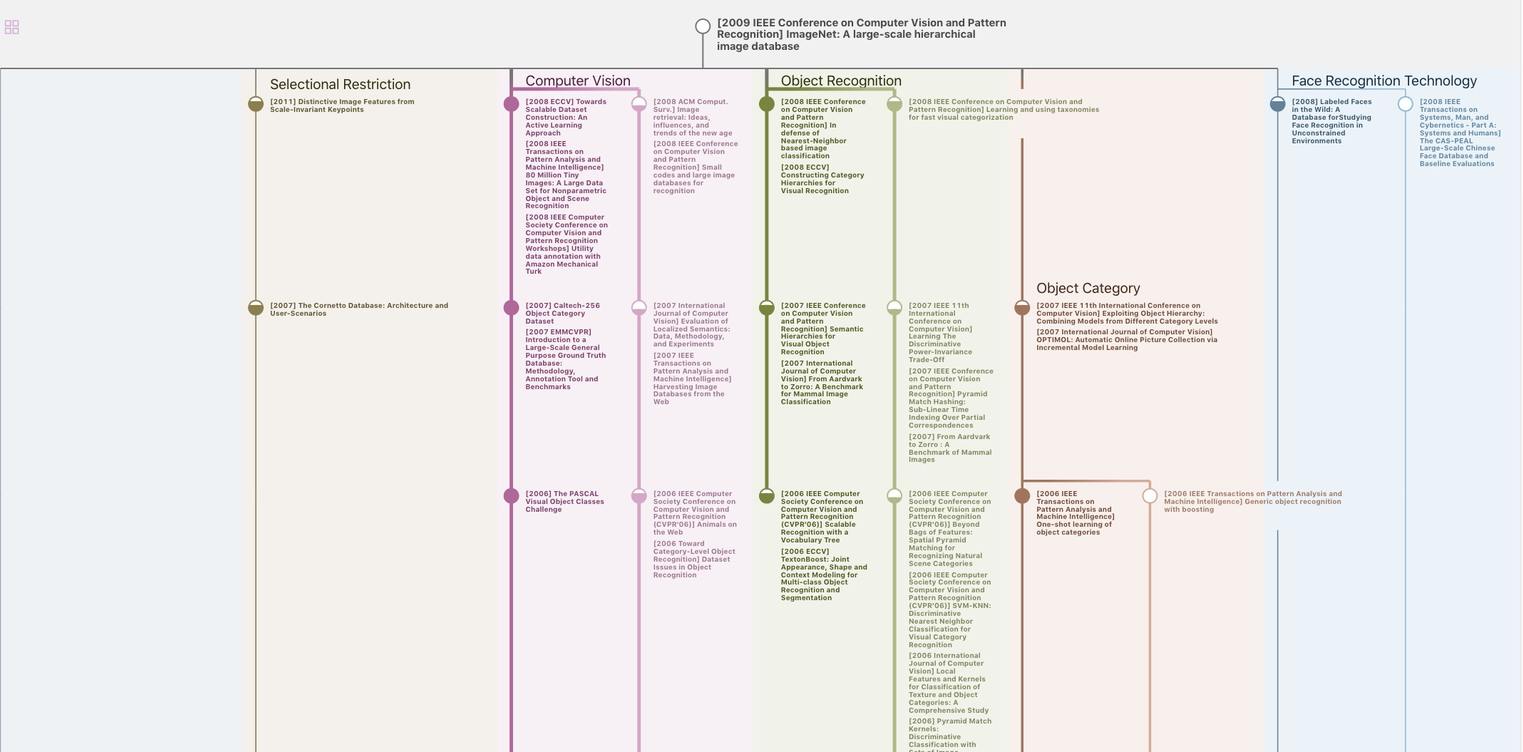
生成溯源树,研究论文发展脉络
Chat Paper
正在生成论文摘要