Deep reinforcement learning based optimal scheduling of active distribution system considering distributed generation, energy storage and flexible load
Energy(2023)
摘要
The increasing integration of distributed resources, such as distributed generations (DGs), energy storage systems (ESSs), and flexible loads (FLs), has ushered in a new era for the active distribution system (ADS), characterized by more reliable, economical, and low-carbon. Nonetheless, with the increase in number and variety, how to realize self-consistent and self-optimal operation among these distributed resources has become major challenge for ADS. In this paper, a multi-agent deep reinforcement learning (MADRL) based algorithm with strategic goals of the real-time optimal scheduling of ADS is proposed, in which the uncertainty of renewable generations (RDGs), loads and electricity price are considered. The control variables contain the active and reactive power of dispatchable thermal DGs, the reactive power of photovoltaic and wind turbine DGs, the exchange power of ESSs, and the demand response (DR) of FLs. Besides, the region ownership of distributed resources is considered in our MADRL framework to resolve the partitioned optimization problem in large-scale ADS. Finally, the effectiveness and superiority of the proposed algorithm are demonstrated on the 33-node and 152-node active distribution system, including the terms of cost-effective and uncertainty adaptation.
更多查看译文
关键词
Active distribution system,Distributed generation,Deep reinforcement learning,Optimal scheduling
AI 理解论文
溯源树
样例
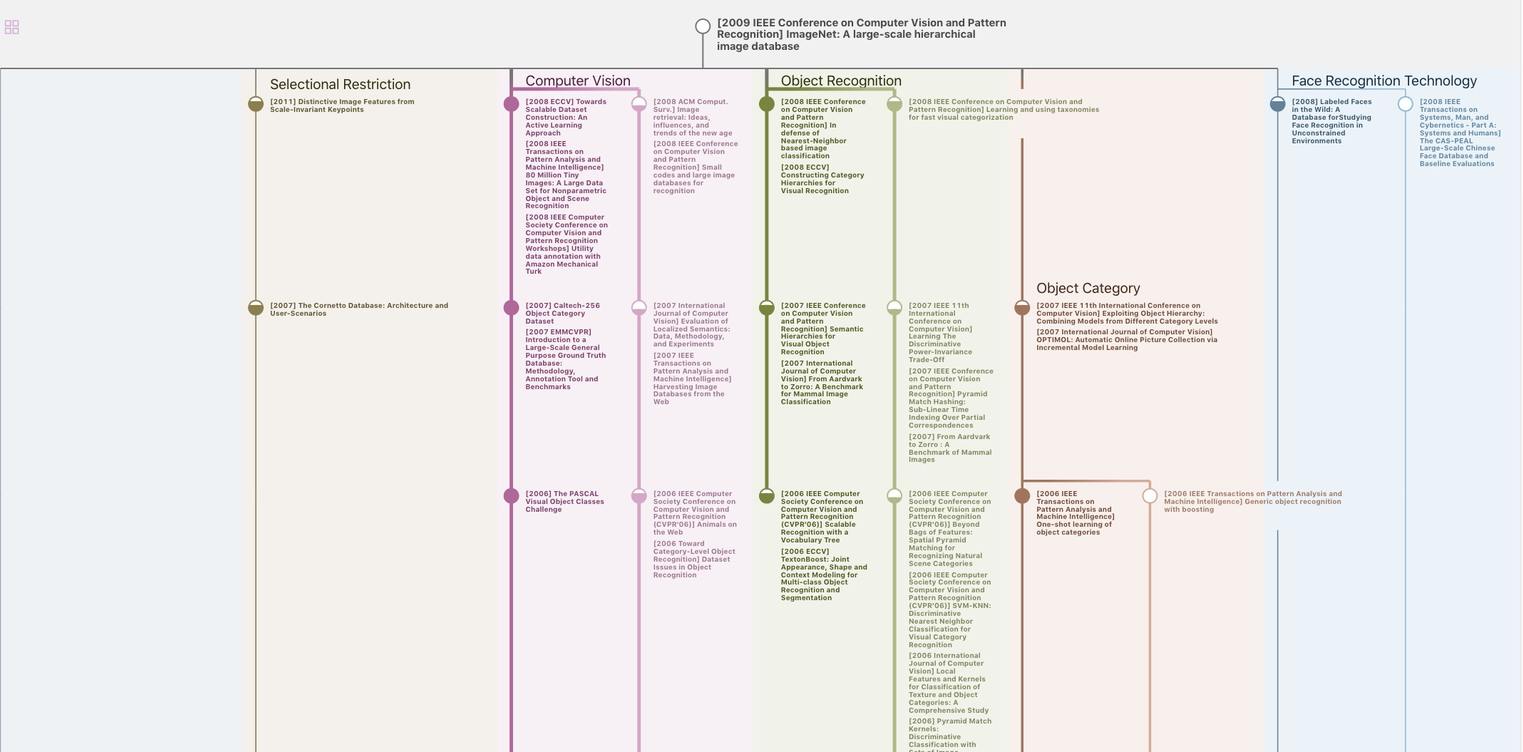
生成溯源树,研究论文发展脉络
Chat Paper
正在生成论文摘要