Random health indicator and shallow neural network based robust capacity estimation for lithium-ion batteries with different fast charging protocols
Energy(2023)
Abstract
Different fast charging protocols will cause diverse degradation rates of lithium-ion batteries (LIBs), which makes accurate health prognostic challenging. Considering the disorder of fast charging protocols and charging intervals, a random health indicator and shallow neural network based robust capacity estimation approach is developed. First, partial raw charging data of LIBs is used to extract random health indicator based on a simple moving window without any manual feature engineering. Specifically, two health indicators with 20 and 100 data points are designed for capacity estimation, the charging start voltage can be any value above 3 V, which satisfies most charging scenarios in the real-world application. Second, the shallow neural network with only 3 convolutional sections is employed for capacity estimation modeling, which performs high accuracy and strong robustness against the influence of different fast charging protocols on battery degradation. Finally, several prevalent networks are used for comparison, which demonstrates the superior performance of proposed method. The experimental test results show that root mean square errors (RMSEs) of less than 1.9% and mean absolute errors (MAEs) of less than 1.3% can be obtained using the charging data in daily charging interval, and the RMSEs and MAEs can be further decreased to less than 0.93% and 0.61% respectively by using charging data in higher voltage interval.
MoreTranslated text
Key words
Lithium -ion batteries,Capacity estimation,Fast charging,Shallow neural network,Hierarchical scenarios
AI Read Science
Must-Reading Tree
Example
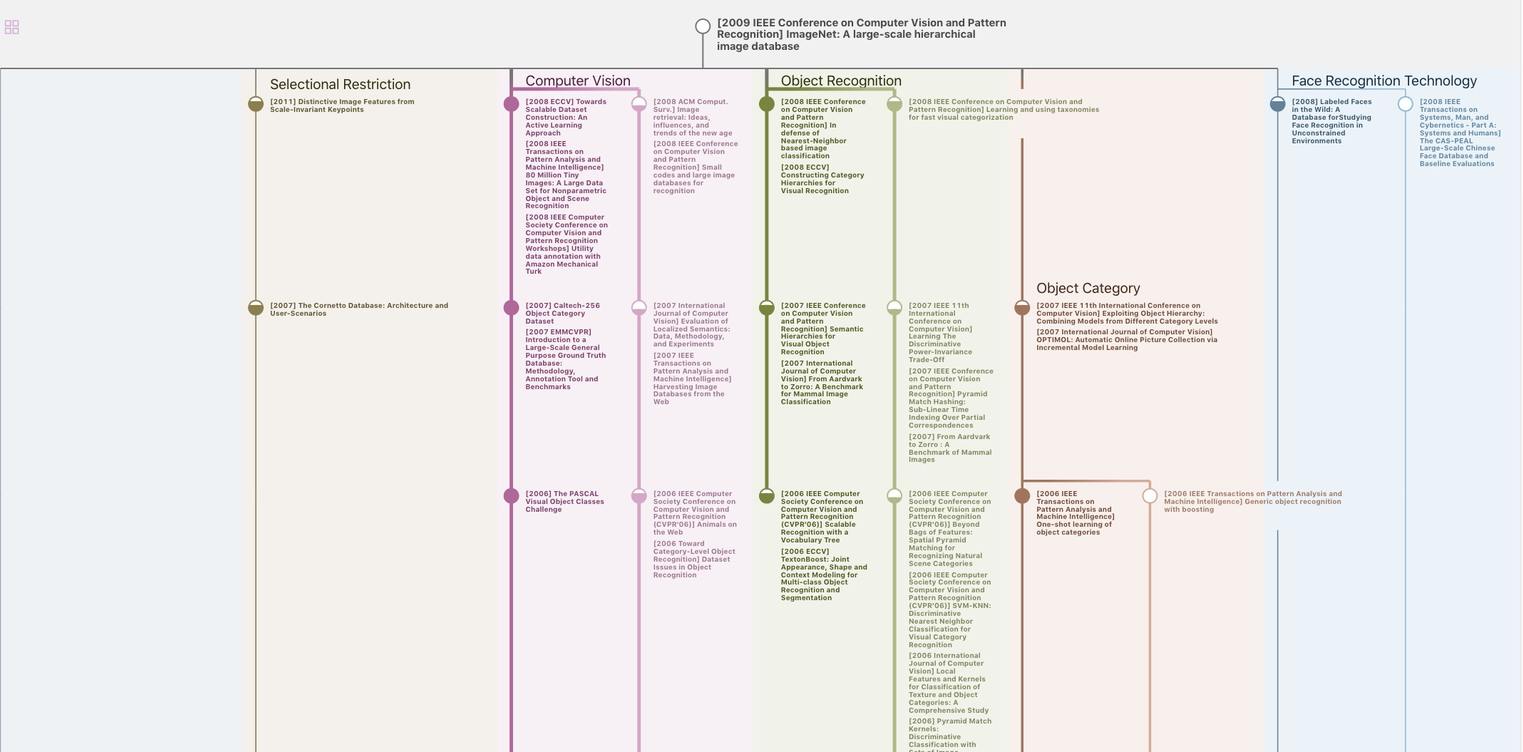
Generate MRT to find the research sequence of this paper
Chat Paper
Summary is being generated by the instructions you defined