Sequence similarity governs generalizability of de novo deep learning models for RNA secondary structure prediction
PLoS computational biology(2023)
摘要
Making no use of physical laws or co-evolutionary information, de novo deep learning (DL) models for RNA secondary structure prediction have achieved far superior performances than traditional algorithms. However, their statistical underpinning raises the crucial question of generalizability. We present a quantitative study of the performance and generalizability of a series of de novo DL models, with a minimal two-module architecture and no post-processing, under varied similarities between seen and unseen sequences. Our models demonstrate excellent expressive capacities and outperform existing methods on common benchmark datasets. However, model generalizability, i.e., the performance gap between the seen and unseen sets, degrades rapidly as the sequence similarity decreases. The same trends are observed from several recent DL and machine learning models. And an inverse correlation between performance and generalizability is revealed collectively across all learning-based models with wide-ranging architectures and sizes. We further quantitate how generalizability depends on sequence and structure identity scores via pairwise alignment, providing unique quantitative insights into the limitations of statistical learning. Generalizability thus poses a major hurdle for deploying de novo DL models in practice and various pathways for future advances are discussed. Author summaryLearning-based de novo models of RNA secondary structures critically rely on training data to associate sequences with structures. The practical utility of such models thus hinges on not only training performances but generalizations over unseen sequences. Model generalizability, however, remains poorly understood. By delineating sequence similarity at three distinct levels, we develop a series of DL models and evaluate their performance and generalizability, as well as several current DL and machine learning models. First establishing the decisive role of sequence similarity in generalizability, we further quantitate their dependencies via pairwise sequence and structure alignment. The gained quantitative insights make valuable guidelines for deploying DL models in practice and advocate RNA secondary structure as a unique platform for developing generalizable learning-based models.
更多查看译文
关键词
rna,de novo,deep learning models,deep learning,secondary structure
AI 理解论文
溯源树
样例
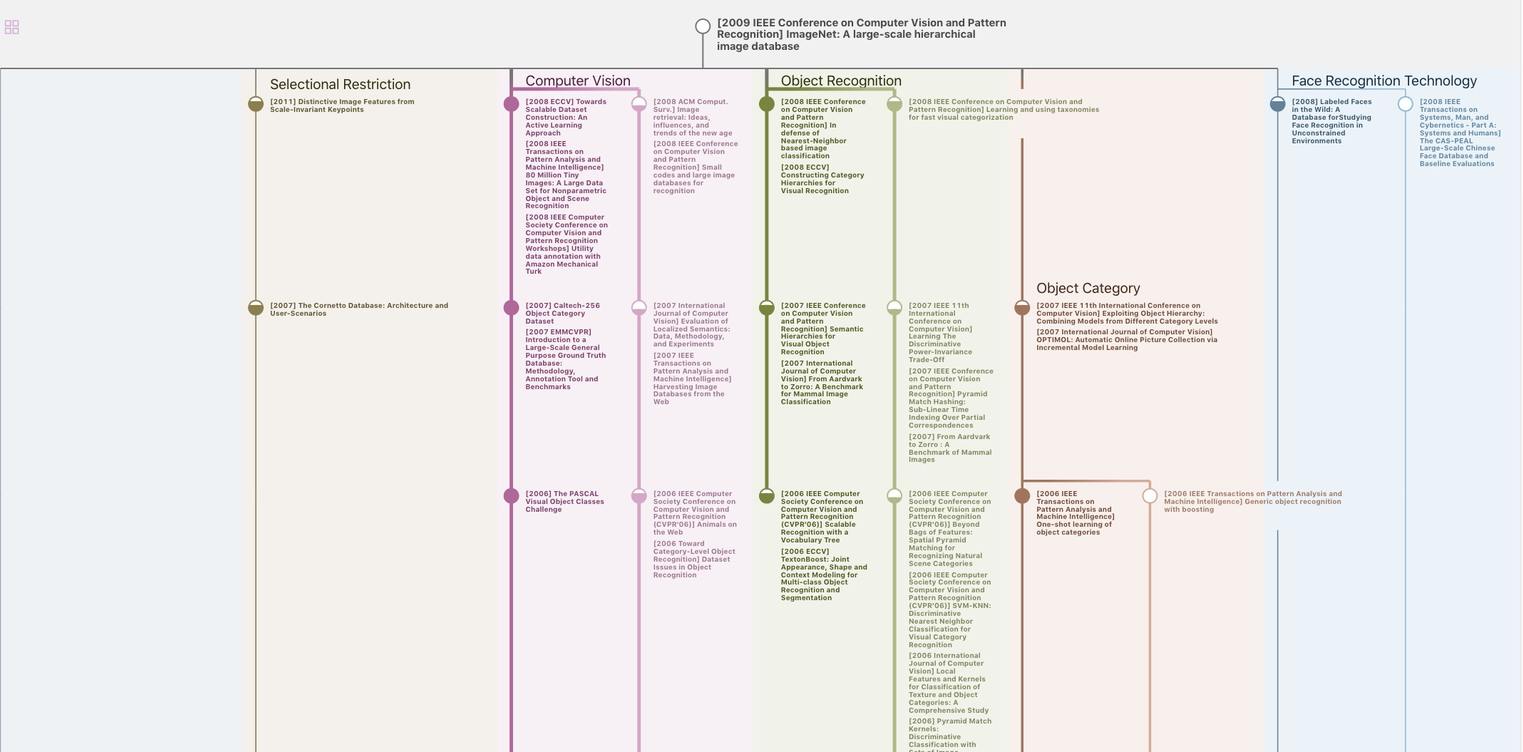
生成溯源树,研究论文发展脉络
Chat Paper
正在生成论文摘要