A training program to reduce reader search errors for liver metastasis detection in CT.
Proceedings of SPIE--the International Society for Optical Engineering(2023)
摘要
Detection of low contrast liver metastases varies between radiologists. Training may improve performance for lower-performing readers and reduce inter-radiologist variability. We recruited 31 radiologists (15 trainees, 8 non-abdominal staff, and 8 abdominal staff) to participate in four separate reading sessions: pre-test, search training, classification training, and post-test. In the pre-test, each radiologist interpreted 40 liver CT exams containing 91 metastases, circumscribed suspected hepatic metastases while under eye tracker observation, and rated confidence. In search training, radiologists interpreted a separate set of 30 liver CT exams while receiving eye tracker feedback and after coaching to increase use of coronal reformations, interpretation time, and use of liver windows. In classification training, radiologists interpreted up to 100 liver CT image patches, most with benign or malignant lesions, and compared their annotations to ground truth. Post-test was identical to pre-test. Between pre- and post-test, sensitivity increased by 2.8% (p = 0.01) but AUC did not change significantly. Missed metastases were classified as search errors (<2 seconds gaze time) or classification errors (>2 seconds gaze time) using the eye tracker. Out of 2775 possible detections, search errors decreased (10.8% to 8.1%; p < 0.01) but classification errors were unchanged (5.7% vs 5.7%). When stratified by difficulty, easier metastases showed larger reductions in search errors: for metastases with average sensitivity of 0-50%, 50-90%, and 90-100%, reductions in search errors were 16%, 35%, and 58%, respectively. The training program studied here may be able to improve radiologist performance by reducing errors but not classification errors.
更多查看译文
关键词
Eye tracking,low contrast detectability,reader performance,reader training
AI 理解论文
溯源树
样例
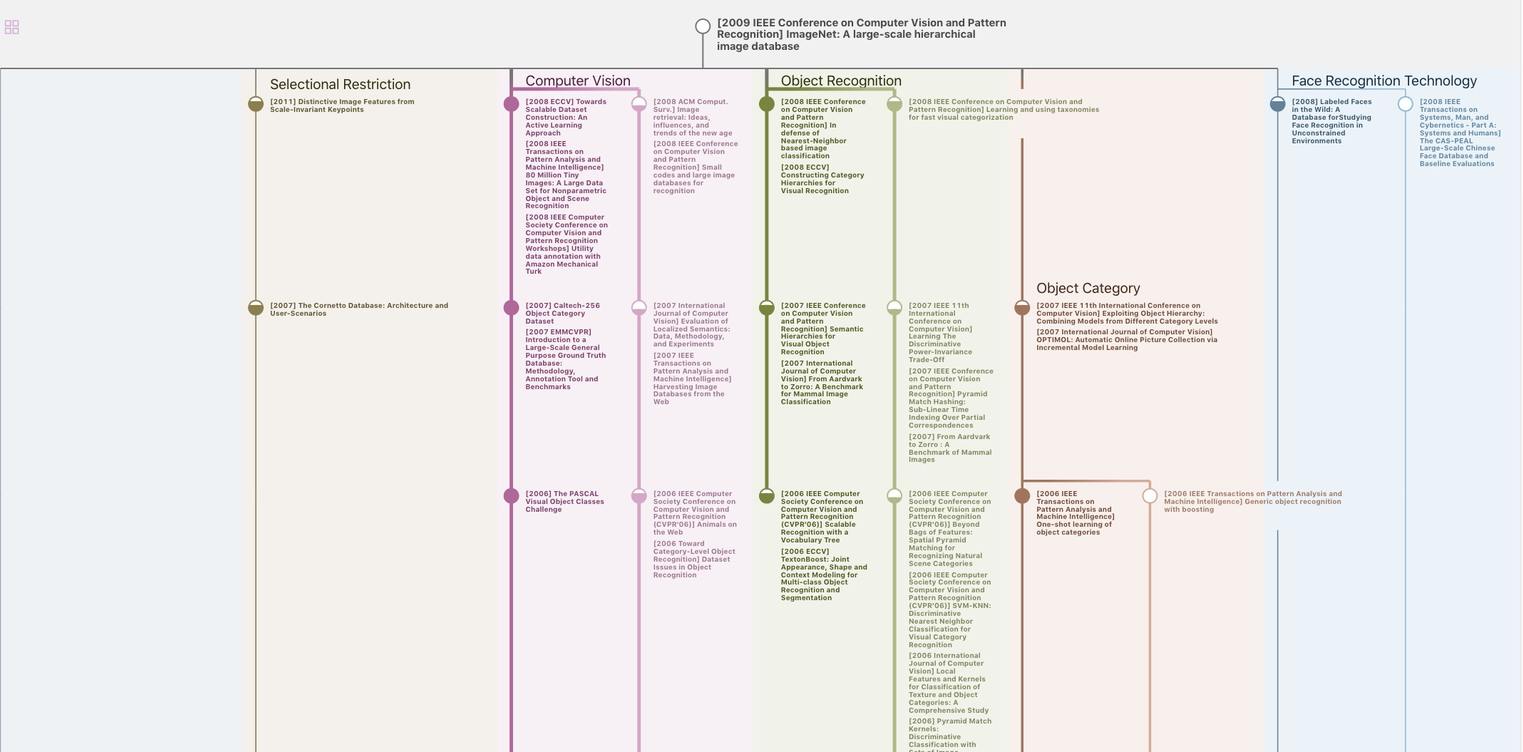
生成溯源树,研究论文发展脉络
Chat Paper
正在生成论文摘要