Feature extraction and prediction of fine particulate matter (PM2.5) chemical constituents using four machine learning models
Expert Systems with Applications(2023)
摘要
The concentrations of fine particulate matter (PM2.5) constituents, which are very important and essential in-formation for the identification of air pollution sources, were predicted at three sites (Seoul, Ulsan, Baeng-nyeong) in South Korea between 2016 and 2018 using four machine learning (ML) models: generative adversarial imputation network (GAIN), fully connected deep neural network (FCDNN), random forest (RF), and k-nearest neighbor (kNN). 3 PM2.5 constituent groups, namely 8 ions, 2 carbons, and 15 trace elements, were targeted for prediction. The latest hyperparameter optimization techniques were used to learn air pollution characteristics from ambient PM2.5-related information, such as time, meteorology, and air pollutant concen-trations. We compared the feature extraction abilities of the four models. The prediction accuracy identified by the coefficient of determination (R2) between prediction and observation was highest in GAIN, followed by FCDNN and RF or kNN. On availability of data on the time, air pollutant concentrations, and/or meteorology, simultaneously missed 20 % data of all PM2.5 constituent groups were predicted, with R2 = 0.897, 0.861, 0.785, and 0.801 by the GAIN, FCDNN, RF, and kNN, respectively. As missing ratios (20 %, 40 %, 60 %, 80 %) of input data increased, prediction accuracy decreased in the four models and was predominantly more noticeable in GAIN and kNN. As the available period of data increased, prediction accuracy increased in GAIN and FCDNN. Trace elements were predicted with the lowest R2 in all models among the target constituent groups. Study sites with more emission sources showed lower prediction accuracy, resulting in the highest R2 in Baengnyeong island and the lowest in Ulsan. According to the current findings, ML models can be used to evaluate various air pollution issues for which data is missing.
更多查看译文
关键词
PM2,5 chemical constituents,Machine learning,Generative adversarial imputation networks,Fully connected deep neural network,Random forest,K-nearest neighbor
AI 理解论文
溯源树
样例
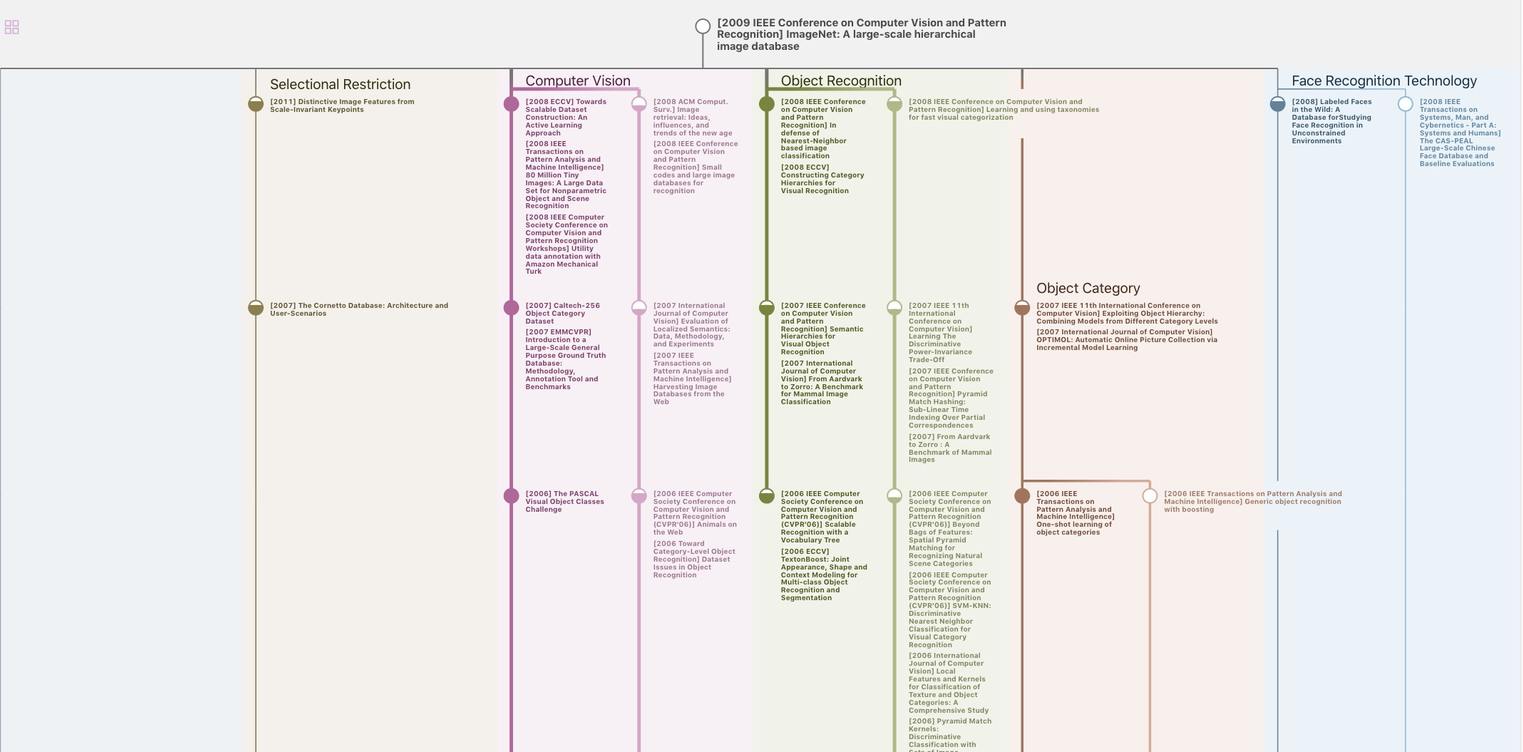
生成溯源树,研究论文发展脉络
Chat Paper
正在生成论文摘要