Compositional Probabilistic and Causal Inference using Tractable Circuit Models
CoRR(2023)
摘要
Probabilistic circuits (PCs) are a class of tractable probabilistic models, which admit efficient inference routines depending on their structural properties. In this paper, we introduce md-vtrees, a novel structural formulation of (marginal) determinism in structured decomposable PCs, which generalizes previously proposed classes such as probabilistic sentential decision diagrams. Crucially, we show how mdvtrees can be used to derive tractability conditions and efficient algorithms for advanced inference queries expressed as arbitrary compositions of basic probabilistic operations, such as marginalization, multiplication and reciprocals, in a sound and generalizable manner. In particular, we derive the first polytime algorithms for causal inference queries such as backdoor adjustment on PCs. As a practical instantiation of the framework, we propose MDNets, a novel PC architecture using md-vtrees, and empirically demonstrate their application to causal inference.
更多查看译文
关键词
causal inference,tractable circuit,models
AI 理解论文
溯源树
样例
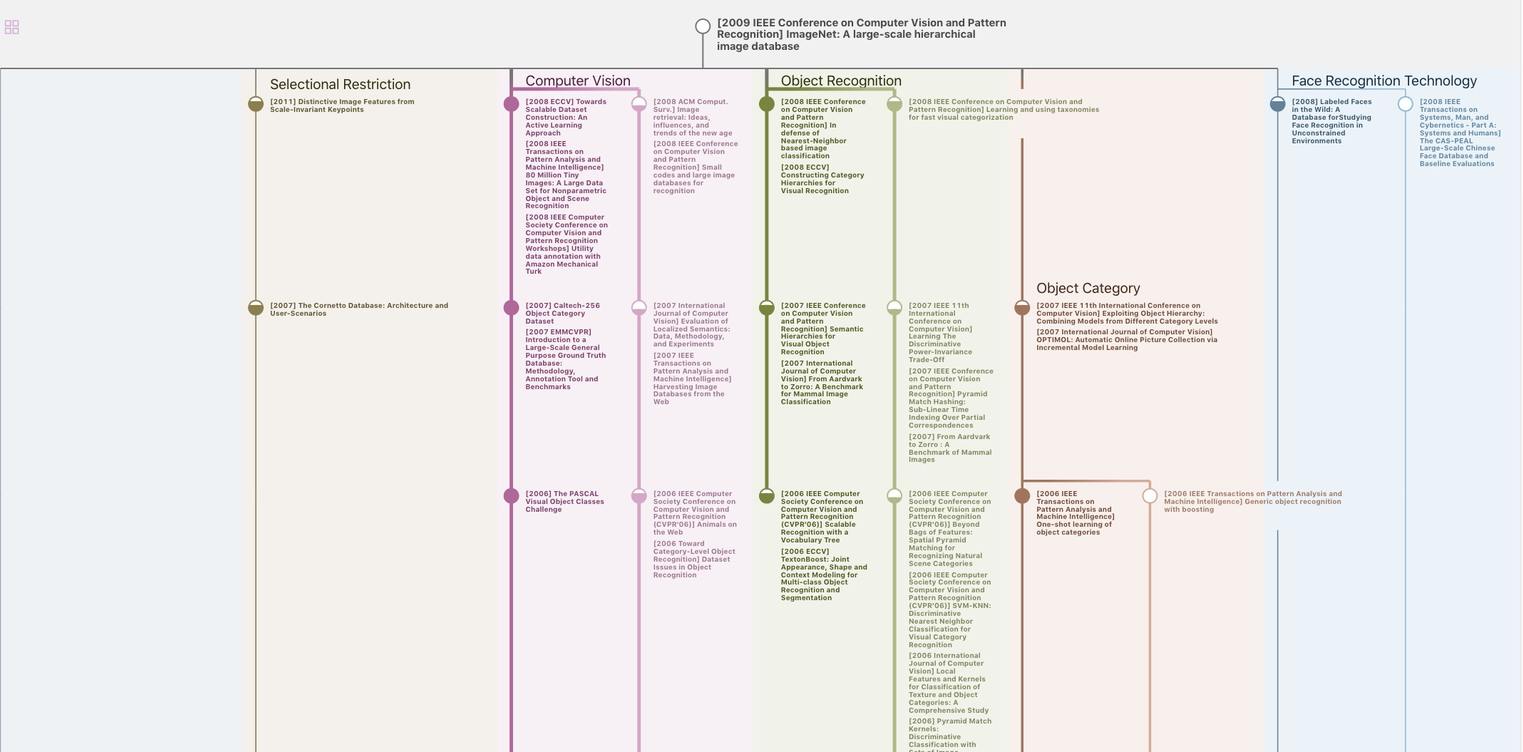
生成溯源树,研究论文发展脉络
Chat Paper
正在生成论文摘要