DeepSegmenter: Temporal Action Localization for Detecting Anomalies in Untrimmed Naturalistic Driving Videos
CoRR(2023)
Abstract
Identifying unusual driving behaviors exhibited by drivers during driving is essential for understanding driver behavior and the underlying causes of crashes. Previous studies have primarily approached this problem as a classification task, assuming that naturalistic driving videos come discretized. However, both activity segmentation and classification are required for this task due to the continuous nature of naturalistic driving videos. The current study therefore departs from conventional approaches and introduces a novel methodological framework, DeepSegmenter, that simultaneously performs activity segmentation and classification in a single framework. The proposed framework consists of four major modules namely Data Module, Activity Segmentation Module, Classification Module and Postprocessing Module. Our proposed method won 8th place in the 2023 AI City Challenge, Track 3, with an activity overlap score of 0.5426 on experimental validation data. The experimental results demonstrate the effectiveness, efficiency, and robustness of the proposed system.
MoreTranslated text
Key words
Activity Segmentation Module,Classification Module,classification task,current study therefore departs,Data Module,DeepSegmenter,driver behavior,identifying unusual driving behaviors,novel methodological framework,Postprocessing Module,temporal action localization,untrimmed naturalistic driving videos
AI Read Science
Must-Reading Tree
Example
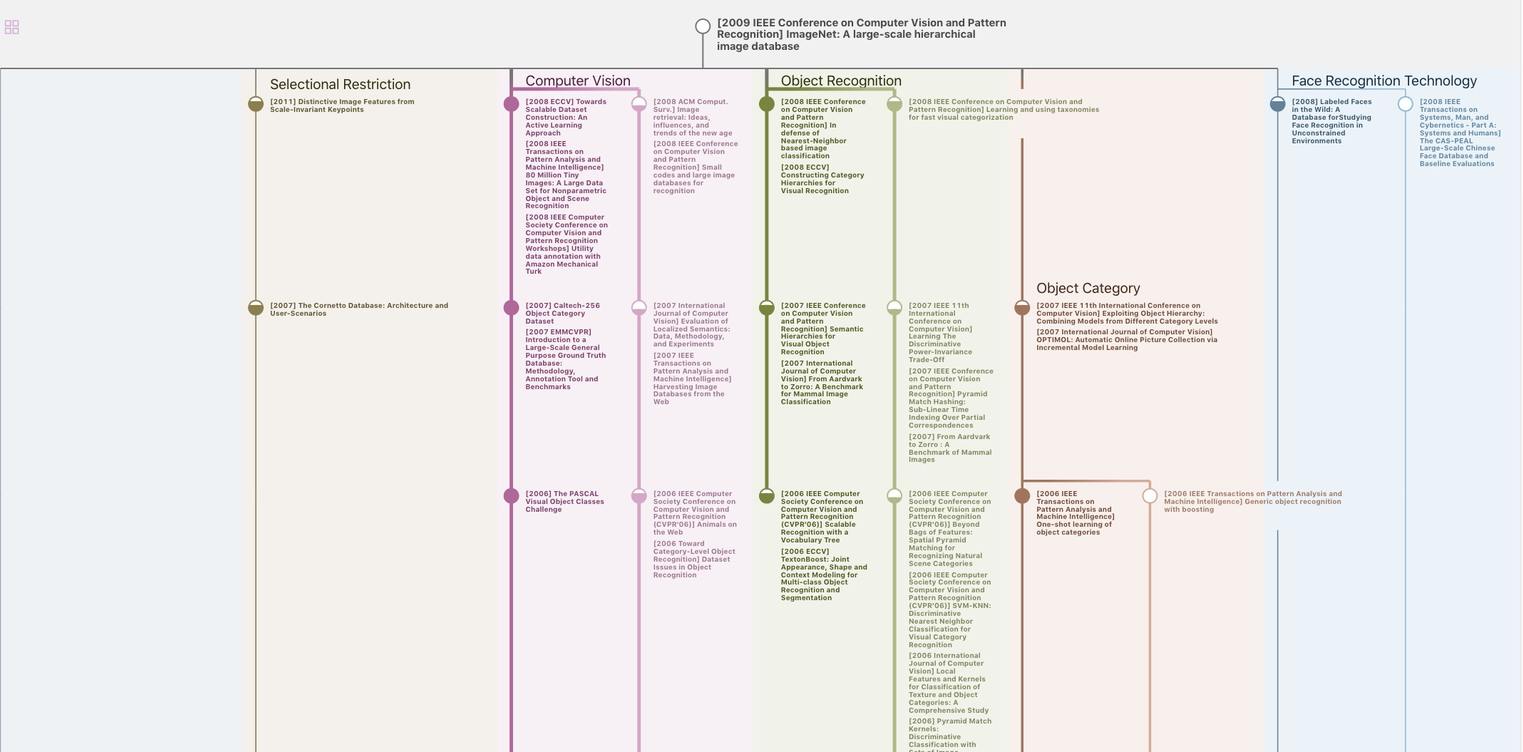
Generate MRT to find the research sequence of this paper
Chat Paper
Summary is being generated by the instructions you defined