Leveraging Multi-view Data for Improved Detection Performance: An Industrial Use Case
CoRR(2023)
摘要
Printed circuit boards (PCBs) are essential components of electronic devices, and ensuring their quality is crucial in their production. However, the vast variety of components and PCBs manufactured by different companies makes it challenging to adapt to production lines with speed demands. To address this challenge, we present a multi-view object detection framework that offers a fast and precise solution. We introduce a novel multi-view dataset with semi-automatic ground-truth data, which results in significant labeling resource savings. Labeling PCB boards for object detection is a challenging task due to the high density of components and the small size of the objects, which makes it difficult to identify and label them accurately. By training an object detector model with multi-view data, we achieve improved performance over single-view images. To further enhance the accuracy, we develop a multi-view inference method that aggregates results from different viewpoints. Our experiments demonstrate a 15% improvement in mAP for detecting components that range in size from 0.5 to 27.0 mm.
更多查看译文
关键词
improved detection performance,industrial use case,multi-view
AI 理解论文
溯源树
样例
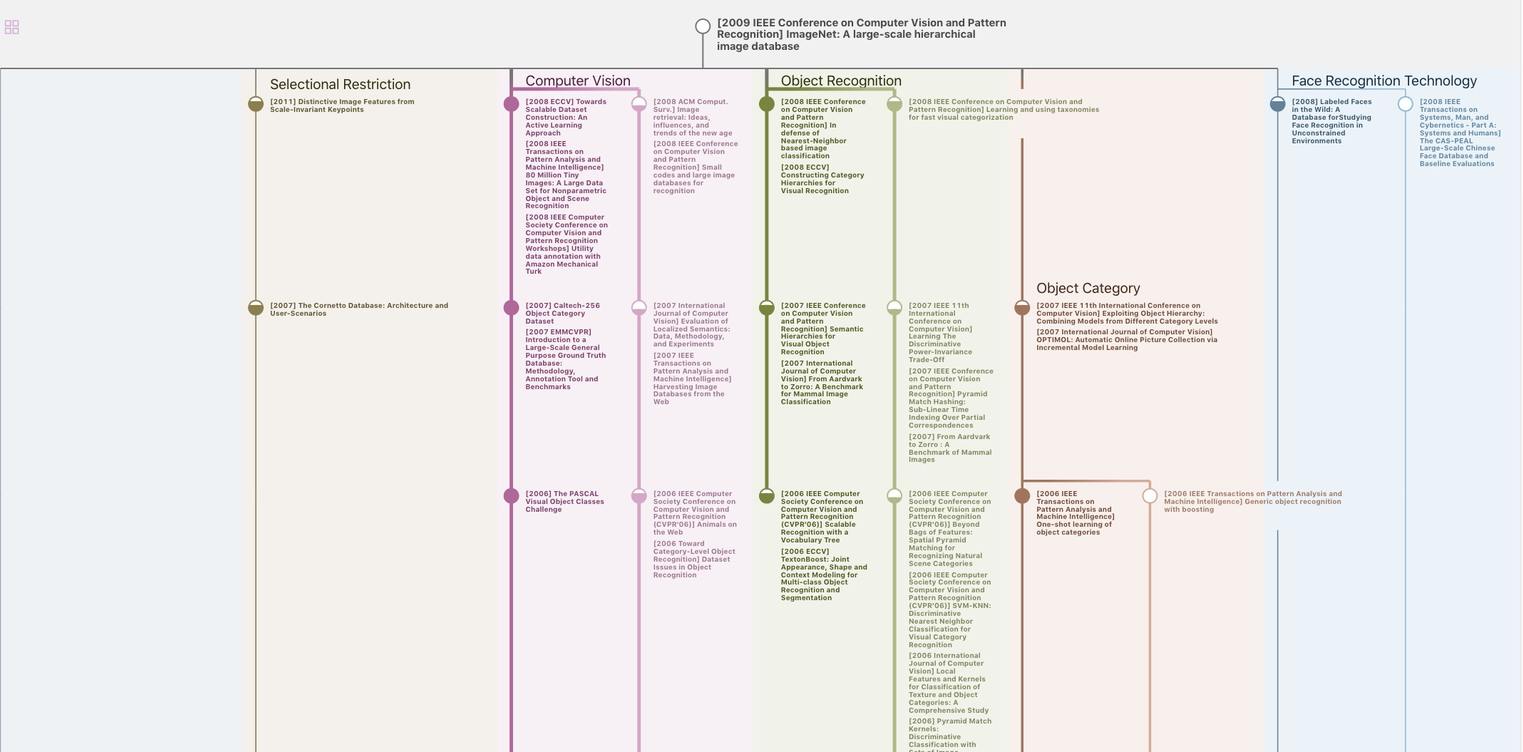
生成溯源树,研究论文发展脉络
Chat Paper
正在生成论文摘要