DeepSim-Nets: Deep Similarity Networks for Stereo Image Matching
CoRR(2023)
摘要
We present three multi-scale similarity learning architectures, or DeepSim networks. These models learn pixel-level matching with a contrastive loss and are agnostic to the geometry of the considered scene. We establish a middle ground between hybrid and end-to-end approaches by learning to densely allocate all corresponding pixels of an epipolar pair at once. Our features are learnt on large image tiles to be expressive and capture the scene's wider context. We also demonstrate that curated sample mining can enhance the overall robustness of the predicted similarities and improve the performance on radiometrically homogeneous areas. We run experiments on aerial and satellite datasets. Our DeepSim-Nets outperform the baseline hybrid approaches and generalize better to unseen scene geometries than end-to-end methods. Our flexible architecture can be readily adopted in standard multi-resolution image matching pipelines.
更多查看译文
关键词
deepsim-nets similarity networks
AI 理解论文
溯源树
样例
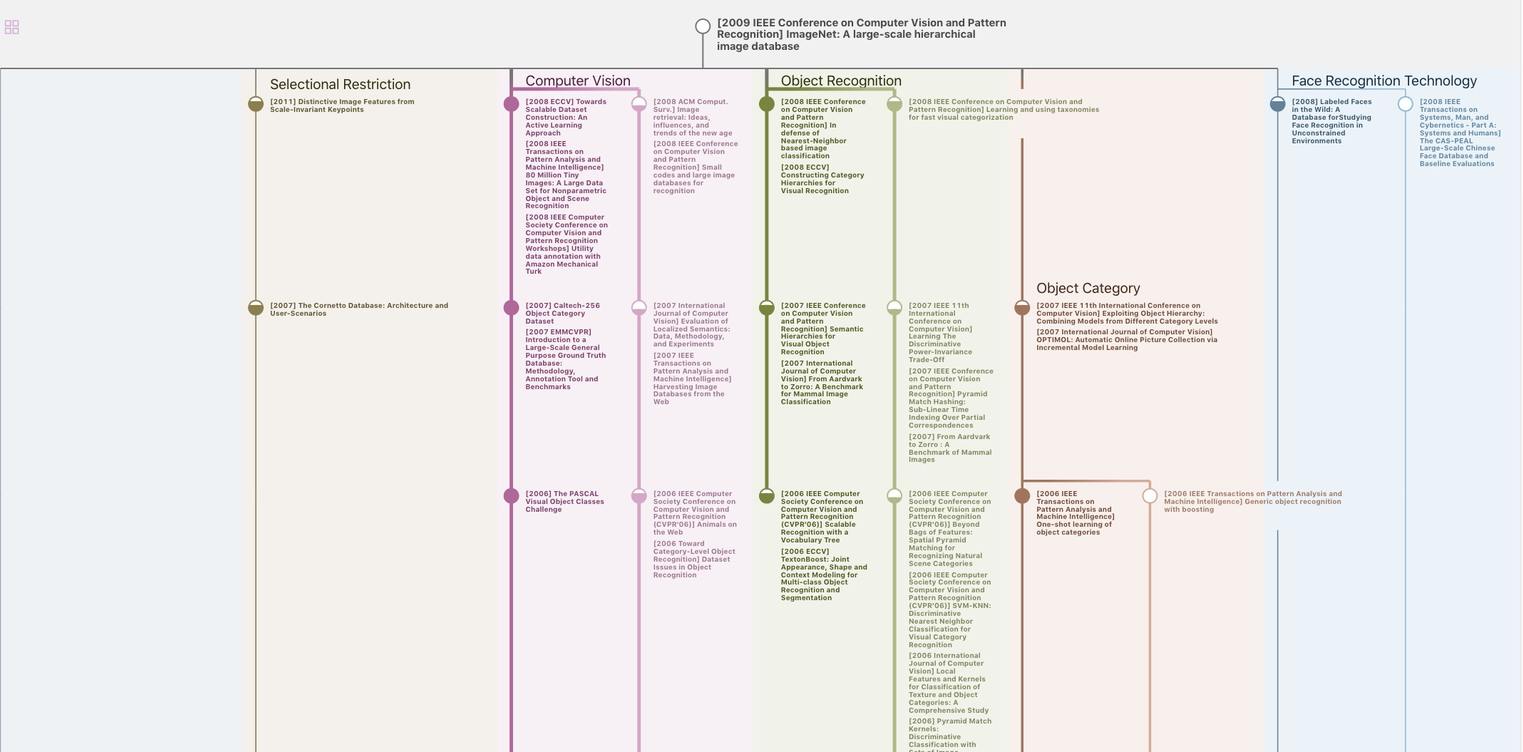
生成溯源树,研究论文发展脉络
Chat Paper
正在生成论文摘要