An investigation of non-informative priors for Bayesian dose-response modeling.
Regulatory toxicology and pharmacology : RTP(2023)
摘要
Toxicology analyses are built around dose-response modeling, and increasingly these methodologies utilize Bayesian estimation techniques. Bayesian estimation is unique because it includes prior distributional information in the analysis, which may impact the dose-response estimate meaningfully. As such analyses are often used for human health risk assessment, the practitioner must understand the impact of adding prior information into the dose-response study. One proposal in the literature is the use of the flat uniform prior distribution, which places a uniform prior probability over the dose-response model's parameters for a chosen range of values. Though the motivation of such a prior distribution is laudable in that it is most like maximum likelihood estimation seeking unbiased estimates of the dose-response, one can show that such priors add information and may introduce unexpected biases into the analysis. This manuscript shows through numerous empirical examples why prior distributions that are non-informative across all endpoints of interest do not exist for dose-response models; that is, other quantities of interest will be informed by choosing one inferential quantity not informed.
更多查看译文
关键词
Concentration response,Nonlinear models,Toxicology experiments,Uniform prior distributions
AI 理解论文
溯源树
样例
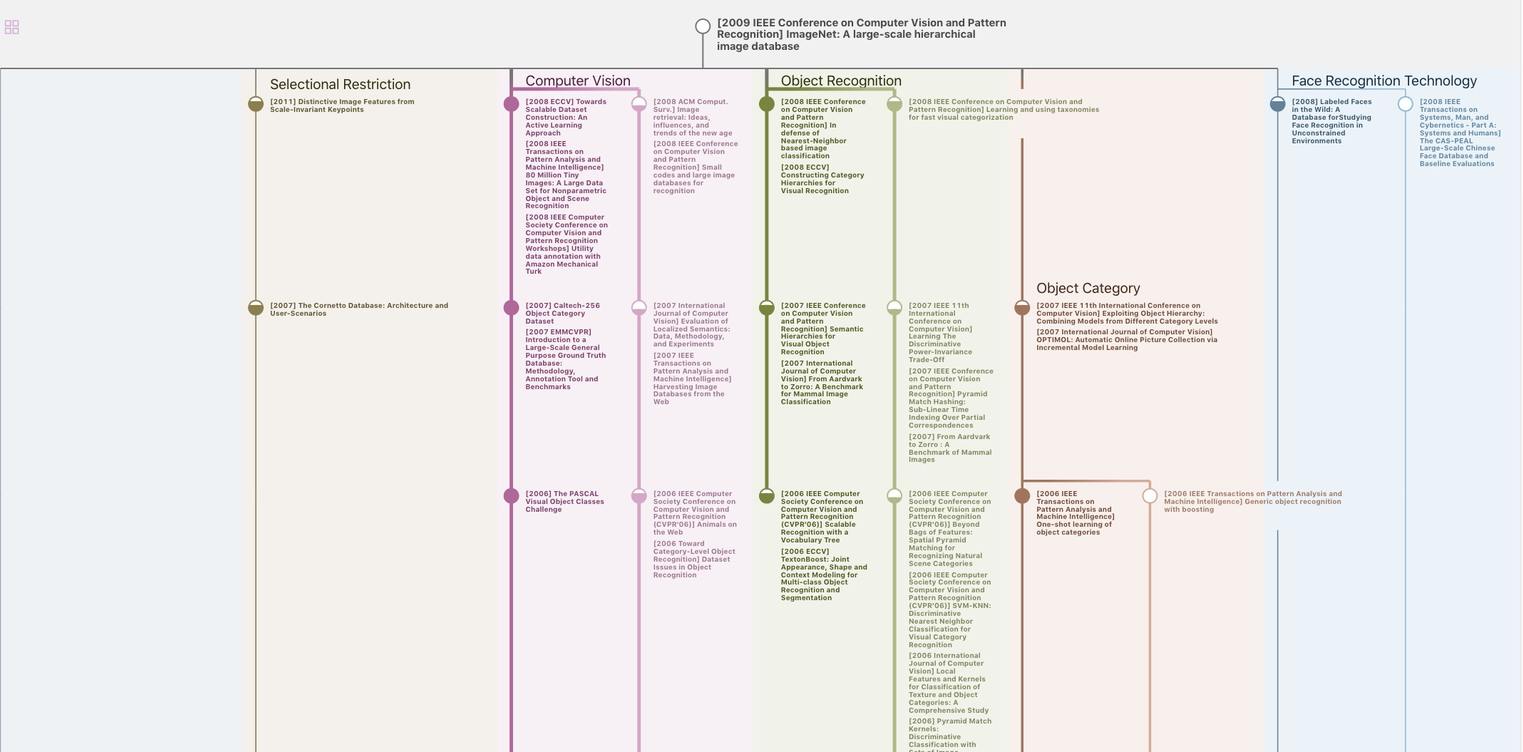
生成溯源树,研究论文发展脉络
Chat Paper
正在生成论文摘要