Accurate Prediction of in Vivo Protein Abundances by Coupling Constraint-Based Modelling and Machine Learning
Metabolic Engineering(2023)
Abstract
Quantification of how different environmental cues affect protein allocation can provide important insights for understanding cell physiology. While absolute quantification of proteins can be obtained by resource-intensive mass-spectrometry-based technologies, prediction of protein abundances offers another way to obtain insights into protein allocation. Yet, building machine learning models of high accuracy across diverse, sub-optimal conditions remains notoriously difficult due to the dynamic nature of protein allocation. Here we present CAMEL, a framework that couples constraint-based modelling with machine learning to predict protein abundance for any environmental condition. This is achieved by building machine learning models that leverage static features, derived from protein sequences, and condition-dependent features predicted from protein-constrained metabolic models. Our findings demonstrate that CAMEL results in excellent prediction of protein allocation in E. coli (average Pearson correlation of at least 0.9), and moderate performance in S. cerevisiae (average Pearson correlation of at least 0.5). Therefore, CAMEL outperformed contending approaches without using molecular read-outs from unseen conditions and provides a valuable tool for using protein allocation in biotechnological applications. Significance Statement Proteins perform diverse functions, and their allocation to different pathways affects the physiology of cellular systems. However, quantification of protein allocation is a challenging task, and methods for accurate prediction of protein abundance offer an alternative for characterizing the relationship between protein expression and cellular physiology. Yet, prediction of protein allocation remains notoriously difficult, particularly under suboptimal conditions, with existing attempts relying on other molecular read-outs from the respective environments. Here, we present an approach that couples machine learning with advances in constraint-based modelling to accurately predict in vivo protein abundances. The usage of environment-specific features, predicted from enzyme-constrained metabolic models, along with static protein features, renders the approach readily applicable in variety of biotechnologically relevant scenarios simulated by these models. ### Competing Interest Statement The authors have declared no competing interest.
MoreTranslated text
AI Read Science
Must-Reading Tree
Example
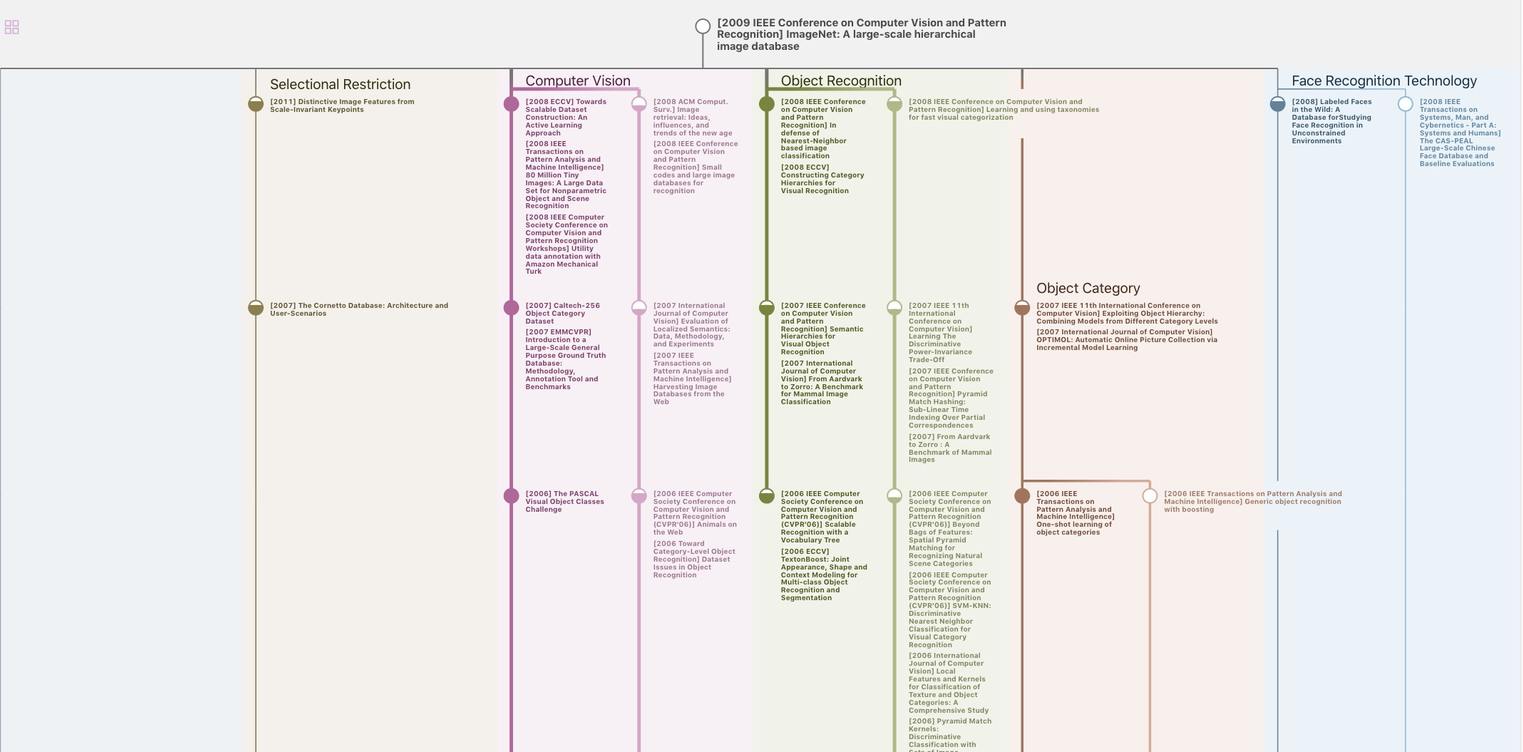
Generate MRT to find the research sequence of this paper
Chat Paper
Summary is being generated by the instructions you defined