Development and Validation of a Deep Learning Predictive Model Combining Clinical and Radiomic Features for Short-Term Postoperative Facial Nerve Function in Acoustic Neuroma Patients
Current medical science(2023)
摘要
Objective This study aims to construct and validate a predictable deep learning model associated with clinical data and multi-sequence magnetic resonance imaging (MRI) for short-term postoperative facial nerve function in patients with acoustic neuroma. Methods A total of 110 patients with acoustic neuroma who underwent surgery through the retrosigmoid sinus approach were included. Clinical data and raw features from four MRI sequences (T1-weighted, T2-weighted, T1-weighted contrast enhancement, and T2-weighted-Flair images) were analyzed. Spearman correlation analysis along with least absolute shrinkage and selection operator regression were used to screen combined clinical and radiomic features. Nomogram, machine learning, and convolutional neural network (CNN) models were constructed to predict the prognosis of facial nerve function on the seventh day after surgery. Receiver operating characteristic (ROC) curve and decision curve analysis (DCA) were used to evaluate model performance. A total of 1050 radiomic parameters were extracted, from which 13 radiomic and 3 clinical features were selected. Results The CNN model performed best among all prediction models in the test set with an area under the curve (AUC) of 0.89 (95% CI, 0.84–0.91). Conclusion CNN modeling that combines clinical and multi-sequence MRI radiomic features provides excellent performance for predicting short-term facial nerve function after surgery in patients with acoustic neuroma. As such, CNN modeling may serve as a potential decision-making tool for neurosurgery.
更多查看译文
关键词
acoustic neuroma,convolutional neural network,facial nerve function,machine learning,multi-sequence magnetic resonance imaging
AI 理解论文
溯源树
样例
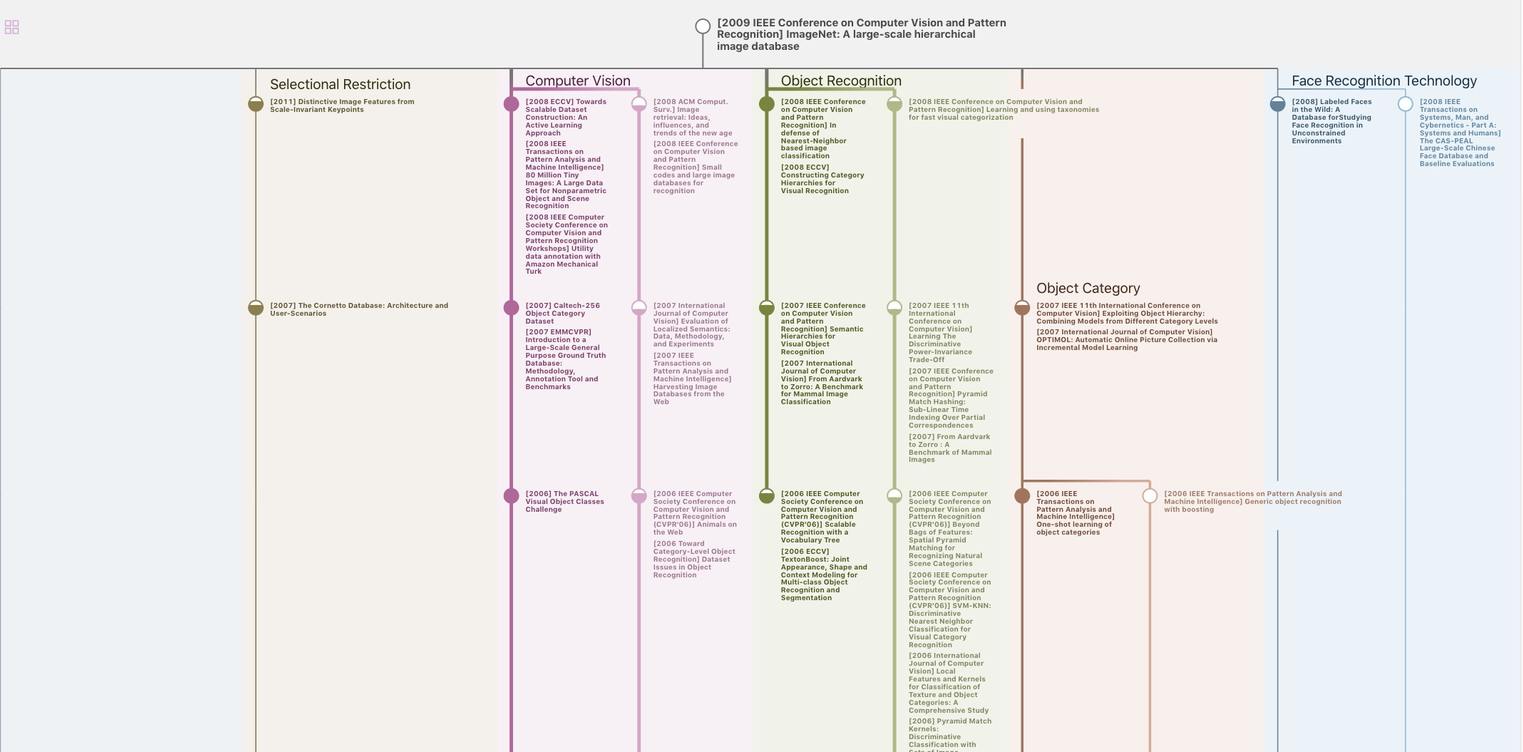
生成溯源树,研究论文发展脉络
Chat Paper
正在生成论文摘要