Data-driven microscopy allows for automated context-specific acquisition of high-fidelity image data
CELL REPORTS METHODS(2023)
摘要
Light microscopy is a powerful single-cell technique that allows for quantitative spatial information at subcellular resolution. However, unlike flow cytometry and single-cell sequencing techniques, microscopy has issues achieving high-quality population-wide sample characterization while maintaining high resolution. Here, we present a general framework, data-driven microscopy (DDM) that uses real-time populationwide object characterization to enable data-driven high-fidelity imaging of relevant phenotypes based on the population context. DDM combines data-independent and data-dependent steps to synergistically enhance data acquired using different imaging modalities. As a proof of concept, we develop and apply DDM with plugins for improved high-content screening and live adaptive microscopy for cell migration and infection studies that capture events of interest, rare or common, with high precision and resolution. We propose that DDM can reduce human bias, increase reproducibility, and place single-cell characteristics in the context of the sample population when interpreting microscopy data, leading to an increase in overall data fidelity.
更多查看译文
关键词
adaptive feedback microscopy,automation,cell migration,data analysis,data-driven microscopy,event-driven microscopy,high-content screening,host-pathogen interactions,image analysis
AI 理解论文
溯源树
样例
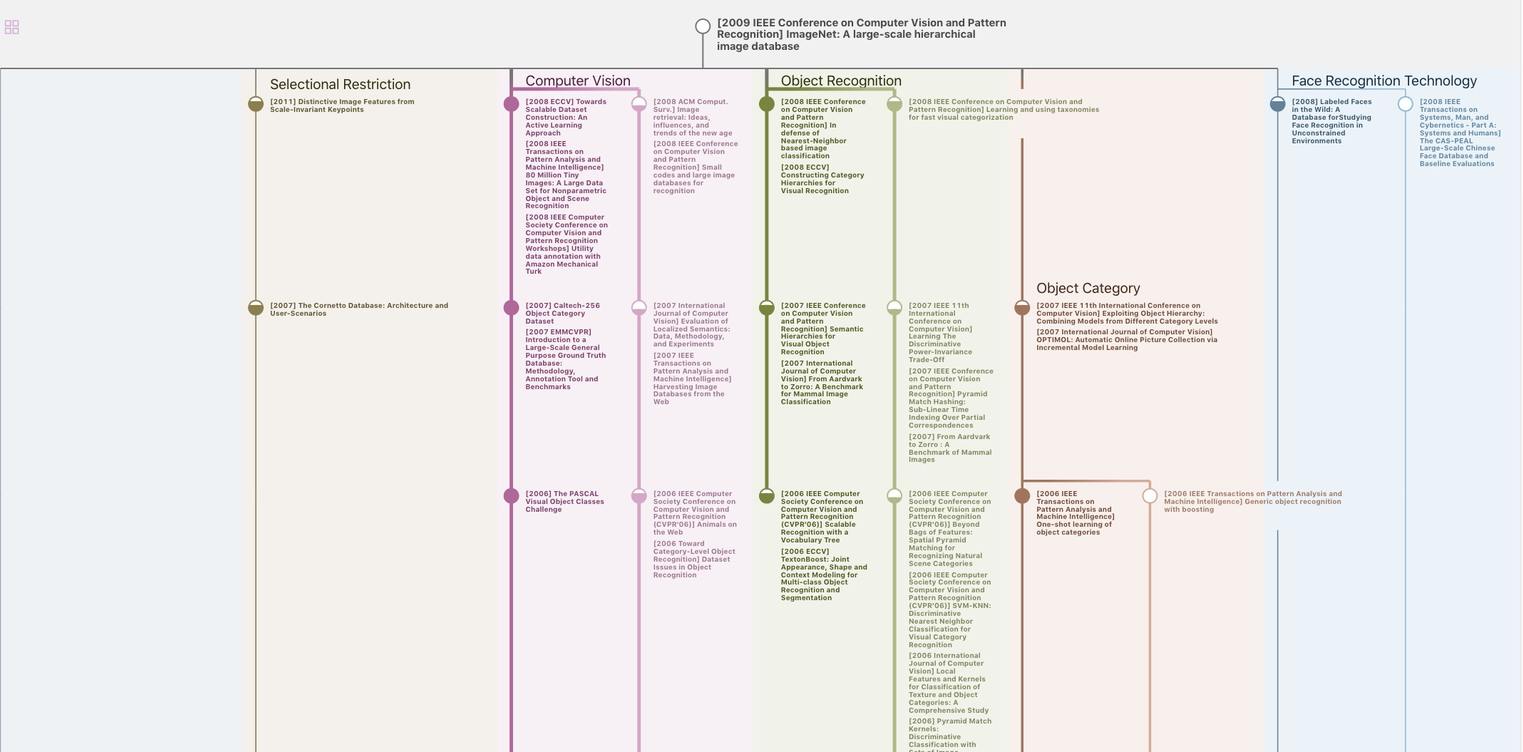
生成溯源树,研究论文发展脉络
Chat Paper
正在生成论文摘要