Online visual monitoring method for liquid rocket engine nozzle welding based on a multi-task deep learning model
Journal of Manufacturing Systems(2023)
Abstract
Online quality monitoring is important in liquid rocket engine nozzle (LREN) welding procedures. Convolutional neural networks (CNNs) are widely applied in welding quality monitoring. However, most CNNs perform single visual tasks and provide limited information. They are not competent in LREN welding quality monitoring. This paper presents an online visual sensing method for LREN welding quality monitoring based on a multi-task deep learning model. Weld-detection-segmentation network (WDS-net), a multi-task CNN for simultaneous detection and segmentation is proposed for weld defect detection and weld width measurement. WDS-net obtains more information from welding images and depicts the welding process more adequately compared with single-task models. A machine vision system is constructed to monitor the LREN welding process. Then, experiments are conducted to obtain LREN welding images and construct a dataset. WDS-net is trained and tested on the dataset and obtains a 95.85% mean average precision (mAP) for weld defect detection and a 92.63% intersection over union (IoU) for weld zone segmentation. Finally, WDS-net is tested on LREN welding image sequences, and the results are compared with the offline measurement results of corresponding welds. WDS-net detects, locates the defects without omission, and measures weld width with a mean error within 0.1 mm compared with the actual weld. WDS-net’s monitoring performance and its average inference rate of 147 frames per second meet the requirements of online monitoring of LREN welding.
MoreTranslated text
Key words
Deep learning,Liquid rocket engine (LRE) nozzle welding,Machine vision,Multi-task model,Welding quality monitoring
AI Read Science
Must-Reading Tree
Example
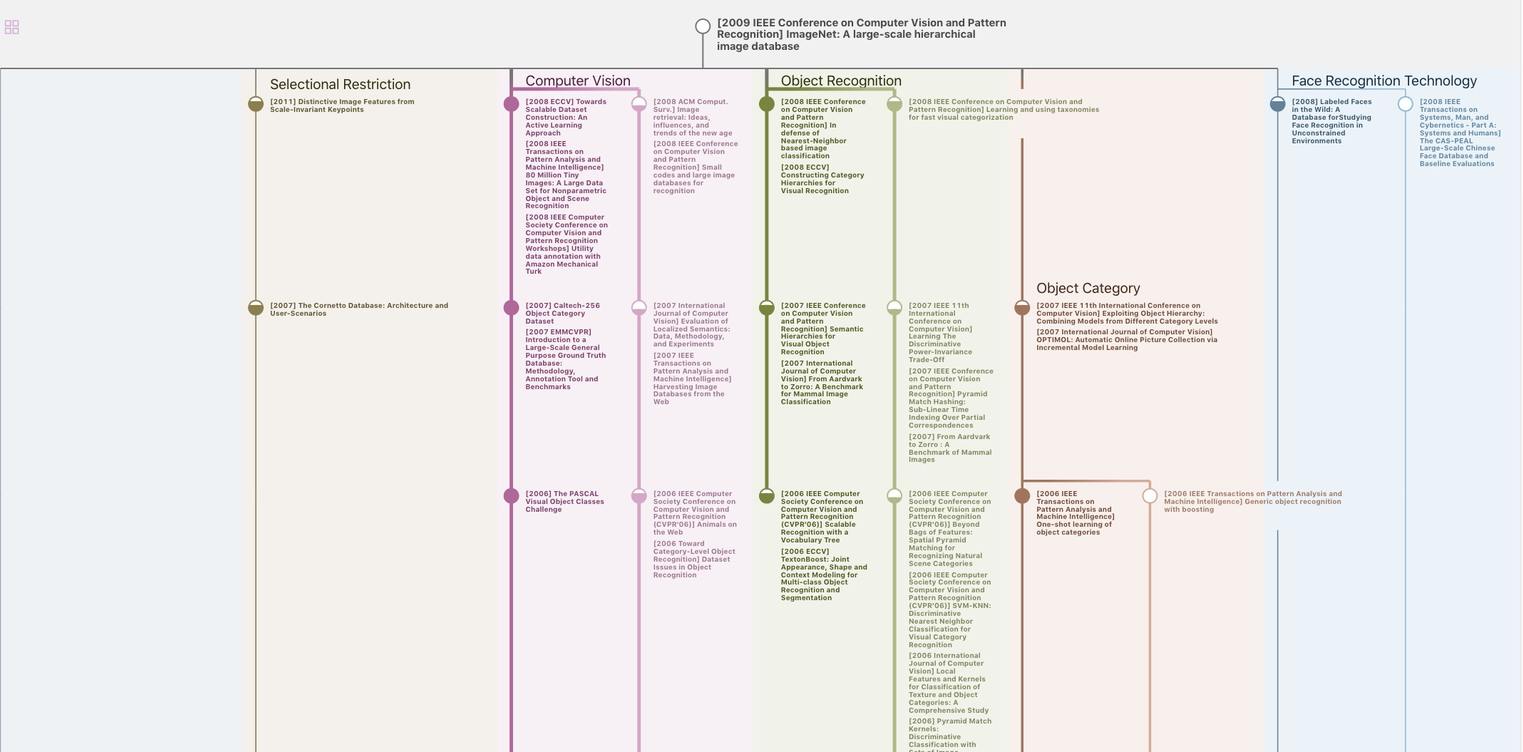
Generate MRT to find the research sequence of this paper
Chat Paper
Summary is being generated by the instructions you defined