Variational Bayesian State Space Model for dynamic process fault detection
Journal of Process Control(2023)
摘要
Industrial processes are subject to various noise disturbances that lead to the stochastic nature of the modeled system and the uncertainty of the model parameters. In this paper, a variational Bayesian State Space Model (VBSSM) model is developed for dynamic process monitoring, allowing the uncertainty of parameters to be described by probabilities. The conjugate prior allows the posterior distribution of the parameters to be estimated iteratively through the Rauch Tung Striebel (RTS) smoothing estimation with the VB framework. The next state can be better predicted even in the presence of noisy perturbations, and imperceptible correlations among multiple variables can be found. By maximizing the lower bound of the objective function to approximate the true posterior distribution of the parameters, the fault detection index is constructed by the corresponding residual. Finally, the effectiveness and superiority of the proposed method is verified by numerical simulations, Tennessee Eastman process and hot rolling industry examples.
更多查看译文
关键词
Dynamic process,Fault detection,State space model,Rauch Tung Striebel smoothing,Variational Bayesian inference
AI 理解论文
溯源树
样例
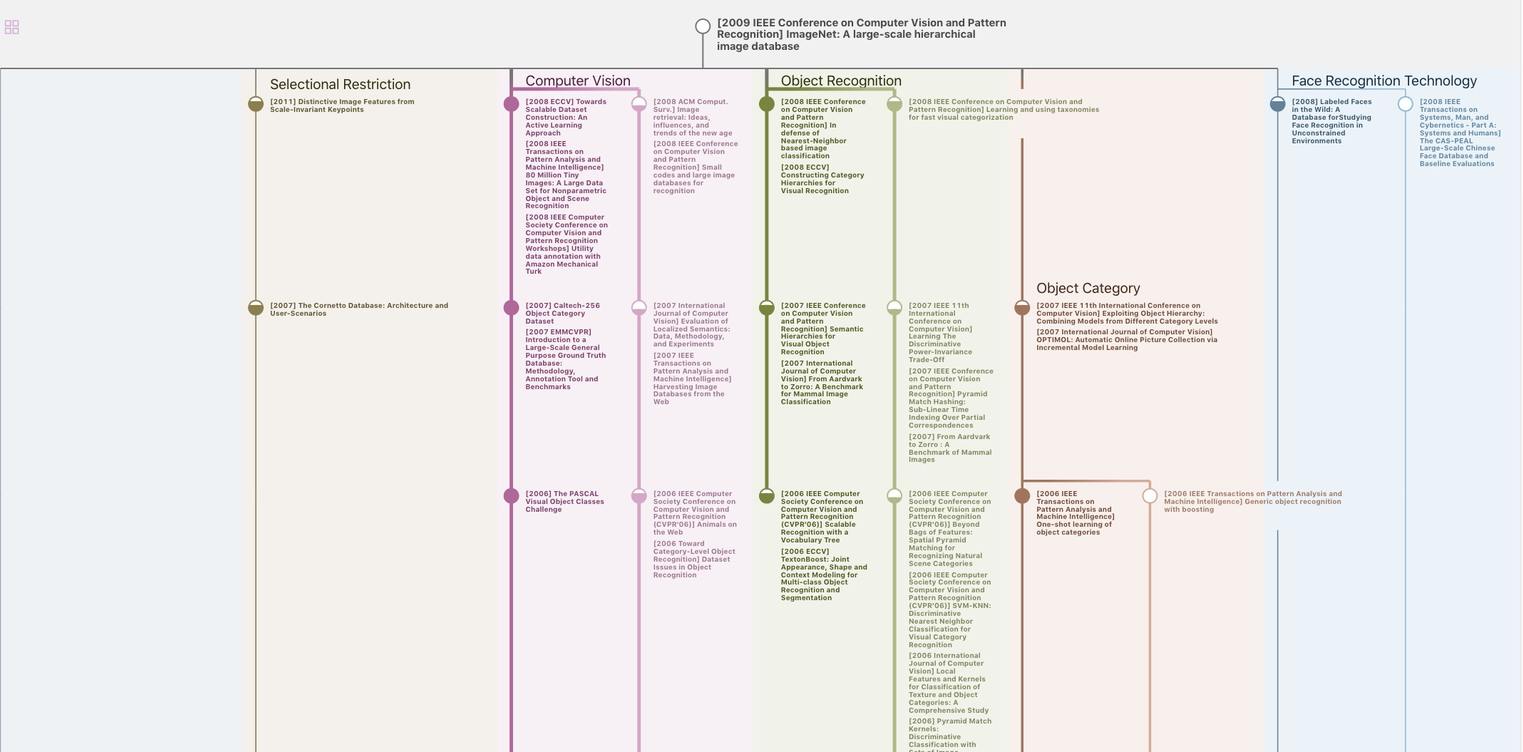
生成溯源树,研究论文发展脉络
Chat Paper
正在生成论文摘要