Seventy years later: the legacy of the Hodgkin and Huxley model in computational neuroscience
The Journal of physiology(2023)
摘要
The theory of the action potential was formulated by Hodgkin and Huxley 70 years ago. Five landmark papers summarizing their experimental data and quantitative model were published in The Journal of Physiology in 1952 providing the first description of action potential generation. This is one of the first examples of a close collaboration between mathematics and neuroscience that played a key role in laying the foundation of modern computational neuroscience. In recent decades computational neuroscience has become recognised as a prominent and independent subdiscipline of neuroscience. Existing computational approaches are used to advance our knowledge across all scales relevant to the neuroscience endeavour: from nanometre-sized sub-cellular structures to brain-wide neuroimaging studies and quantification of organismal behaviour. Moreover, experimental data, pouring onto the storage devices of our computers at an exponentially increasing rate, inspire the development of new cutting-edge computational approaches. Collaborative efforts between computational, theoretical and experimental neuroscientists play an increasingly important role in the deeper understanding of brain function and dysfunction. In this special issue of The Journal of Physiology on Computational Neuroscience, we aim to give a broad overview of the diverse avenues of research utilising computational approaches to better understand the basic principles of neuronal communication, how the brain processes, stores and retrieves information. The invited reviews in this issue address numerous aspects of computational approaches in neuroscience research. How did the Hodgkin–Huxley model influence neuroscience since its publication in 1952? Its impact on research into single cell computation mechanisms continues to grow to this day. These efforts are not restricted to neuroscience. Since 1960 the model has been extended to cardiac muscle cells, and the impact of the model on modern physiology in general is discussed in this issue by Noble (2022). The relationship between current neuronal models and the Hodgkin–Huxley model is detailed in two reviews. The first, by Petousakis et al. (2023), discusses recent studies in which this biophysical modelling was relied upon to unveil principles of dendritic information processing spanning from non-linear dendritic integration to the contribution of backpropagating action potentials and synaptic plasticity to single neuron computation. The second, by Haufler et al. (2023), reviews the legacy of the Hodgkin–Huxley model in the field of neocortical network modelling. The authors discuss how the extension of the model's framework through single-neuron and small-network modelling enabled the evolution of cortical network modelling. How do various plastic changes combine to improve behavioural outcomes? Richards & Kording (2023) explore the utility of gradient descent to better understand how small changes occurring in the forms of synaptic plasticity lead to improved performance during learning. In their review they examine various forms of cellular and circuit plasticity mechanisms and their relationships to gradients. What type of models capture network activity? A review by Bernáez Timón et al. (2023) provides an overview of the diversity of neural network models, including models relying on recent advances in machine learning, and how they contribute to the understanding of brain function at the level of circuit computations. The authors emphasise the importance of equipping network models with biological connectivity and training network models under realistic biological constraints. They argue that incorporating these biologically relevant parameters helps in understanding the mechanisms by which biological neural network functions emerge. The review by Hadjiabadi & Soltesz (2023) explores how computational modelling can help to unveil the functional role of individual cells in network activity. They explore this through examining the behaviour of the sparse but highly connected hub neurons. This review gives an insight into how the combination of computational modelling and machine learning methods helps in understanding higher-order neural communication at the cellular level in large-scale networks. The formation of neuronal assemblies in recurrent networks and the contribution of synaptic plasticity, symmetry breaking, competition and stability to this process are reviewed by Miehl et al. (2023). They highlight different novel concepts that identify assemblies as computational units and explore how they can be useful in understanding the brain's computational prowess. The review by Hennig (2023) investigates the relationship between network structure and function. Does structure determine the function of the network? The review explores the question whether degeneracy, sloppiness and representational drift challenge the idea of a tight structure–function relationship, or alternatively they offer insights into the organising principles of neuronal network dynamics. Finally, the review of Levenstein & Okun (2023) summarises present knowledge of the basic statistical properties of neuronal spiking activity in vivo and the analytical probability distributions that best capture them. The last review in this special issue explores the relevance of computational models in a clinical setting. Spinal cord stimulation for chronic pain management relies on computational models for optimisation of stimulating electrode design and stimulation parameters. Liang et al. (2023) provide a systematic literature review and a qualitative meta-synthesis of the evolution of computational models used in this setting to evaluate whether increased complexity in modelling leads to improved accuracy and better clinical efficacy. This special issue also contains several research articles that address a wide variety of questions related to ion channel and receptor function, network dynamics in large scale networks and clinical applications. This special issue of The Journal of Physiology will be a valuable resource to everyone who is currently using computational models in their studies or interested in doing so in the future. It is also an interesting issue for researchers who are applying theoretical concepts to their work. The topics in this issue are diverse and stretch from cellular to network level analysis. The authors of this special issue are leaders in their fields, and they provide in-depth insights into their areas of research. The topics discussed in this special issue hopefully will stimulate novel computational approaches that will continue to enrich neuroscience research in the years and decades to come. Please note: The publisher is not responsible for the content or functionality of any supporting information supplied by the authors. Any queries (other than missing content) should be directed to the corresponding author for the article. No competing interests declared. Both authors have read and approved the final version of this manuscript and agree to be accountable for all aspects of the work in ensuring that questions related to the accuracy or integrity of any part of the work are appropriately investigated and resolved. All persons designated as authors qualify for authorship, and all those who qualify for authorship are listed. None.
更多查看译文
关键词
huxley model,computational neuroscience,hodgkin
AI 理解论文
溯源树
样例
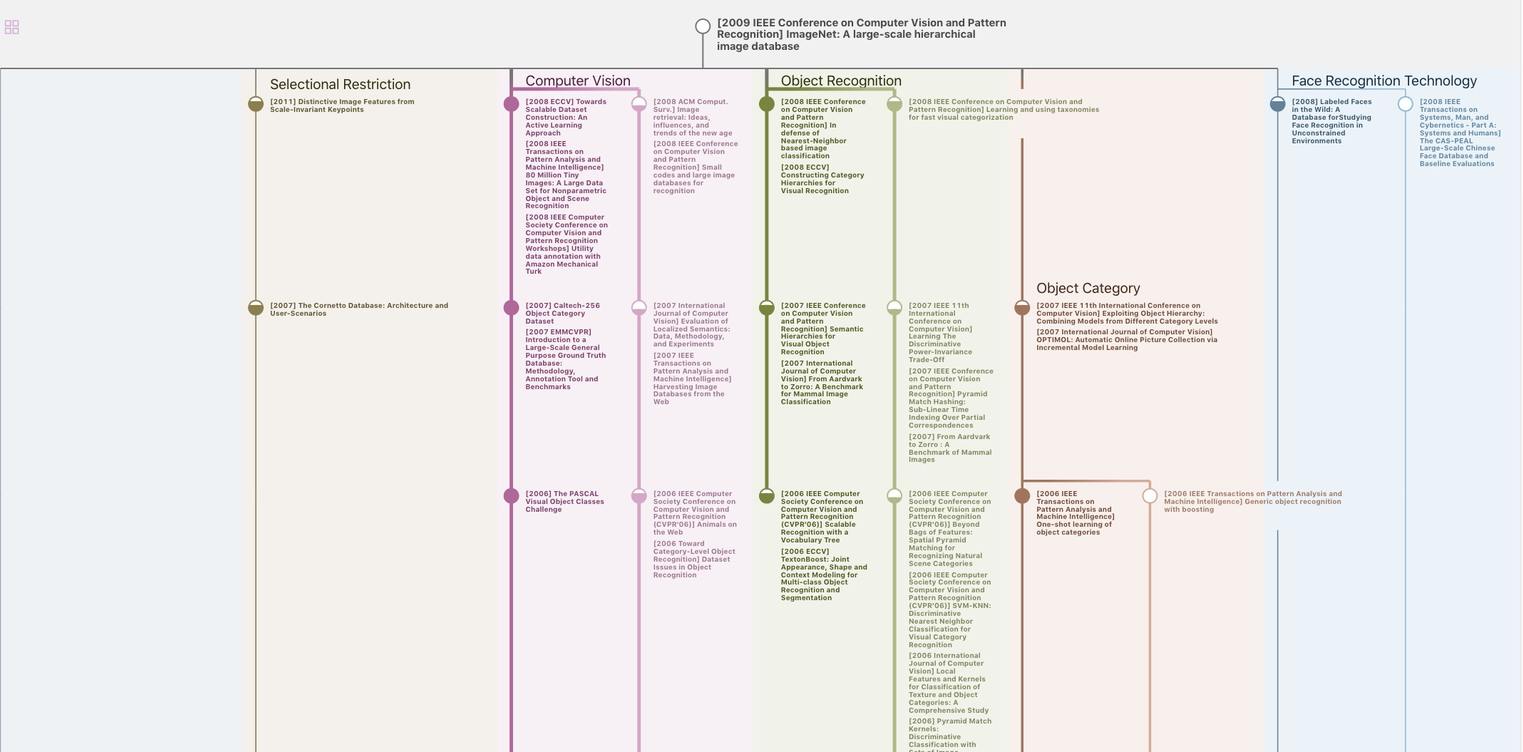
生成溯源树,研究论文发展脉络
Chat Paper
正在生成论文摘要