Early detection of in-patient deterioration: one prediction model does not fit all
medRxiv (Cold Spring Harbor Laboratory)(2020)
摘要
Objectives Early detection of subacute potentially catastrophic illnesses using available data is a clinical imperative, and scores that report risk of imminent events in real time abound. Patients deteriorate for a variety of reasons, and it is unlikely that a single predictor such as an abnormal National Early Warning Score (NEWS) will detect all of them equally well. The objective of this study was to test the idea that the diversity of reasons for clinical deterioration leading to ICU transfer mandates multiple targeted predictive models.
Design Individual chart review to determine the clinical reason for ICU transfer; determination of relative risks of individual vital signs, lab tests and cardiorespiratory monitoring measures for prediction of each clinical reason for ICU transfer; logistic regression modeling for the outcome of ICU transfer for a specific clinical reason.
Setting Cardiac medical-surgical ward; tertiary care academic hospital.
Patients 8111 adult patients, 457 of whom were transferred to an ICU for clinical deterioration.
Interventions None.
Measurements and main results We calculated the contributing relative risks of individual vital signs, lab tests and cardiorespiratory monitoring measures for prediction of each clinical reason for ICU transfer, and used logistic regression modeling to calculate ROC areas and relative risks for the outcome of ICU transfer for a specific clinical reason. The reasons for clinical deterioration leading to ICU transfer were varied, as were their predictors. For example, the three most common reasons – respiratory instability, infection and suspected sepsis, and heart failure requiring escalated therapy – had distinct signatures of illness. Statistical models trained to target specific reasons for ICU transfer performed better than one model targeting combined events, and both performed better than the untrained NEWS score.
Conclusions and relevance A single predictive model for clinical deterioration does not perform as well as having multiple models trained for the individual specific clinical events leading to ICU transfer.
### Competing Interest Statement
J Randall Moorman, M.D. is Chief Medical Officer and shareholder and Matthew T. Clark, PhD is Chief Scientific Officer and shareholder in Advanced Medical Predictive Devices, Diagnostics, and Displays, Charlottesville, VA.
### Funding Statement
Grant support was provided by the Frederick Thomas Advanced Medical Analytics Fund at the University of Virginia.
### Author Declarations
All relevant ethical guidelines have been followed; any necessary IRB and/or ethics committee approvals have been obtained and details of the IRB/oversight body are included in the manuscript.
Yes
All necessary patient/participant consent has been obtained and the appropriate institutional forms have been archived.
Yes
I understand that all clinical trials and any other prospective interventional studies must be registered with an ICMJE-approved registry, such as ClinicalTrials.gov. I confirm that any such study reported in the manuscript has been registered and the trial registration ID is provided (note: if posting a prospective study registered retrospectively, please provide a statement in the trial ID field explaining why the study was not registered in advance).
Yes
I have followed all appropriate research reporting guidelines and uploaded the relevant EQUATOR Network research reporting checklist(s) and other pertinent material as supplementary files, if applicable.
Yes
Clinical data obtained with IRB approval for the 8111 patients in this study is stored under encryption on servers at the University of Virginia. Inquiries regarding access to data from the University of Virginia's Center for Advanced Medical Analytics should be done by contacting J Randall Moorman, M.D. via email at rm3h{at}virginia.edu.
更多查看译文
关键词
early detection,prediction model,in-patient
AI 理解论文
溯源树
样例
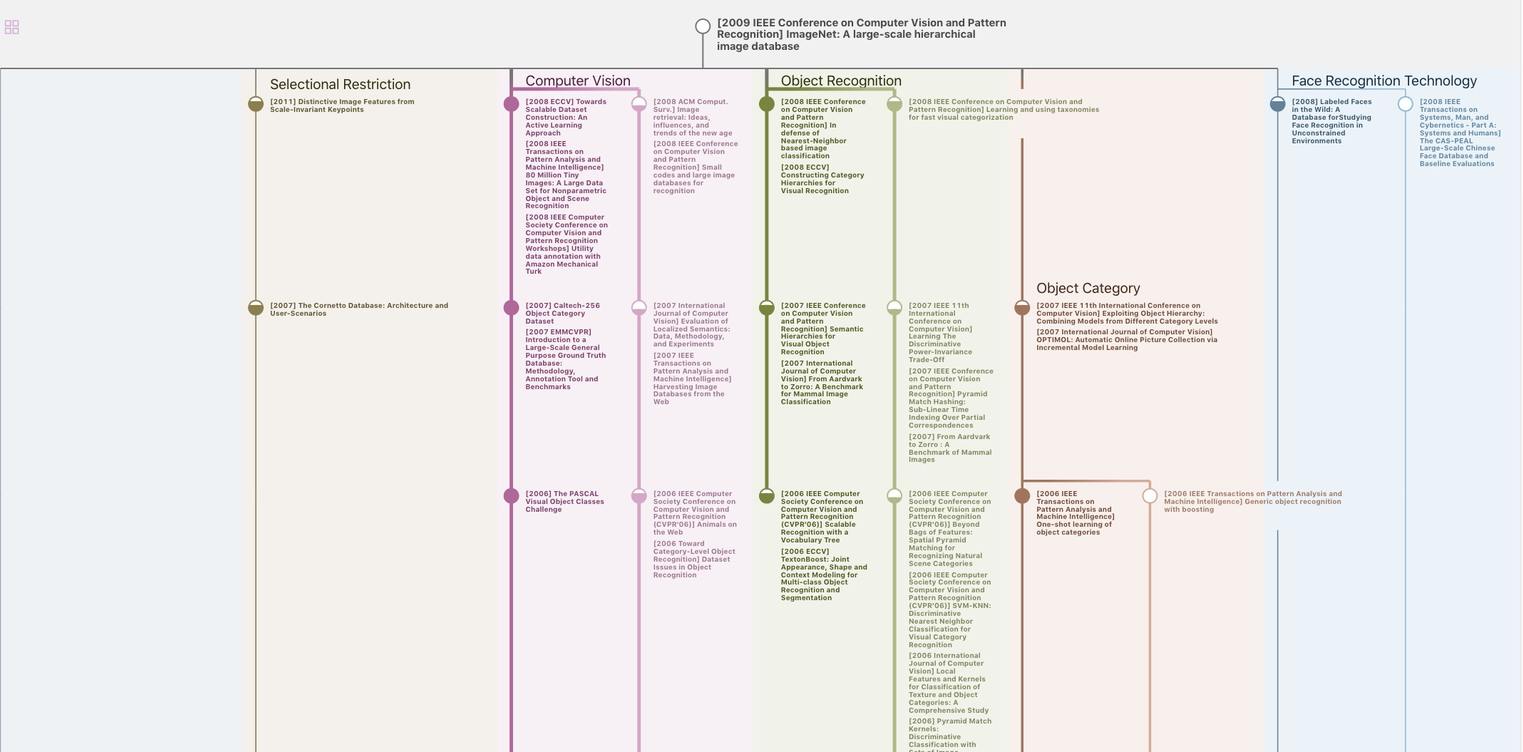
生成溯源树,研究论文发展脉络
Chat Paper
正在生成论文摘要