Identifying robust biomarkers of infection through an omics-based meta-analysis
medRxiv (Cold Spring Harbor Laboratory)(2020)
摘要
A fundamental problem for disease treatment is that while antibiotics are a powerful counter to bacteria, they are ineffective against viruses. To ensure a given individual receives optimal treatment given their disease state and to reduce over-prescription of antibiotics leading to antimicrobial resistance, the host response can be measured to distinguish between the two states. To establish a predictive biomarker panel of disease state we conducted a meta-analysis of human blood infection studies using Machine Learning (ML). We focused on publicly available gene expression data from two widely used platforms, Affymetrix and Illumina microarrays, and integrated over 2000 samples for each platform to develop optimal gene panels. On average our models predicted 80% of bacterial and 85% viral samples correctly by class of infection type. For our best performing model, identified with an evolutionary algorithm, 93% of bacterial and 89% of viral samples were classified correctly. To enable comparison between the two differing microarray platforms, we reverse engineered the underlying molecular regulatory network and overlay the identified models. This revealed that although the exact gene-level overlap between models generated from the two technologies was relatively low, both models contained genes in the same areas of the network, indicating that the same functional changes in host biology were being detected, providing further confidence in the robustness of our models. Specifically, this convergence was to pathways including the Type I interferon Signalling Pathway, Chemotaxis, Apoptotic Processes, and Inflammatory / Innate Response. Amongst and related to these pathways we found three genes, IFI27, LY6E , and CD177 , particularly prevalent throughout our analysis.
Author summary Bacterial and viral disease require specific treatments, and whilst there are various treatment options for specific infection types, rapid diagnosis and identification of the optimal treatment remains challenging. Even in wealthier countries with developed healthcare systems, unnecessary prescription of antibiotics to patients with viral infections is causing phenomena such as multi-drug resistent bacteria. One way to distinguish a viral from bacterial infection is to measure an individual’s responses, for example by measuring the expression of particular genes in a blood sample, as different types of infections trigger different types of responses. In our study we analysed thousands of previously collected data sets from human blood, where individuals had either viral, bacterial or no infection (control). We used machine learning to identify “signatures” – small sets of genes that are indicative of the type of infection (if any) carried by an individual. Within data sets we used two different technology platforms had been used to collect data. We demonstrated that their gene-level signatures do not overlap perfectly when derived from the different platforms, the biological networks from which those genes were derived, however, had a high overlap – giving confidence that our models are robust against technology artefacts or bias. We have identified a small set of genes that serve as strong biomarkers of infection status in humans.
### Competing Interest Statement
The authors have declared no competing interest.
### Funding Statement
This work was also supported by the Chem-Bio Diagnostics program contract HDTRA1-12-D-0003-0023 from the Department of Defense Chemical and Biological Defense program through the Defense Threat Reduction Agency (DTRA).
### Author Declarations
I confirm all relevant ethical guidelines have been followed, and any necessary IRB and/or ethics committee approvals have been obtained.
Yes
The details of the IRB/oversight body that provided approval or exemption for the research described are given below:
The publication used already available data. Therefore no additional approval was required.
All necessary patient/participant consent has been obtained and the appropriate institutional forms have been archived.
Yes
I understand that all clinical trials and any other prospective interventional studies must be registered with an ICMJE-approved registry, such as ClinicalTrials.gov. I confirm that any such study reported in the manuscript has been registered and the trial registration ID is provided (note: if posting a prospective study registered retrospectively, please provide a statement in the trial ID field explaining why the study was not registered in advance).
Yes
I have followed all appropriate research reporting guidelines and uploaded the relevant EQUATOR Network research reporting checklist(s) and other pertinent material as supplementary files, if applicable.
Yes
All data is available at the following Gene expression Omnibus IDs: GSE49954, GSE50628, GSE54992, GSE25504, GSE66099, GSE69606, GSE6269, GSE18090, GSE28750, GSE34205, GSE52428, GSE95104, GSE17156, GSE30550, GSE29385, GSE32707, GSE37250, GSE40396, GSE60244, GSE64456, GSE68310.
更多查看译文
关键词
robust biomarkers,infection,omics-based,meta-analysis
AI 理解论文
溯源树
样例
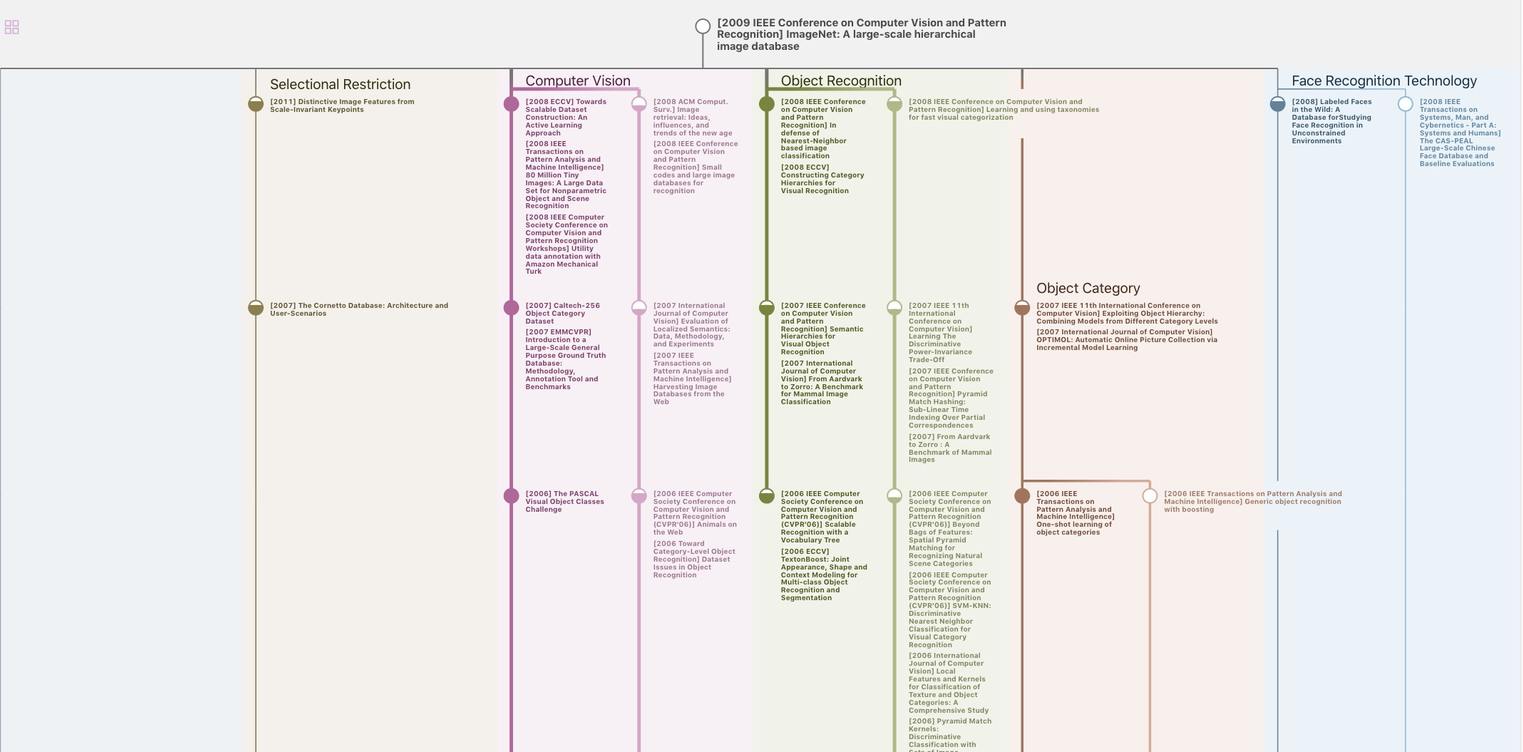
生成溯源树,研究论文发展脉络
Chat Paper
正在生成论文摘要