Accelerant Facilitation for an Adaptive Weighting-Based Multi-Index Assessment of Cyber Physical Power Systems
2023 IEEE 3rd International Conference in Power Engineering Applications (ICPEA)(2023)
摘要
The determination of criteria weights is known to be a challenging and complex issue, particularly for the Multi-Criteria Decision Making (MCDM) problems involved in the assessment of Cyber Physical Power Systems (CPPS). Traditionally, assessment methodologies for CPPS have often leveraged a Multi-Index Assessment Framework (MIAF) approach, whose involved MCDM problems have various competing objectives and/or attributes that need to be optimized concurrently; yet, the utilized Adaptive Weighting Methodologies (AWM) have often been beset with selection bias (e.g., particular indices utilized, heuristics formulated, parameters selected, etc). The selection bias issue has been compounded even further when the utilized Artificial Intelligence (AI) decision-support mechanisms also have inherent selection bias; this phenomenon has contributed to the Explainable AI (XAI) movement. This paper’s mitigation approach is two-pronged. First, a mitigation approach to the AWM selection bias centers upon the use of an AWM that considers/hybridizes various subjective and objective approaches. For example, a bespoke Technique for Order of Preference by Similarity to Ideal Solution (TOPSIS)-CRiteria Importance through Intercriteria Correlation (CRITIC) et al. hybridized construct (C#1) conjoined with a bespoke R-Fuzzy Set (RFS), Adaptation of the Grey Relational Analysis (AGRA), and New Normalized Projection Entropy Weighting Model (NNP-EWM) et al. construct (C#2) can reduce the AWM selection bias. Along with AGRA, C#2 can leverage Type-2 Fuzzy Sets (T2FS) and Spherical Fuzzy Set (SFS) AWM-derived entropy weights to support C#1. This paper further facilitates the C#1 and C#2 constructs with an Extended Matrix Shanks Transformation Accelerant (EMSTA). Second, a mitigation approach to the involved AI selection bias is to leverage a bespoke AWM Support Layer (ASL) in the form of a Particle Swarm Optimization-based Robust Convex Relaxation (PSO-RCR) pseudo-white-box construct, which operationalizes the tightest possible relaxation for the involved successive neural network convolutional layers (which contain the cascading of ever-smaller Continuous Wavelet Transform-like convolutional filters). Hence, both the AWM and AI selection bias are reduced, and a principal contribution of this paper is the enhanced efficacy of the MIAF for use with CPPS, particularly those which are potentially vulnerable to a Sequential Topology Attack (STA) effectuated by an Advanced Persistent Threat (APT) Insider Threat Paradigm (ITP). Preliminary results indicate that the C#1 and C#2 amalgam has high efficacy, and the performance, numerical stability, and consistency of the results — when leveraging EMSTA — is promising.
更多查看译文
关键词
Smart Grid,Adaptive Weighting Methodology,Artificial Intelligence,Power System Operations and Planning,Decision Engineering,Data Analytics in Power Engineering
AI 理解论文
溯源树
样例
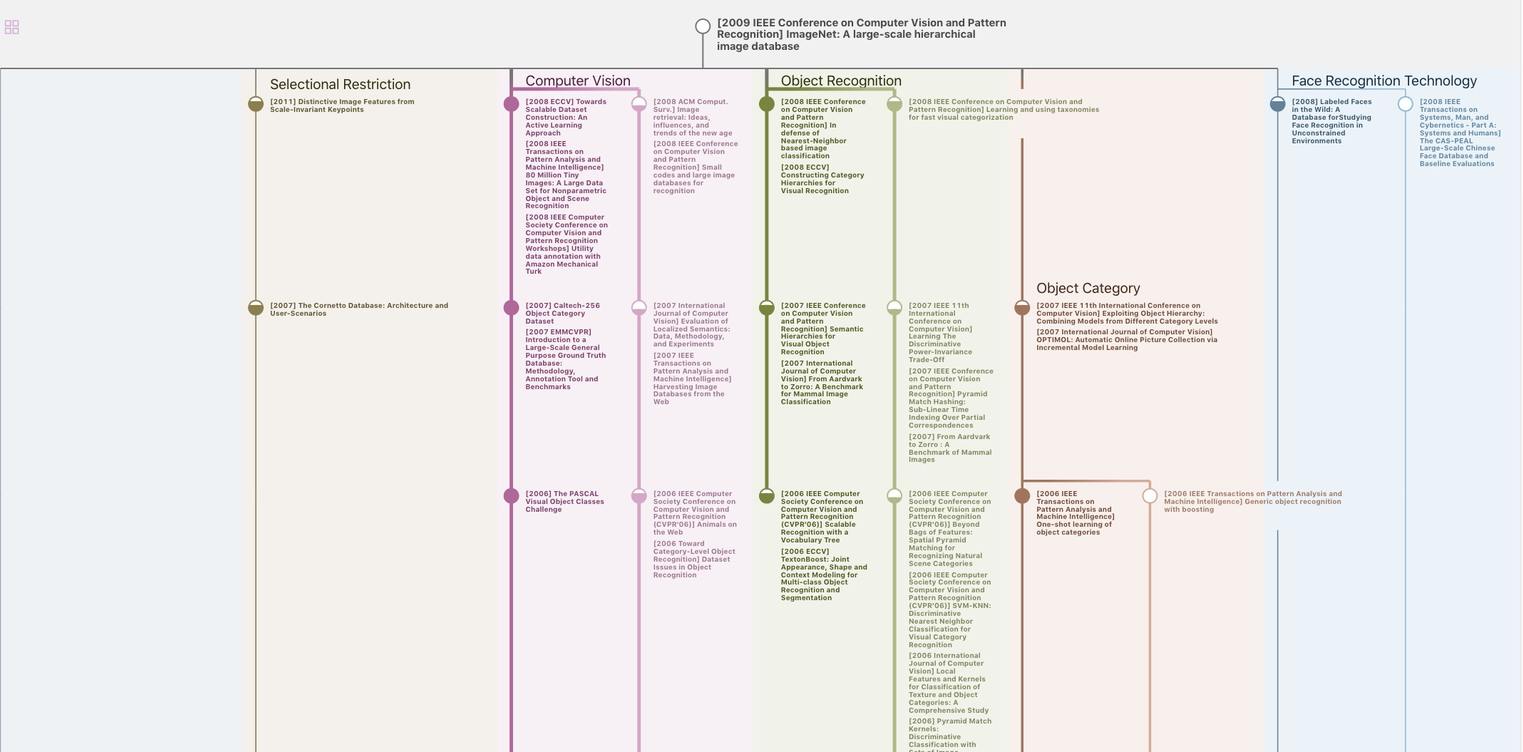
生成溯源树,研究论文发展脉络
Chat Paper
正在生成论文摘要