Exploring the Effect of Adversarial Attacks on Deep Learning Architectures for X-Ray Data
2022 IEEE Applied Imagery Pattern Recognition Workshop (AIPR)(2022)
摘要
As artificial intelligent models continue to grow in their capacity and sophistication, they are often trusted with very sensitive information. In the sub-field of adversarial machine learning, developments are geared solely towards finding reliable methods to systematically erode the ability of artificial intelligent systems to perform as intended. These techniques can cause serious breaches of security, interruptions to major systems, and irreversible damage to consumers. Our research evaluates the effects of various white box adversarial machine learning attacks on popular computer vision deep learning models leveraging a public X-ray dataset from the National Institutes of Health (NIH). We make use of several experiments to gauge the feasibility of developing deep learning models that are robust to adversarial machine learning attacks by taking into account different defense strategies, such as adversarial training, to observe how adversarial attacks evolve over time. Our research details how a variety white box attacks effect different components of InceptionNet, DenseNet, and ResNeXt and suggest how the models can effectively defend against these attacks.
更多查看译文
关键词
adversarial machine learning,ML,attacks,baseline,computer vision,AI,security
AI 理解论文
溯源树
样例
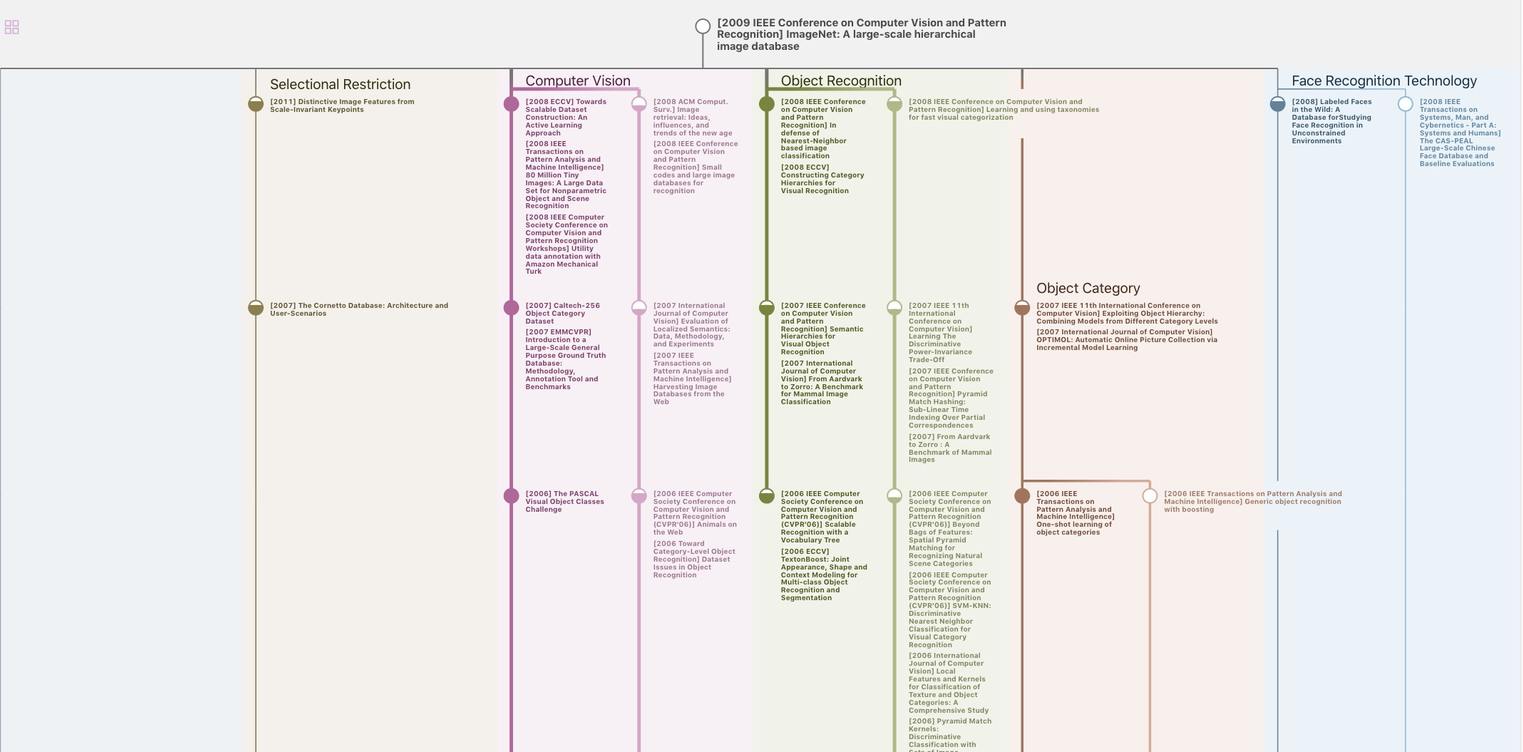
生成溯源树,研究论文发展脉络
Chat Paper
正在生成论文摘要