Achieving Adversarial Robustness in Deep Learning-Based Overhead Imaging
2022 IEEE Applied Imagery Pattern Recognition Workshop (AIPR)(2022)
摘要
The Intelligence, Surveillance, and Reconnaissance (ISR) community relies heavily on the use of overhead imagery for object detection and classification. In these applications, machine learning frameworks have been increasingly used to assist analysts in distinguishing high value targets from mundane objects quickly and effectively. In recent years, the robustness of these frameworks has come under question due to the possibility for disruption using image-based adversarial attacks, and as such, it is necessary to harden existing models against these threats. In this work, we survey a collection of three techniques to address these concerns at various stages of the image processing pipeline: external validation using Activity Based Intelligence, internal validation using Latent Space Analysis, and adversarial prevention using biologically inspired techniques. We found that biologically-inspired techniques were most effective and generalizable for mitigating adversarial attacks on overhead imagery in machine learning frameworks, with improvements as much as 34.6% over traditional augmentations, and 80.4% over a model without any augmentation-based defense.
更多查看译文
关键词
adversarial attacks,deep learning,automatic target recognition,satellite imaging,biological learning
AI 理解论文
溯源树
样例
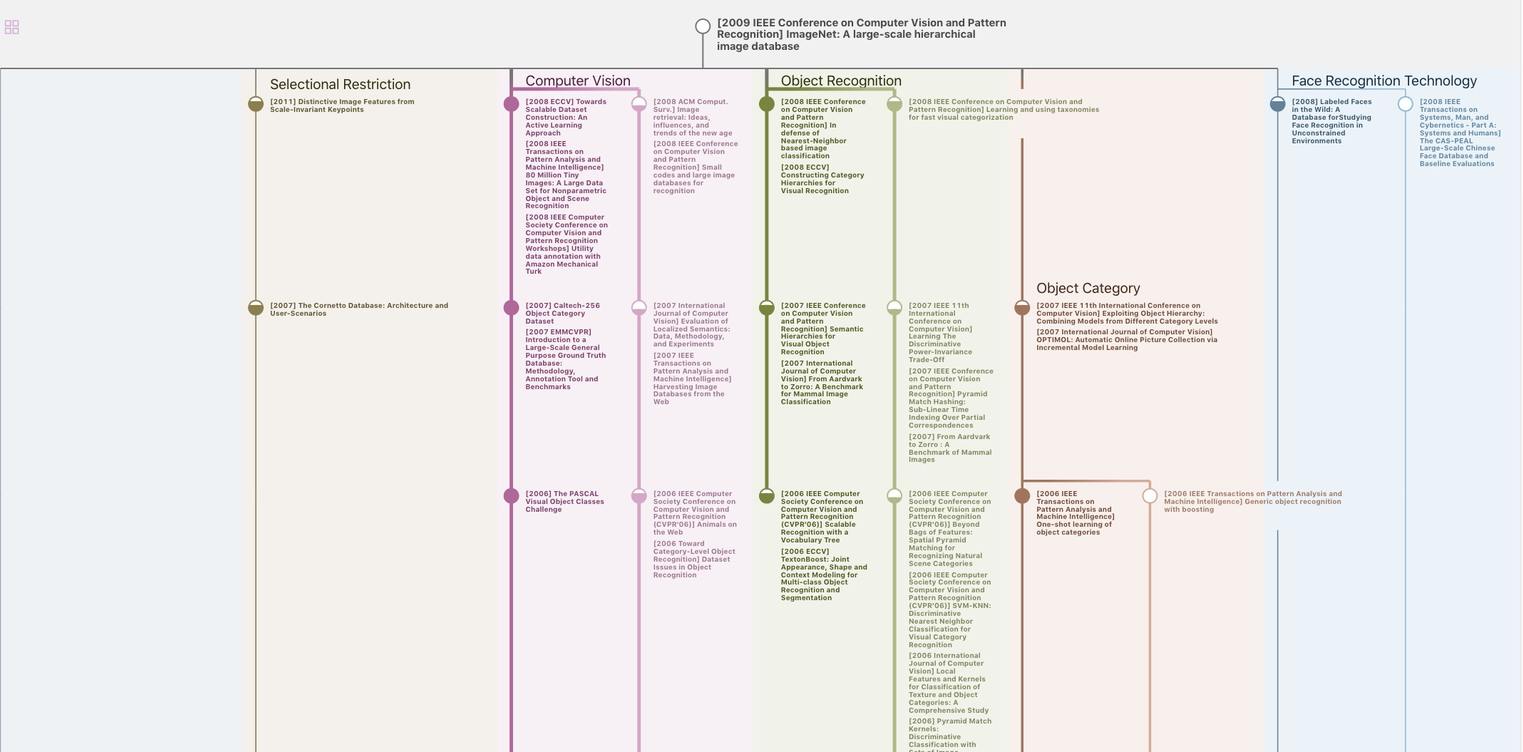
生成溯源树,研究论文发展脉络
Chat Paper
正在生成论文摘要