ML-AWARE: A Machine Learning Approach for Detecting Wormhole Attack Resonance
2022 IEEE Conference on Communications and Network Security (CNS)(2022)
摘要
Internet of Things (IoT), such as a UAV swarm and a car platoon, comprises intelligent mobile and wireless devices with highly heterogeneous sensors and actuators. For managing them, logically centralized management such as Software-defined Network (SDN) is more beneficial than conventional distributed and ad hoc management approaches in terms of performance, scalability, and flexibility. However, the security issues for centralized management of heterogeneous IoT systems have not been explored well. The existing centralized security countermeasures are not enhancing IoT’s security issues, mainly due to the control path vulnerability. Specifically, a wormhole attack is one of the most challenging yet detrimental security issues in IoT. Attackers can easily manipulate the centralized SDN controllers by spoofing the wireless control messages. In particular, if a wormhole attacker can exploit spoofing attacks against SDN controllers, SDN is hard to detect the wormhole attackers.This paper proposes a novel wormhole countermeasure algorithm named an ML-AWARE (Machine Learning Approach for Detecting Wormhole Attack Resonance) against attackers with various intelligent spoofing capabilities. ML-AWARE proposes a double-step k-means clustering for identifying wormhole attackers, denoising scattered errors and classifying the core area of the wormhole attackers. By clustering with the neighbor counts, ML-AWARE exploits the distance between clusters, concentration patterns, and the size of a cluster. We conducted detailed research using both analysis and simulations. Our simulation results show that ML-AWARE can identify wormhole attackers and counter numerous intelligent wormhole attacks without requiring special devices.
更多查看译文
关键词
IoT,SDN,ML,Security,wormhole attack
AI 理解论文
溯源树
样例
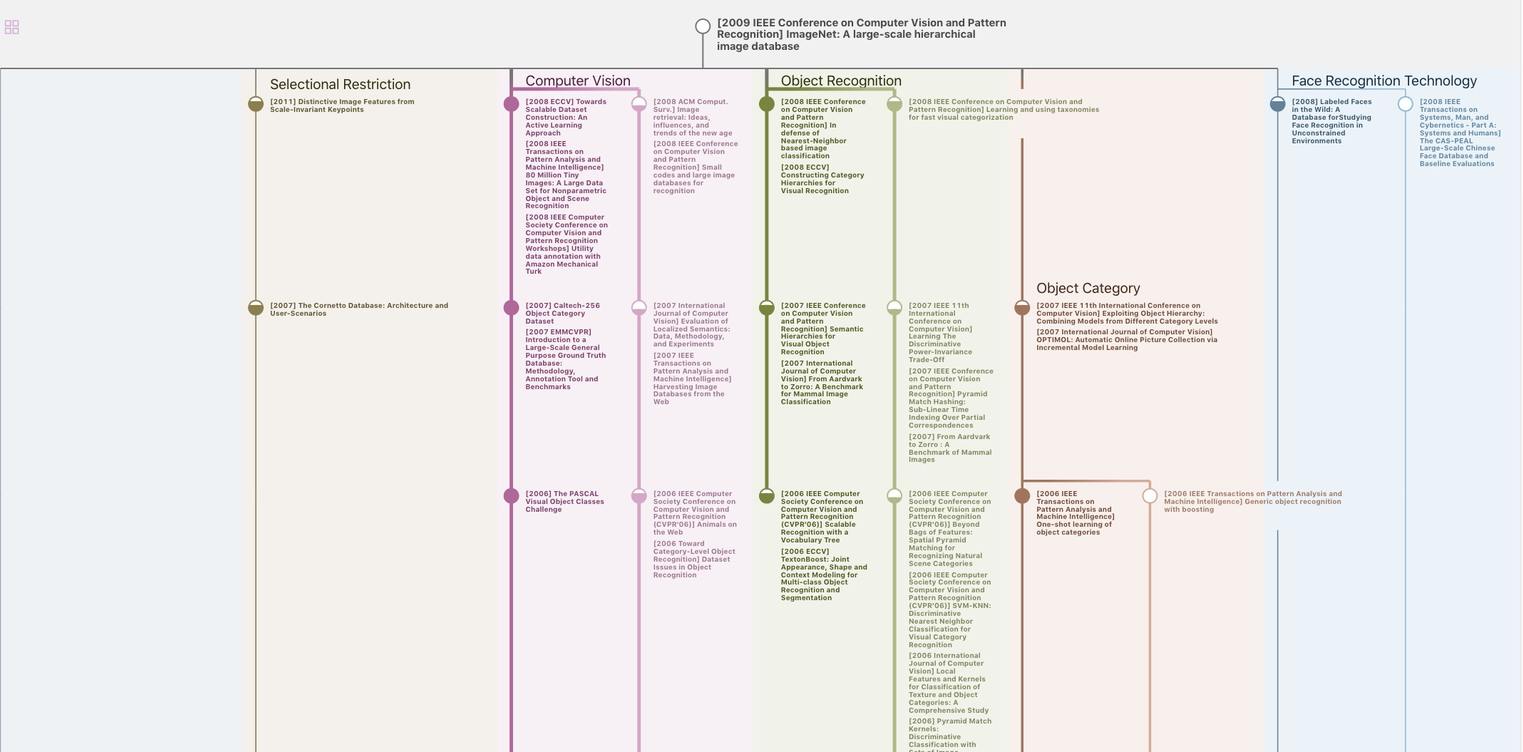
生成溯源树,研究论文发展脉络
Chat Paper
正在生成论文摘要