Linearization of Non-Uniform Quantizers via Adaptive Non-Subtractive Dithering
2023 57th Annual Conference on Information Sciences and Systems (CISS)(2023)
摘要
Non-subtractive dithering is an effective method to improve quantizer performance by injecting input noise that reduces statistical correlations between input signals and quantization error. Existing non-subtractive dither theory has primarily designed dither signal distributions for linear, uniform quantizers, neglecting real-world non-idealities including non-uniformity and finite-level saturation. We develop a generalized analytical condition to guarantee independence of the quantization error moments from the input signal for an arbitrary finite-level non-linear quantizer characteristic. We use this to propose a novel asymmetric, adaptive dither technique for effective linearization of non-uniform quantizers via reduction of the first conditional quantization error moment. These adaptive dither distributions are shown to completely eliminate the first error moment in Lloyd-Max quantizers and significantly reduce it in non-linear quantizers. This allows the use of time-averaging to converge to an arbitrarily precise signal estimate in non-uniform quantizers.
更多查看译文
关键词
dithering,quantization,analog-to-digital conversion,linearization,non-linear,lloyd-max
AI 理解论文
溯源树
样例
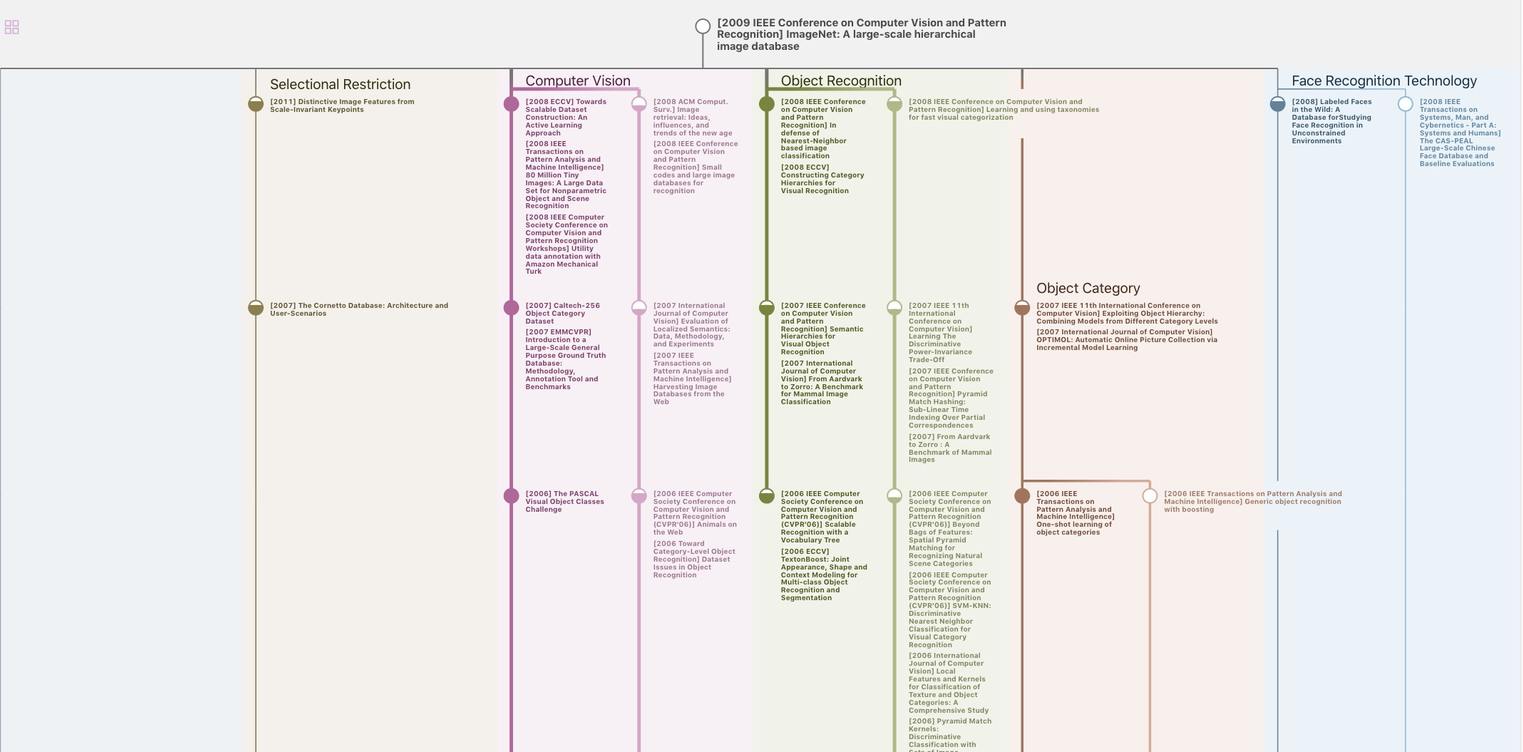
生成溯源树,研究论文发展脉络
Chat Paper
正在生成论文摘要