A Decision Making Model Where the Cell Exhibits Maximum Detection Probability: Statistical Signal Detection Theory and Molecular Experimental Data
2023 57th Annual Conference on Information Sciences and Systems (CISS)(2023)
摘要
Molecular noise and signaling abnormalities in biochemical signaling systems in cells affect signaling events and consequently may alter cellular decision making results. Since unexpected and altered cellular decisions may contribute to the development of many pathological conditions and diseases, it is of interest to develop proper models to characterize and measure molecular signal detection parameters and cellular decisions. In this paper and using the Neyman-Pearson signal detection theorem, we propose a signal detection model in which the cell maximizes its signal detection probability in the presence of noise. To evaluate the usefulness of the proposed model, we use measured molecular experimental data of the important TNF-NF-
$\kappa \mathbf{B}$
cell signaling system. Our results demonstrate that the proposed model provides biologically relevant findings. The introduced Neyman-Pearson-based molecular signal detection framework allows to systematically model and quantify the signal detection behavior and failure of molecular signaling systems, and compute their key decision making parameters such as detection and false alarm probabilities. With regard to the specific TNF-NF-
$\kappa\mathbf{B}$
system case study in this paper and given the high involvement of the transcription factor NF-
$\kappa \mathbf{B}$
in cell survival, programmed cell death, immune signaling and stress response, the developed signal detection framework can serve as a useful tool to model the associated cell decision making processes.
更多查看译文
关键词
cell decision making,biochemical signals,detection theory,Neyman-Pearson detector,A20 deficiency,cancer
AI 理解论文
溯源树
样例
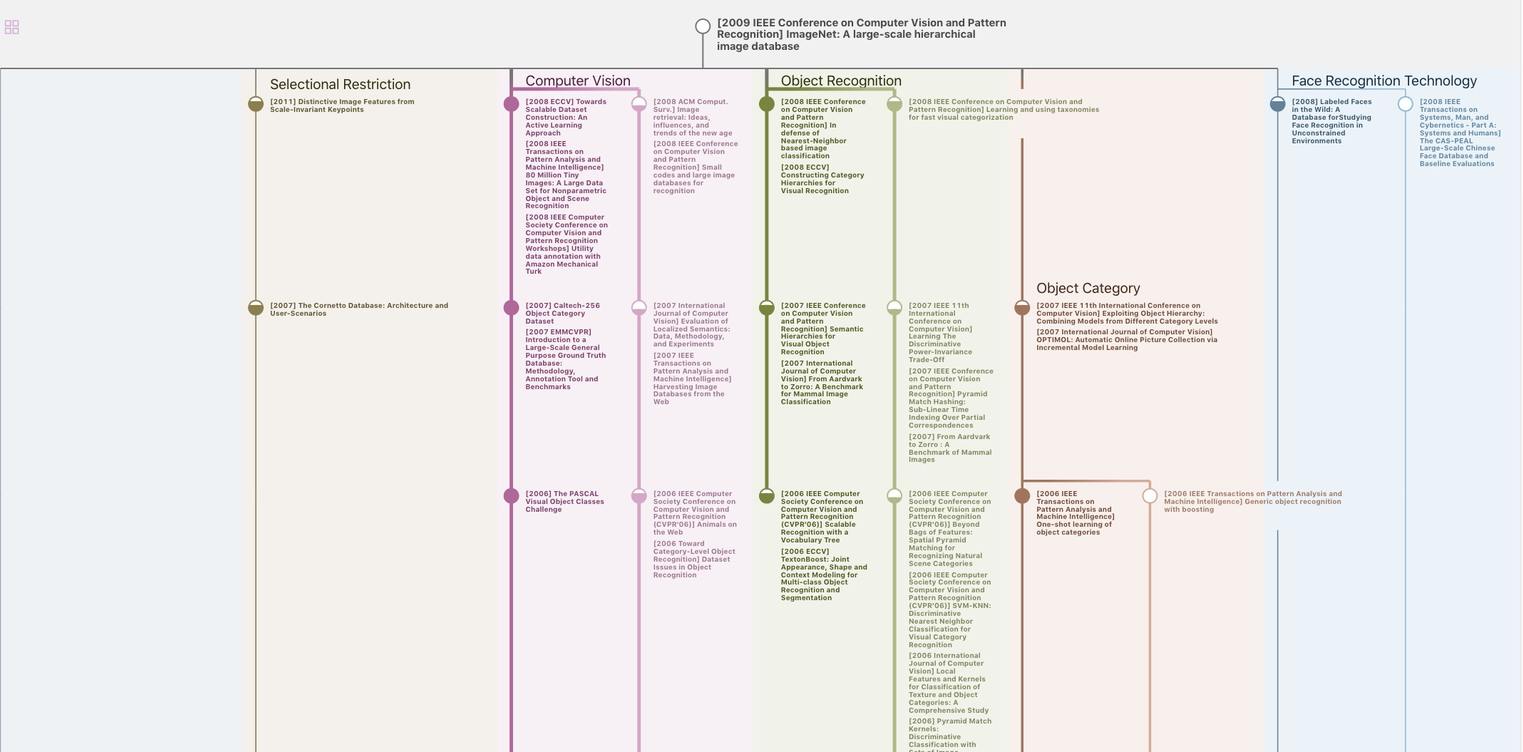
生成溯源树,研究论文发展脉络
Chat Paper
正在生成论文摘要