Integrating Spatial Details With Long-Range Contexts for Semantic Segmentation of Very High-Resolution Remote-Sensing Images
IEEE Geoscience and Remote Sensing Letters(2023)
摘要
This letter presents a cross-learning network (i.e., CLCFormer) integrating fine-grained spatial details within long-range global contexts based upon convolutional neural networks (CNNs) and transformer, for semantic segmentation of very high-resolution (VHR) remote-sensing images. More specifically, CLCFormer comprises two parallel encoders, derived from the CNN and transformer, and a CNN decoder. The encoders are backboned on SwinV2 and EfficientNet-B3, from which the extracted semantic features are aggregated at multiple levels using a bilateral feature fusion module (BiFFM). First, we used attention gate (ATG) modules to enhance feature representation, improving segmentation results for objects with various shapes and sizes. Second, we used an attention residual (ATR) module to refine spatial features’s learning, alleviating boundary blurring of occluded objects. Finally, we developed a new strategy, called auxiliary supervise strategy (ASS), for model optimization to further improve segmentation performance. Our method was tested on the WHU, Inria, and Potsdam datasets, and compared with CNN-based and transformer-based methods. Results showed that our method achieved state-of-the-art performance on the WHU building dataset (92.31% IoU), Inria building dataset (83.71% IoU), and Potsdam dataset (80.27% MIoU). We concluded that CLCFormer is a flexible, robust, and effective method for the semantic segmentation of VHR images. The codes of the proposed model are available at
https://github.com/long123524/CLCFormer
.
更多查看译文
关键词
Auxiliary supervise,CLCFormer,convolutional neural networks (CNNs),semantic segmentation,transformer,very high-resolution (VHR) images
AI 理解论文
溯源树
样例
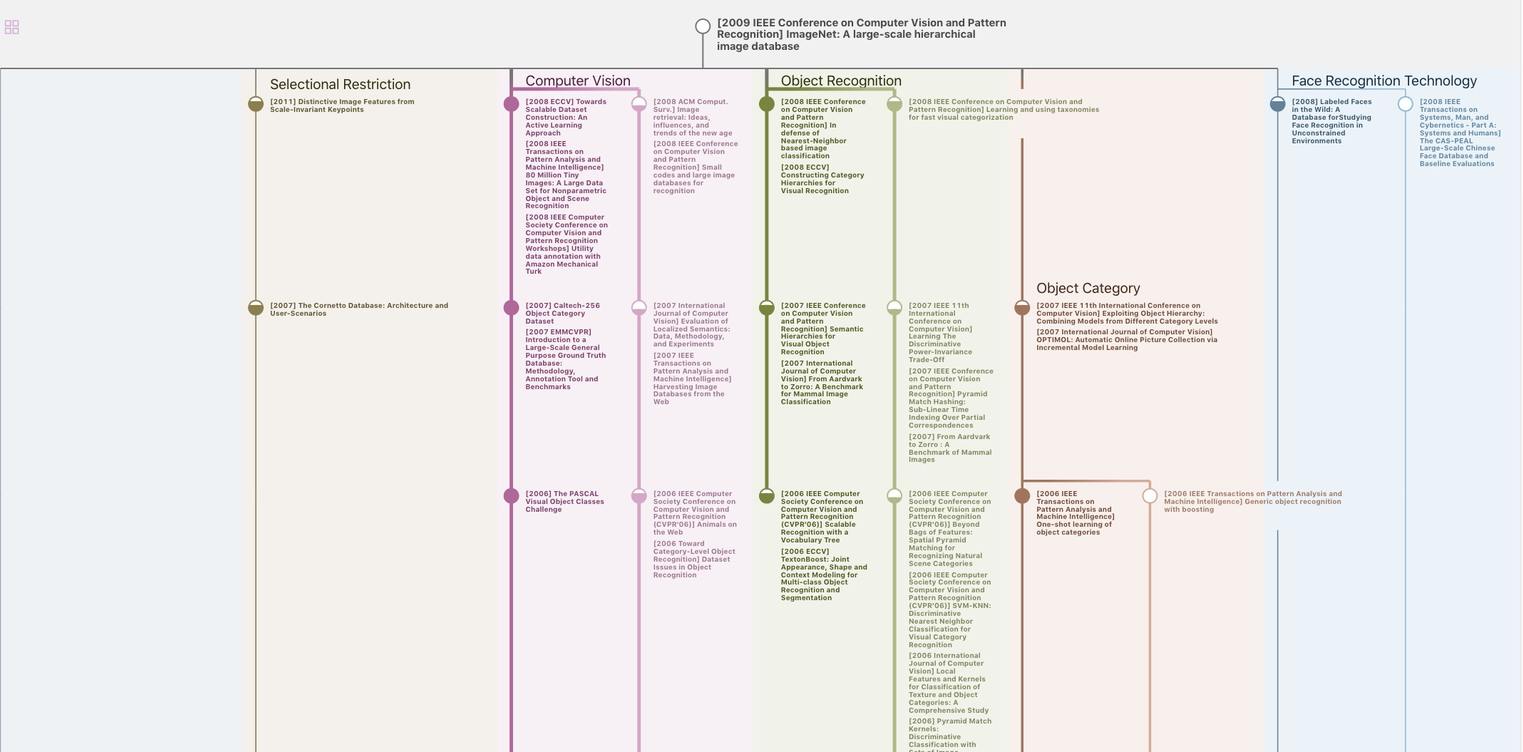
生成溯源树,研究论文发展脉络
Chat Paper
正在生成论文摘要