Reinforcement Learning Quantum Local Search
2023 IEEE International Conference on Quantum Computing and Engineering (QCE)(2023)
摘要
Quantum Local Search (QLS) is a promising approach that employs small-scale quantum computers to tackle large combinatorial optimization problems through local search on quantum hardware, starting from an initial point. However, the random selection of the sub-problem to solve in QLS may not be efficient. In this study, we propose a reinforcement learning (RL) based approach to train an agent for improved subproblem selection in QLS, beyond random selection. Our results demonstrate that the RL agent effectively enhances the average approximation ratio of QLS on fully-connected random Ising problems, indicating the potential of combining RL techniques with Noisy Intermediate-scale Quantum (NISQ) algorithms. This research opens a promising direction for integrating RL into quantum computing to enhance the performance of optimization tasks.
更多查看译文
关键词
quantum,reinforcement,search,learning
AI 理解论文
溯源树
样例
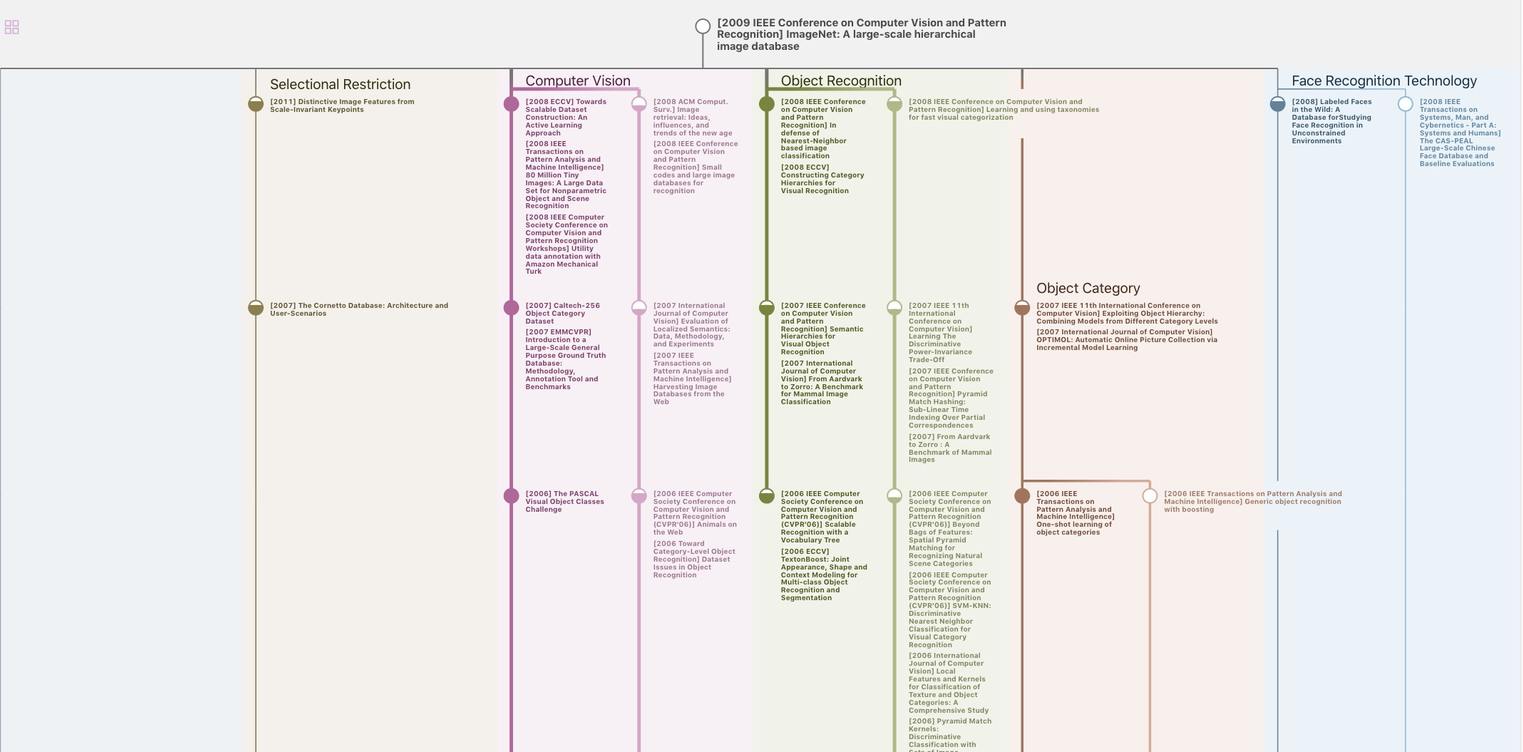
生成溯源树,研究论文发展脉络
Chat Paper
正在生成论文摘要