EWT: Efficient Wavelet-Transformer for single image denoising
Neural Networks(2024)
摘要
Transformer-based image denoising methods have shown remarkable potential but suffer from high computational cost and large memory footprint due to their linear operations for capturing long-range dependencies. In this work , we aim to develop a more resource-efficient Transformer-based image denoising method that maintains high performance. To this end, we propose an Efficient Wavelet Transformer (EWT), which incorporates a Frequency-domain Conversion Pipeline (FCP) to reduce image resolution without losing critical features, and a Multi-level Feature Aggregation Module (MFAM) with a Dual-stream Feature Extraction Block (DFEB) to harness hierarchical features effectively. EWT achieves a faster processing speed by over 80% and reduces GPU memory usage by more than 60% compared to the original Transformer, while still delivering denoising performance on par with state-of-the-art methods. Extensive experiments show that EWT significantly improves the efficiency of Transformer-based image denoising, providing a more balanced approach between performance and resource consumption.
更多查看译文
关键词
Image denoising,Wavelet transform,Dual-stream network
AI 理解论文
溯源树
样例
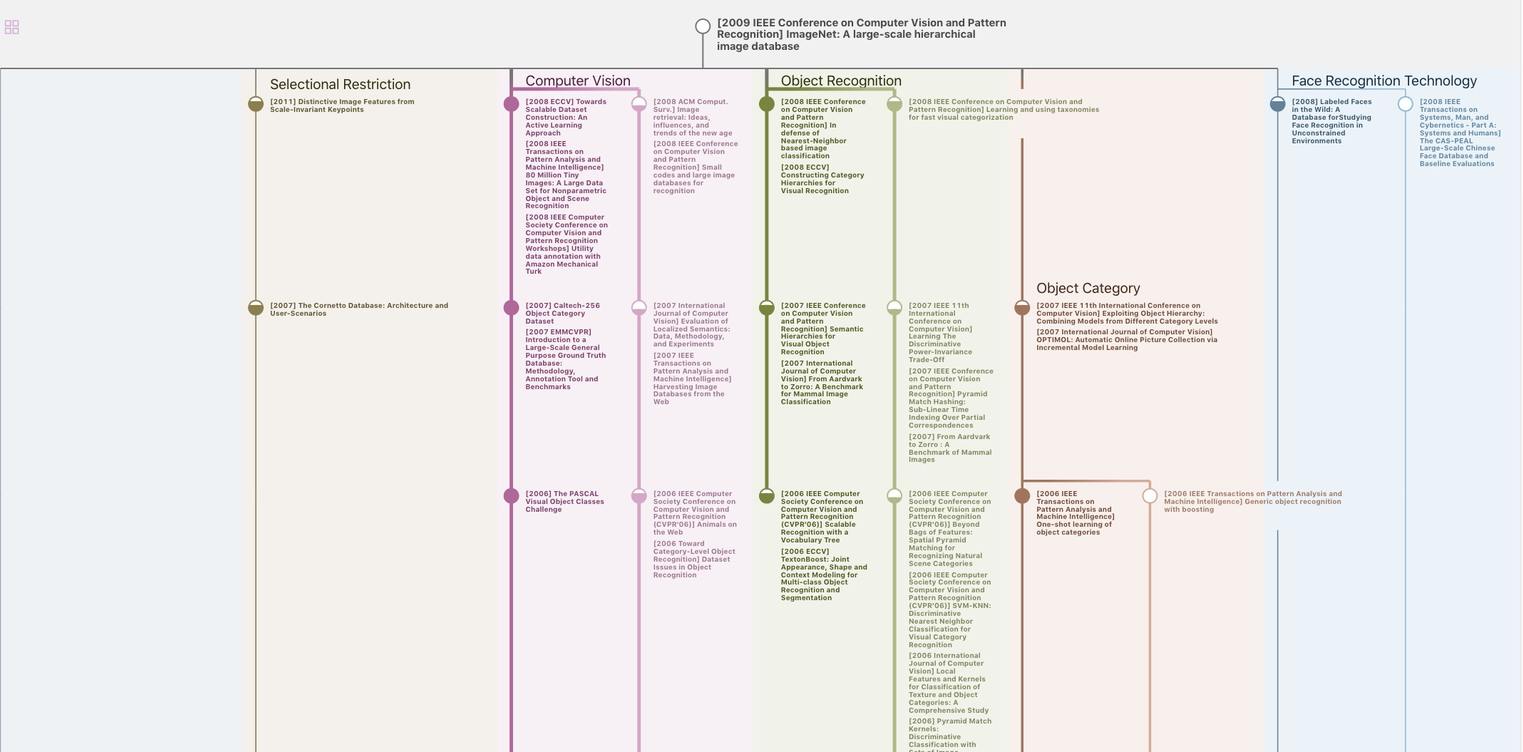
生成溯源树,研究论文发展脉络
Chat Paper
正在生成论文摘要