A physical neural network training approach toward multi-plane light conversion design
arXiv (Cornell University)(2023)
摘要
Multi-plane light converter (MPLC) designs supporting hundreds of modes are attractive in high-throughput optical communications. These photonic structures typically comprise >10 phase masks in free space, with millions of independent design parameters. Conventional MPLC design using wavefront matching updates one mask at a time while fixing the rest. Here we construct a physical neural network (PNN) to model the light propagation and phase modulation in MPLC, providing access to the entire parameter set for optimization, including not only profiles of the phase masks and the distances between them. PNN training supports flexible optimization sequences and is a superset of existing MPLC design methods. In addition, our method allows tuning of hyperparameters of PNN training such as learning rate and batch size. Because PNN-based MPLC is found to be insensitive to the number of input and target modes in each training step, we have demonstrated a high-order MPLC design (45 modes) using mini batches that fit into the available computing resources.
更多查看译文
关键词
light
AI 理解论文
溯源树
样例
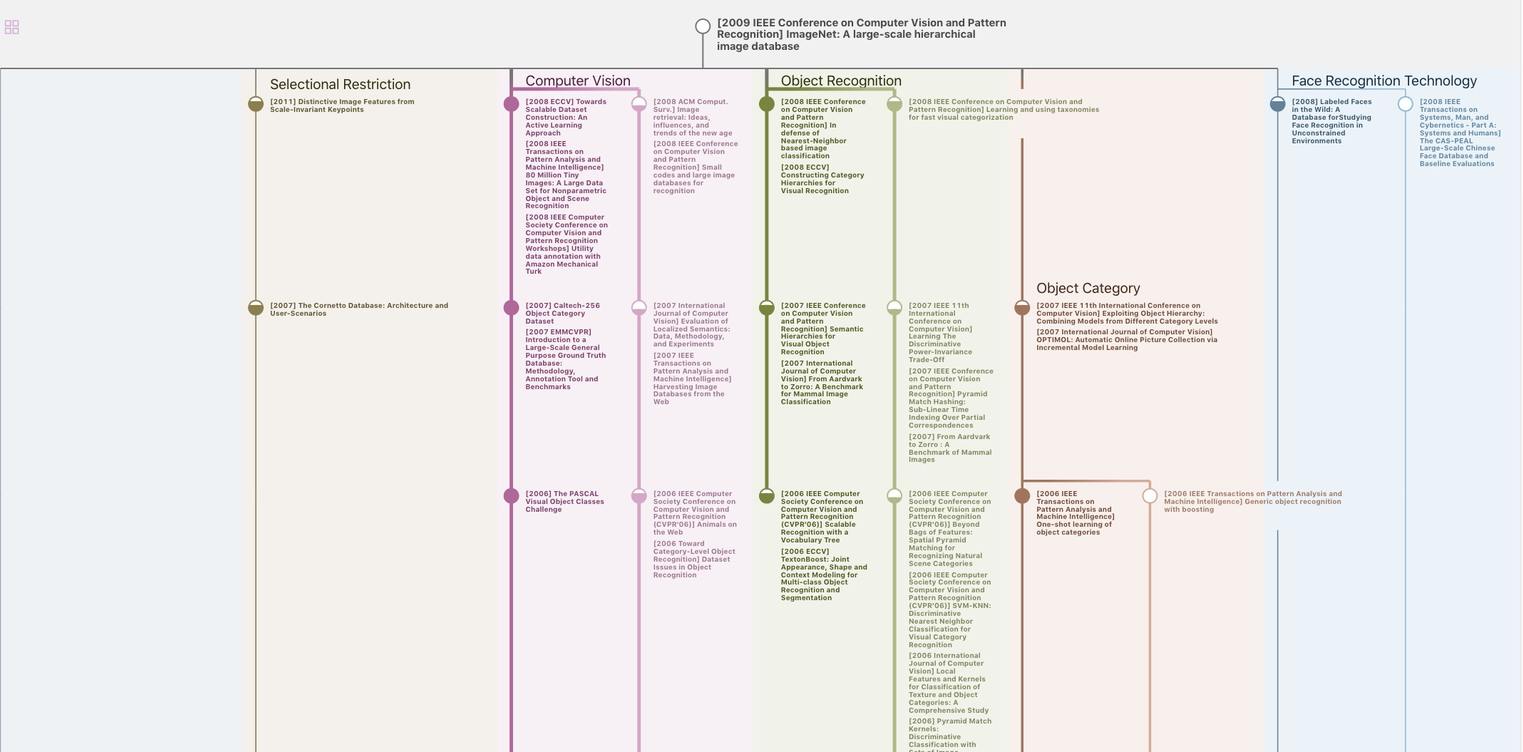
生成溯源树,研究论文发展脉络
Chat Paper
正在生成论文摘要