Multi-source data fusion for insider threat detection using residual networks
2022 3rd International Conference on Electronics, Communications and Information Technology (CECIT)(2022)
Abstract
With the increased diversity of audit data sources and data heterogeneity within organizations, leveraging various data sources and fusing them is critical to improving insider threat detection. The data context between different sources is a part that is often overlooked by existing anomaly detection techniques. In this paper, we propose an insider threat detection method using residual convolutional neural networks for multisource data fusion to discover undetectable combinations of potential threats. The method first transforms tabular data into neighborhood-correlated feature maps, which allows for the contextual information fusion of the same layer by convolutional structure. Skip connections are then added between convolutional layers of different depths for multi-level feature fusion, which allows rich features at lower level to directly share weight information with highly aggregated features at higher levels. We simulated idealistic and realistic conditions for the experiments, and the results on the CERT dataset demonstrate the effectiveness of the proposed method. The insider detection rate is increased by 6% to 8% compared to 1D convolutional neural network models and by 8% to 14% compared to tree-based (Random Forest, XGBoost) models.
MoreTranslated text
Key words
component,insider threat,multi-source data fusion,residual networks,tabular data,skip connection
AI Read Science
Must-Reading Tree
Example
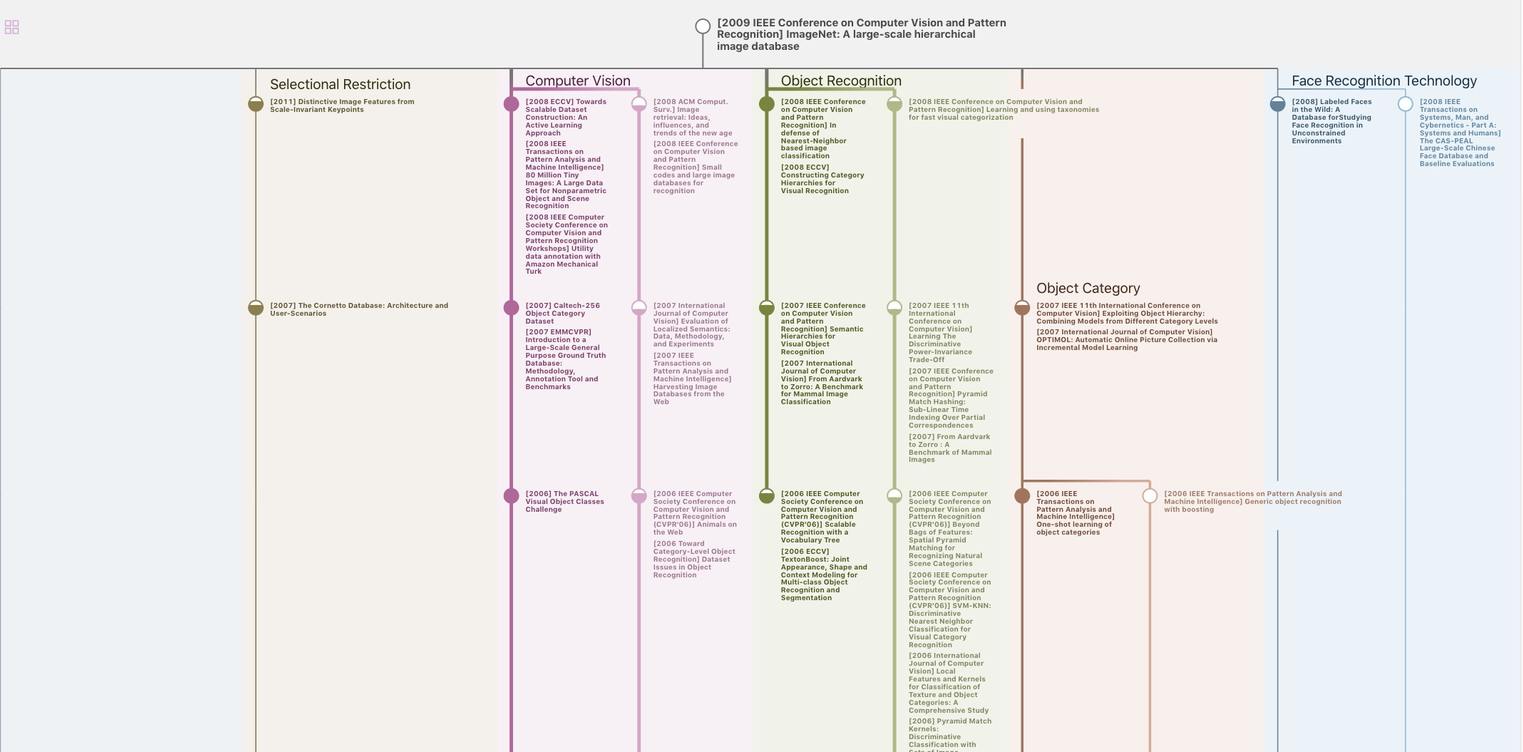
Generate MRT to find the research sequence of this paper
Chat Paper
Summary is being generated by the instructions you defined