Identifying COVID-19 phenotypes using cluster analysis and assessing their clinical outcomes
medRxiv (Cold Spring Harbor Laboratory)(2022)
摘要
Multiple clinical phenotypes have been proposed for COVID-19, but few have stemmed from data-driven methods. We aimed to identify distinct phenotypes in patients admitted with COVID-19 using cluster analysis, and compare their respective characteristics and clinical outcomes.
We analyzed the data from 547 patients hospitalized with COVID-19 in a Canadian academic hospital from January 1, 2020, to January 30, 2021. We compared four clustering algorithms: K-means, PAM (partition around medoids), divisive and agglomerative hierarchical clustering. We used imaging data and 34 clinical variables collected within the first 24 hours of admission to train our algorithm. We then conducted survival analysis to compare clinical outcomes across phenotypes and trained a classification and regression tree (CART) to facilitate phenotype interpretation and phenotype assignment.
We identified three clinical phenotypes, with 61 patients (17%) in Cluster 1, 221 patients (40%) in Cluster 2 and 235 (43%) in Cluster 3. Cluster 2 and Cluster 3 were both characterized by a low-risk respiratory and inflammatory profile, but differed in terms of demographics. Compared with Cluster 3, Cluster 2 comprised older patients with more comorbidities. Cluster 1 represented the group with the most severe clinical presentation, as inferred by the highest rate of hypoxemia and the highest radiological burden. Mortality, mechanical ventilation and ICU admission risk were all significantly different across phenotypes.
We conducted a phenotypic analysis of adult inpatients with COVID-19 and identified three distinct phenotypes associated with different clinical outcomes. Further research is needed to determine how to properly incorporate those phenotypes in the management of patients with COVID-19.
### Competing Interest Statement
The authors have declared no competing interest.
### Funding Statement
M.C. is supported by a Fonds de Recherche Québec Santé (FRQS) Clinical Research scholarship. M.D. is supported by a FRQS Clinical Research scholarship. A.T. is supported by a FRQS Clinical Research Scholarship and a Fondation de l'Association des Radiologistes du Québec (FARQ) Clinical Research Scholarship. This work was partly funded by a Quebec Bio-Imaging Network research grant. The funders had no role in study design, data collection and analysis, decision to publish, or preparation of the manuscript.
### Author Declarations
I confirm all relevant ethical guidelines have been followed, and any necessary IRB and/or ethics committee approvals have been obtained.
Not Applicable
The details of the IRB/oversight body that provided approval or exemption for the research described are given below:
The Institutional Review Board of the CHUM (Centre Hospitalier de l’Université de Montréal) approved the study and informed consent was waived because of its low risk and retrospective nature.
I confirm that all necessary patient/participant consent has been obtained and the appropriate institutional forms have been archived, and that any patient/participant/sample identifiers included were not known to anyone (e.g., hospital staff, patients or participants themselves) outside the research group so cannot be used to identify individuals.
Not Applicable
I understand that all clinical trials and any other prospective interventional studies must be registered with an ICMJE-approved registry, such as ClinicalTrials.gov. I confirm that any such study reported in the manuscript has been registered and the trial registration ID is provided (note: if posting a prospective study registered retrospectively, please provide a statement in the trial ID field explaining why the study was not registered in advance).
Not Applicable
I have followed all appropriate research reporting guidelines and uploaded the relevant EQUATOR Network research reporting checklist(s) and other pertinent material as supplementary files, if applicable.
Not Applicable
The entire code excluding the dataset is publicly available on GitHub () The data that support the findings of this study are available on request from the corresponding author, MC.
* APN
: average proportion of non-overlap
AD
: average distance
ADM
: average distance between means
CART
: classification and regression tree
CCI
: Charlson Comorbidity Index
CXR
: chest radiographs
FAMD
: factor analysis of mixed data
FOM
: figure of merit
ICU
: intensive care unit
MCI
: Medicines Comorbidity Index
MV
: mechanical ventilation
NLR
: neutrophil-to-lymphocyte ratio
PAM
: partition around medoids
PCR
: polymerase chain reaction
POLST
: Physician Orders for Life-Sustaining Treatment
VIA
: variable importance analysis
更多查看译文
关键词
cluster analysis,clinical outcomes
AI 理解论文
溯源树
样例
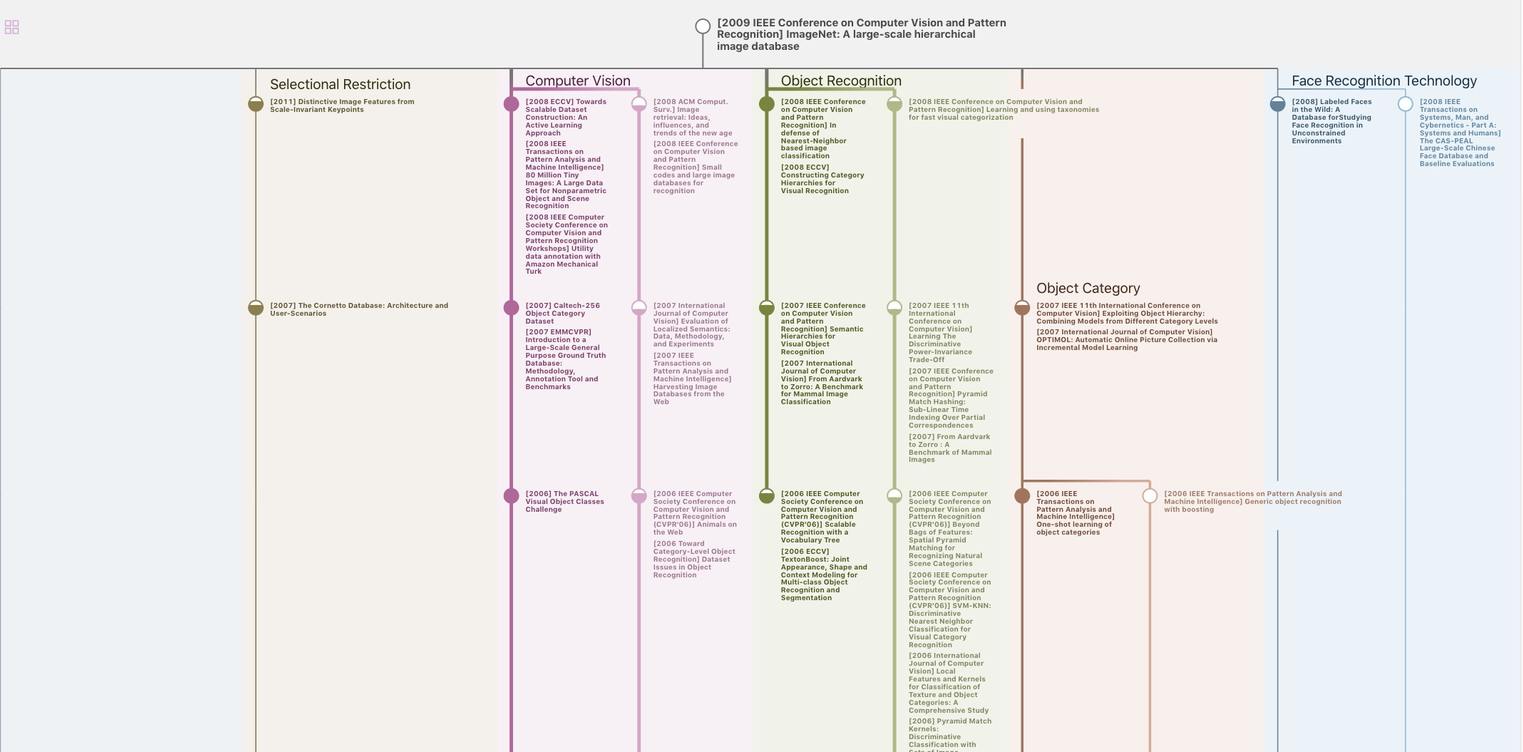
生成溯源树,研究论文发展脉络
Chat Paper
正在生成论文摘要