A deep learning approach to identify seizure-prone and normal patients from their EEG records
medrxiv(2022)
摘要
Various learning models distinguish between an electroencephalogram (EEG) record of a normal patient and one having a seizure. In this paper, we propose a deep-learning based short-term memory (LSTM) model to identify whether an EEG record belongs to a seizure-prone patient with a non-seizure record or to a normal patient. The study builds on two datasets, namely the TUH Abnormal EEG Corpus (TUAB) and the TUH EEG Seizure Corpus (TUSZ) including the classified EEG records for seizure-prone and normal patients. We conducted experiments on both imbalanced and balanced datasets and show results using an LSTM model. We observed that the model performs consistently in both balanced and imbalanced cases using only 5 seconds of EEG data from the patient records. We show that our proposed LSTM model gives test accuracies up to 99.84% in case of 2-class classification between the non-seizure and normal classes and up to 98.87% in case of 3-class classification among non-seizure, seizure, and normal classes. This provides a basis for making improved temporal predictions about the occurrences of seizures.
### Competing Interest Statement
The authors have declared no competing interest.
### Funding Statement
This work utilizes resources supported by the National Science Foundation's Major Research Instrumentation program, grant #1725729, as well as the University of Illinois at Urbana-Champaign.
### Author Declarations
I confirm all relevant ethical guidelines have been followed, and any necessary IRB and/or ethics committee approvals have been obtained.
Yes
The details of the IRB/oversight body that provided approval or exemption for the research described are given below:
I confirm that all necessary patient/participant consent has been obtained and the appropriate institutional forms have been archived, and that any patient/participant/sample identifiers included were not known to anyone (e.g., hospital staff, patients or participants themselves) outside the research group so cannot be used to identify individuals.
Yes
I understand that all clinical trials and any other prospective interventional studies must be registered with an ICMJE-approved registry, such as ClinicalTrials.gov. I confirm that any such study reported in the manuscript has been registered and the trial registration ID is provided (note: if posting a prospective study registered retrospectively, please provide a statement in the trial ID field explaining why the study was not registered in advance).
Yes
I have followed all appropriate research reporting guidelines and uploaded the relevant EQUATOR Network research reporting checklist(s) and other pertinent material as supplementary files, if applicable.
Yes
All datasets are available online at:
更多查看译文
关键词
deep learning,deep learning approach,eeg,seizure-prone
AI 理解论文
溯源树
样例
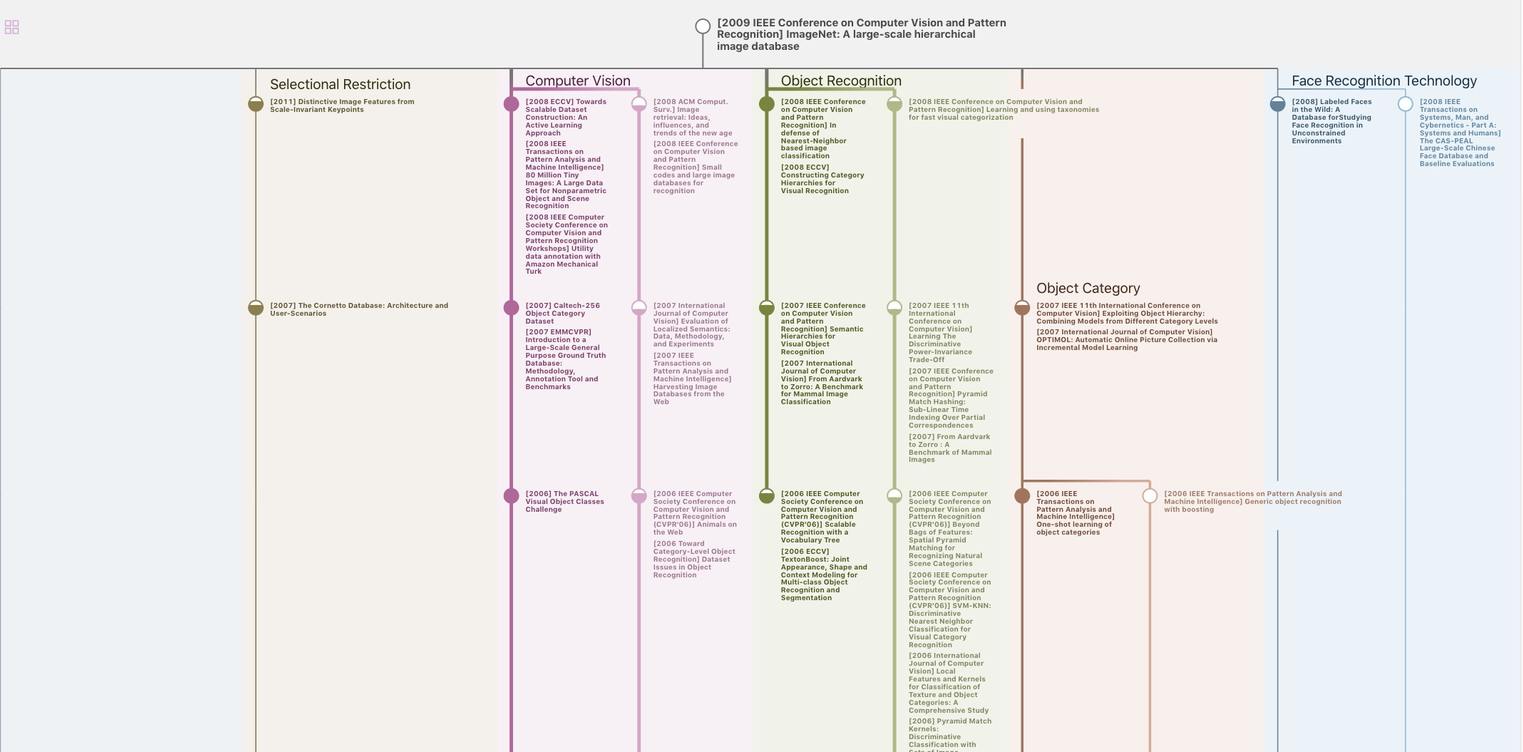
生成溯源树,研究论文发展脉络
Chat Paper
正在生成论文摘要