Benign and Malignant Skin Lesion Detection from Melanoma Skin Cancer Images
2023 International Conference for Advancement in Technology (ICONAT)(2023)
摘要
Skin cancer is the most dangerous and lethal cancer that affects millions of people each year. The accurate identification of skin cancers can not be accomplished without expert dermatologists. However, specific research studies of WHO in Canada, US and Australia, show that in the year 1960s to 1980s, the cases of skin cancer has noted more than two times increased in comparison with the previous years. The identification of skin cancer in its early stage is an expensive and difficult task because it doesn’t cause too much bad in the initial phase. Whereas, the growth of skin cancer requires biopsy and many other treatments each time which is quite costly as per the statistics of India. This challenge makes it a necessary step to identify the existence of skin cancer in the early stages to increase immortality. With the evolution and progression in technology, there are various methods which have participated in and solved medical issues including covid19, pneumonia and many others. Similarly, machine learning(ML) and deep learning(DL) models are applicable to diagnosing skin cancer in its early stages. In this work, the support vector machine (SVM), naive bayes (NB), K-nearest neighbour (KNN) and neural networks(NN) have been used for classifying benign and malignant lesions. Furthermore, for the feature extraction from the dataset, a pre-trained SqueezeNet model has been used. The classification results of KNN, SVM, NB and NN have been shown in the accuracy, recall, F1-Measure, precision, AUC and ROC. The comparison of the models has resulted that the NN model outperforms all other models when applied with the SqueezeNet feature extractor with the highest accuracy, F1-Measure, recall, precision and AUC as 88.2%, 0.882, 0.882, 0.882 and 0.957, respectively. Lastly, the performance metrics analogies results of each model have been illustrated for the classification of benign and malignant lesions.
更多查看译文
关键词
SqueezeNet,neural networks,skin cancer,deep learning,machine learning
AI 理解论文
溯源树
样例
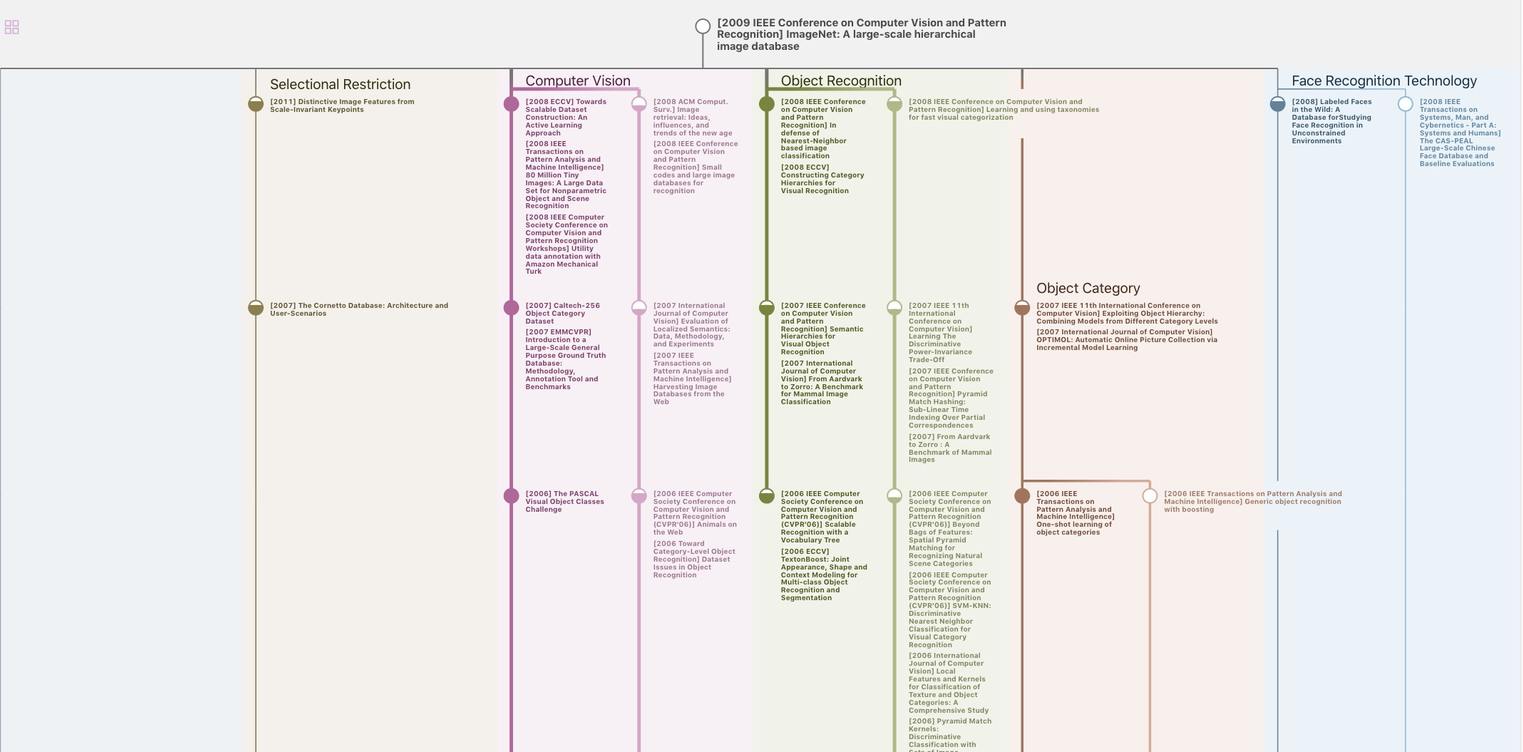
生成溯源树,研究论文发展脉络
Chat Paper
正在生成论文摘要