Points-Based Models for Predicting Severe Disease related to Covid-19 with the SARS-CoV-2 Omicron Variant
medRxiv (Cold Spring Harbor Laboratory)(2022)
摘要
Introduction Throughout the SARS-CoV-2 pandemic, resources for various aspects of patient care have been limited, necessitating risk-stratification. The need for good risk-stratification tools has been enhanced by the availability of new Covid-19 therapeutics that are effective at preventing severe disease among high-risk patients if given promptly following SARS-CoV-2 infection. We describe the development of two points-based models for predicting the risk of deterioration to severe disease from an Omicron-variant SARS-CoV-2 infection.
Methods We developed two logistic regression-based models for predicting the risk of severe Covid-19 within a 21-days follow-up period among Clalit Health Services members aged 18 and older, with confirmed SARS-CoV-2 infection from December 25, 2021 to March 16, 2022. In the first model, aimed for the use of healthcare providers, the model coefficients were linearly transformed into integer risk points. In the second model, a simplified version designed for self-assessment by the general public, the risk points were further scaled down to smaller numbers with less variability across risk factors.
Results 613,513 individuals met the inclusion criteria, of which 1,763 (0.287%) developed the outcome. The AUROC estimates for both models were 0.95, although the ‘full’ model demonstrated more granular risk-stratification capabilities (77 vs. 27 potential thresholds on the test set). Both models proved effective in identifying small subsets of the population enriched with individuals who ended up deteriorating. For example, prioritizing the top 1%, 5% or 10% individuals in the population for interventions with the full model results in coverage of 36%, 68% or 83% (respectively) of the individuals that actually end up deteriorating. Risk point count increased with age, number of chronic conditions and previous hospitalizations, and decreased with recent vaccination and infection.
Discussion The models presented, one more expressive and one more accessible, are transparent and explainable models applicable to the general population that can be used in the prioritization of Covid-19-related resources, including therapeutics.
### Competing Interest Statement
The authors have declared no competing interest.
### Funding Statement
This study did not receive any funding
### Author Declarations
I confirm all relevant ethical guidelines have been followed, and any necessary IRB and/or ethics committee approvals have been obtained.
Yes
The details of the IRB/oversight body that provided approval or exemption for the research described are given below:
Institutional Ethics Committee of Clalit Health Services gave ethical approval (0052-20-COM2 Appendix 2) for this work
I confirm that all necessary patient/participant consent has been obtained and the appropriate institutional forms have been archived, and that any patient/participant/sample identifiers included were not known to anyone (e.g., hospital staff, patients or participants themselves) outside the research group so cannot be used to identify individuals.
Yes
I understand that all clinical trials and any other prospective interventional studies must be registered with an ICMJE-approved registry, such as ClinicalTrials.gov. I confirm that any such study reported in the manuscript has been registered and the trial registration ID is provided (note: if posting a prospective study registered retrospectively, please provide a statement in the trial ID field explaining why the study was not registered in advance).
Yes
I have followed all appropriate research reporting guidelines and uploaded the relevant EQUATOR Network research reporting checklist(s) and other pertinent material as supplementary files, if applicable.
Yes
Owing to data privacy regulations, the raw data for this study cannot be shared.
更多查看译文
关键词
predicting severe disease,points-based,sars-cov
AI 理解论文
溯源树
样例
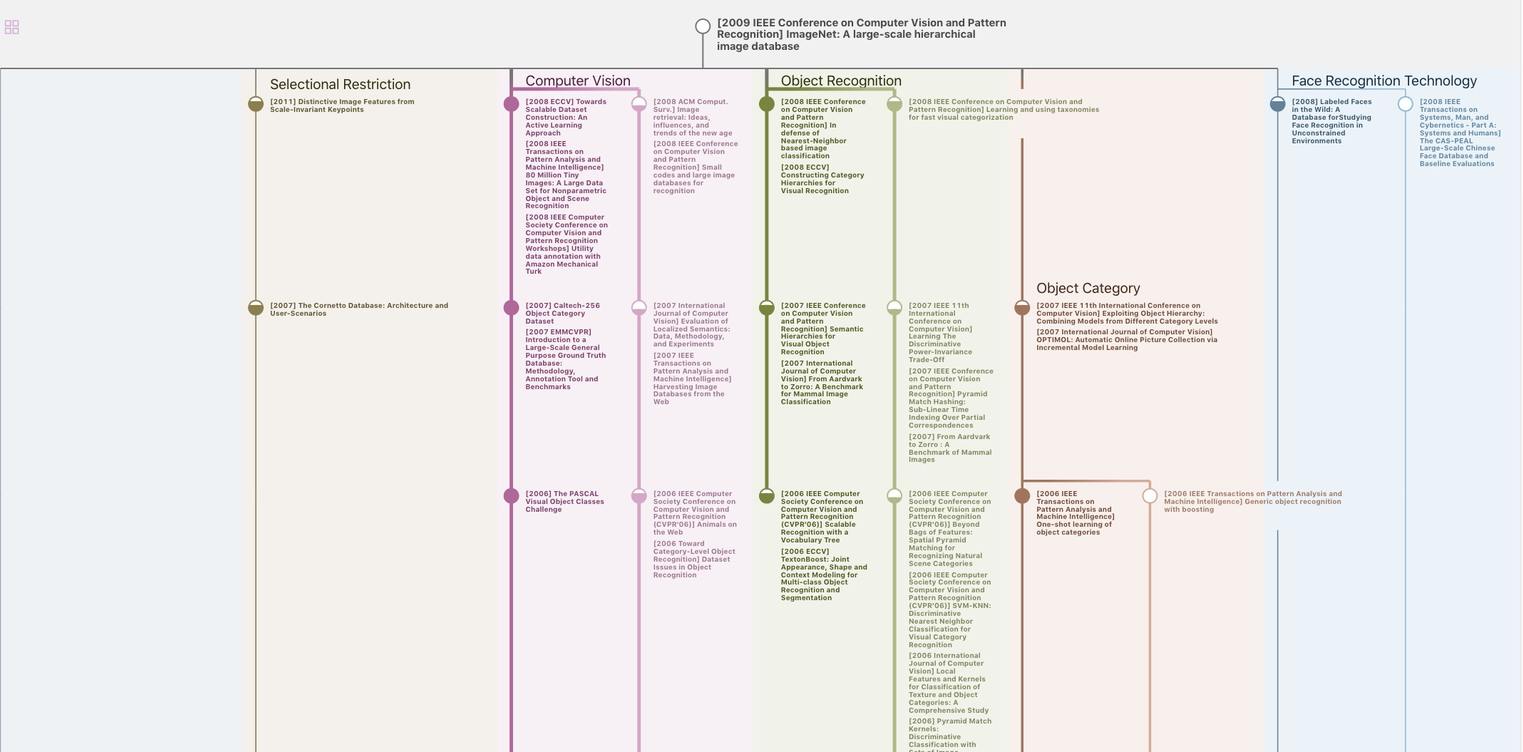
生成溯源树,研究论文发展脉络
Chat Paper
正在生成论文摘要