Using machine learning probabilities to identify effects of COVID-19
PATTERNS(2023)
摘要
Coronavirus disease 2019 (COVID-19), the disease caused by the severe acute respiratory syndrome coro-navirus 2 (SARS-CoV-2) virus, has had extensive economic, social, and public health impacts in the United States and around the world. To date, there have been more than 600 million reported infections worldwide with more than 6 million reported deaths. Retrospective analysis, which identified comorbidities, risk factors, and treatments, has underpinned the response. As the situation transitions to an endemic, retrospective an-alyses using electronic health records will be important to identify the long-term effects of COVID-19. How-ever, these analyses can be complicated by incomplete records, which makes it difficult to differentiate visits where the patient had COVID-19. To address this issue, we trained a random Forest classifier to assign a probability of a patient having been diagnosed with COVID-19 during each visit. Using these probabilities, we found that higher COVID-19 probabilities were associated with a future diagnosis of myocardial infarction, urinary tract infection, acute renal failure, and type 2 diabetes.
更多查看译文
关键词
probabilities,effects
AI 理解论文
溯源树
样例
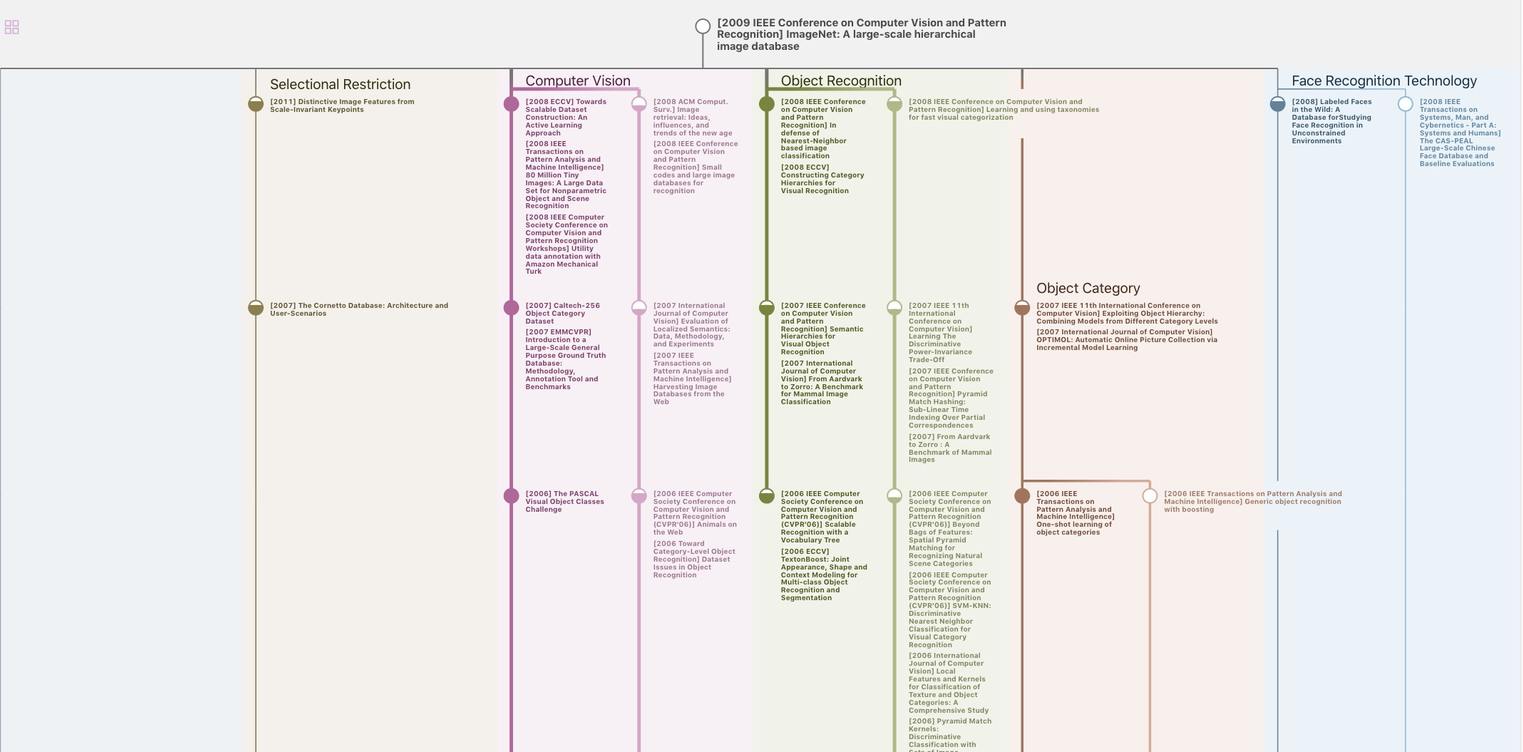
生成溯源树,研究论文发展脉络
Chat Paper
正在生成论文摘要