Energy Theft Detection Using the Wasserstein Distance on Residuals
2023 IEEE Texas Power and Energy Conference (TPEC)(2023)
摘要
Detection of electricity theft improves the sustainability of the smart grid, helps electrical utilities mitigate their financial risks, and improves the overall management of resources. In this work, we utilize an LSTM neural network to forecast a given day’s energy consumption and construct residuals. The residuals are then compared to previous residuals from normal days using the Wasserstein distance. If the Wasserstein distance for the residuals of a day exceeds a threshold, the day is highlighted to indicate suspected energy theft. Our framework can be built upon existing forecasting models with minimal computational overhead to calculate the Wasserstein distance. The framework is also highly explainable, which reduces the cost of false positives significantly. Our framework was evaluated using a public dataset and was able to detect six attack models of energy theft and faulty meters, with a false positive rate of 9% and an average F1 score of 0.91.
更多查看译文
关键词
Energy theft,Non-technical losses,Information distance,Wasserstein distance,Short-term load forecasting
AI 理解论文
溯源树
样例
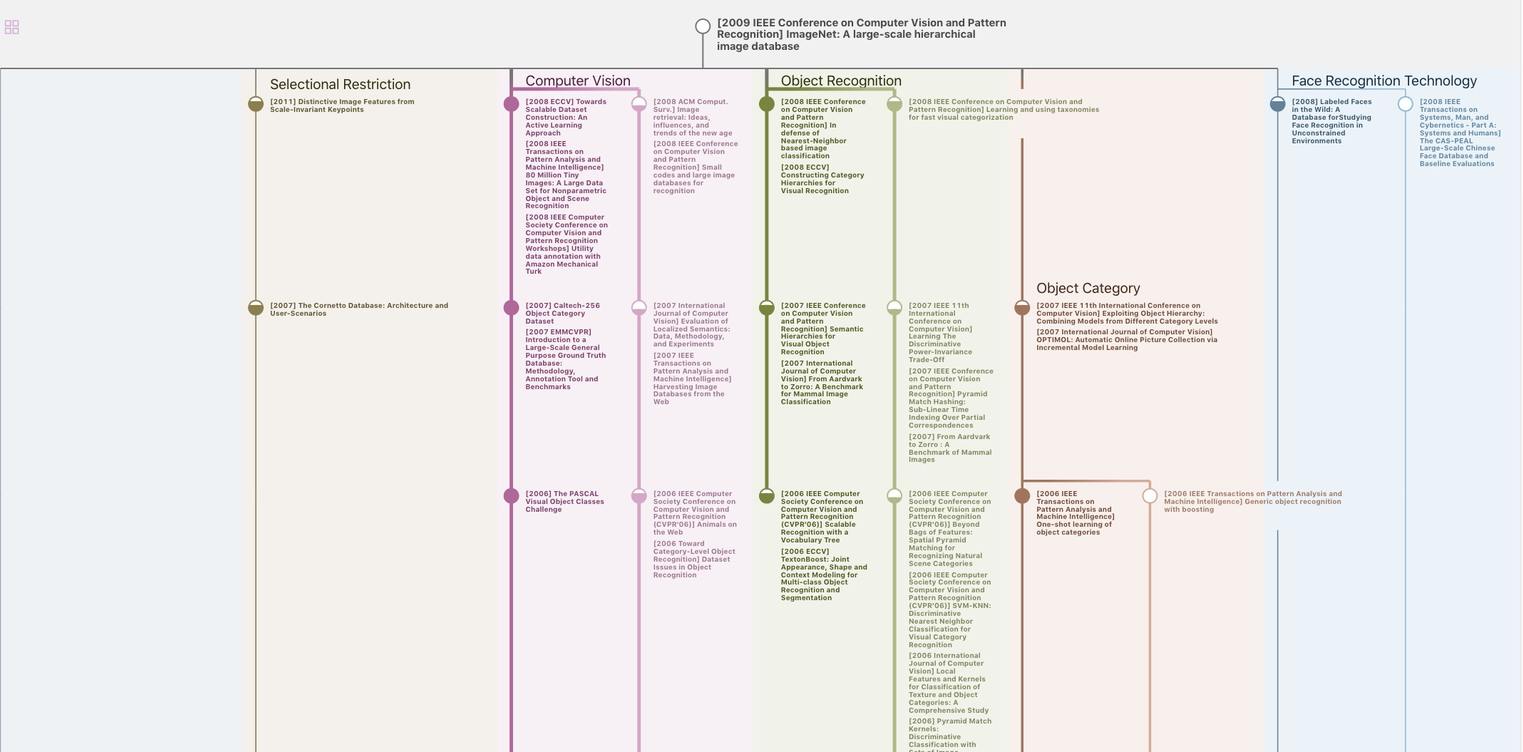
生成溯源树,研究论文发展脉络
Chat Paper
正在生成论文摘要