Reinforcement Learning for Intentional Islanding in Resilient Power Transmission Systems
2023 IEEE Texas Power and Energy Conference (TPEC)(2023)
摘要
Intentional islanding is the process of identifying and deliberately decomposing the transmission network to form self-sustained islands from an endangered network during disruptions to improve resilience and security. Most existing intentional islanding models are offline resilience decision tools and hence do not provide outage responses in a timely manner. In this paper, a reinforcement learning (RL) based model for intentional islanding is developed, which offers real-time switching control, online deployability, and adaptability to varying system conditions. The intentional islanding process is formulated as a Markov decision process, where the optimal transmission switching policy is learned using the RL approach. The control policy is learned over an environment that encompasses a Power System Simulator for Engineering (PSS/E) model of the transmission network, facilitated by an interface to the standard openAI Gym framework. The proposed RL-based methodology aims to form stable and self-sustainable islands by ensuring voltage stability while reducing the power mismatch in the formed islands. A proximal policy optimization algorithm is designed, which is suitable for controlling the on/off status of the switches with multi-layer perceptron as value and actor networks. The effectiveness of the proposed framework in the self-recovery of the grid by island formation is applied on the modified IEEE 39-bus test network and validated by dynamic simulations.
更多查看译文
关键词
Intentional Islanding,Grid Resilience,Reinforcement Learning,OpenAI Gym,PSS/E
AI 理解论文
溯源树
样例
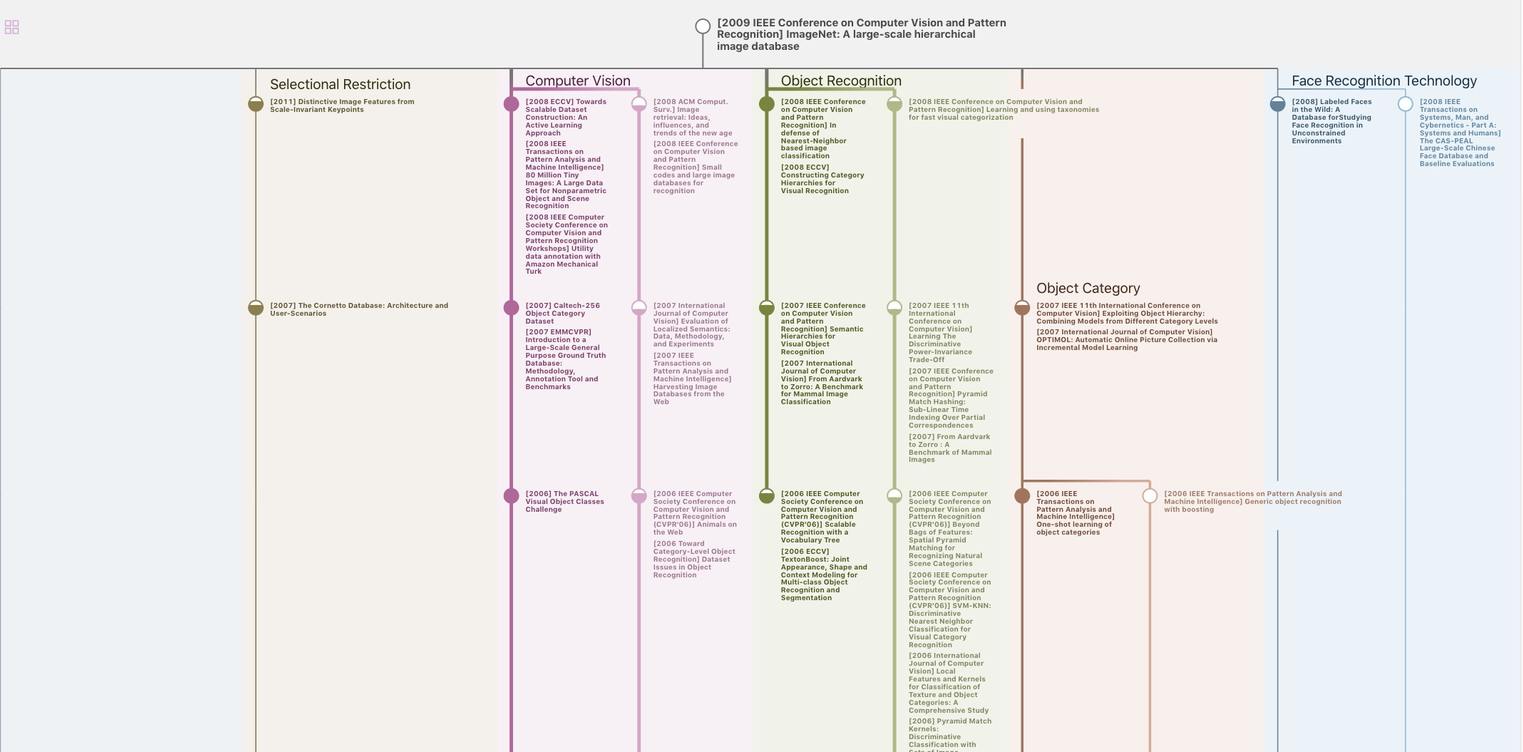
生成溯源树,研究论文发展脉络
Chat Paper
正在生成论文摘要