An Optimized Ensemble Model for COVID Detection
2022 International Conference on Machine Learning, Computer Systems and Security (MLCSS)(2022)
摘要
The entire world has gone through a pandemic situation due to the spread of novel corona virus. In this paper, the authors have proposed an ensemble learning model for the classification of the subjects to be infected by coronavirus. For this purpose, five types of symptoms are considered. The dataset contains 2889 samples with six attributes and is collected from the Kaggle database. Three different types of classifiers such as Support vector machine (SVM), Gradient boosting, and extreme gradient boosting (XGBoost) are considered for classification purposes. For improving the learning strategy and performance of the proposed models subjected to accuracy, the learning rates are varied for each node of the tree-based ensemble classifiers. Also, the hyperparameters of the XGBoost model are optimized by applying the Bayesian optimization (BO) technique. The best accuracy in SVM classifier is found as 91.69%. 96.58% accuracy is obtained in the modified gradient boosting model. The optimized XGBoost model is providing 100% accuracy which is better than other.
更多查看译文
关键词
Coronavirus,Classification,Ensemble learning,Gradient boosting,XGBoost,BO
AI 理解论文
溯源树
样例
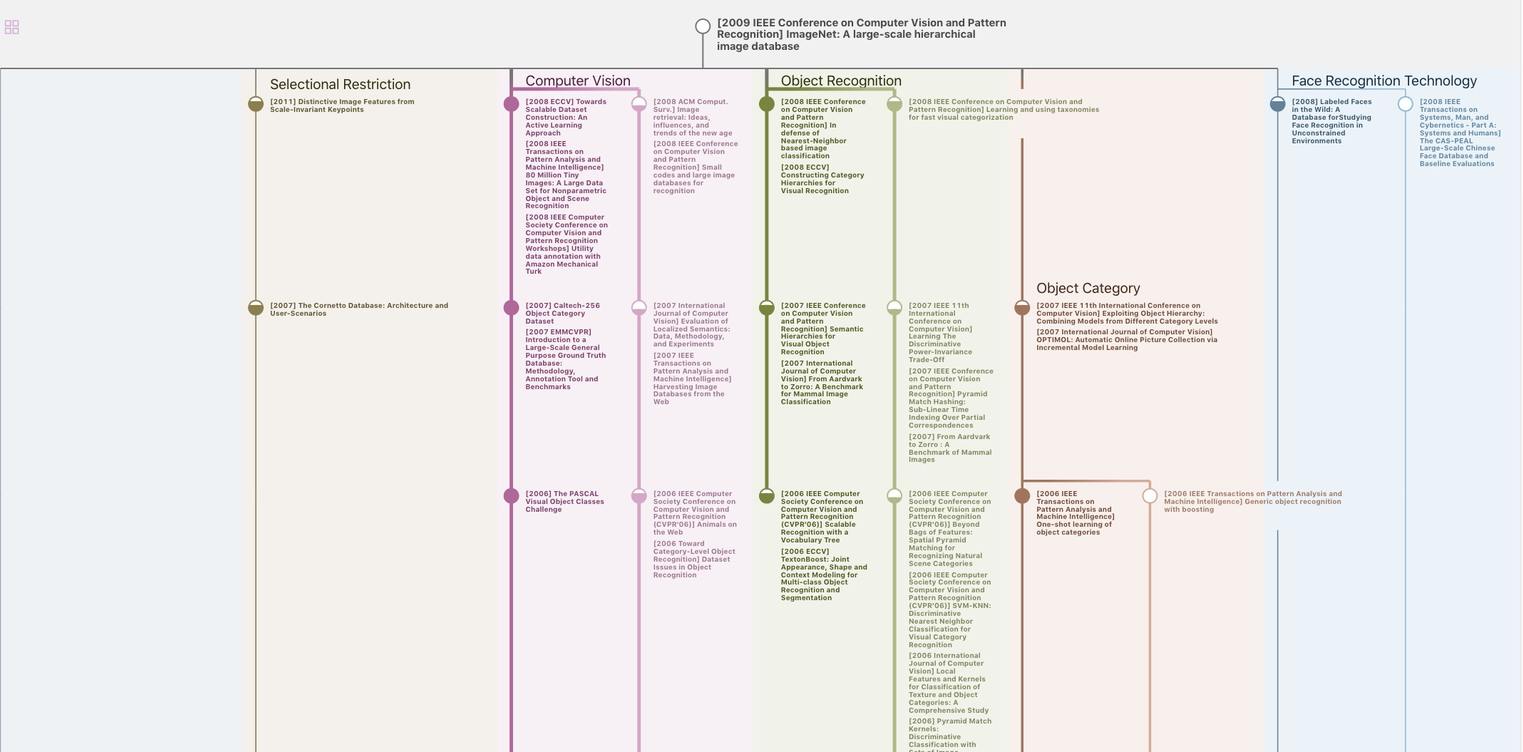
生成溯源树,研究论文发展脉络
Chat Paper
正在生成论文摘要