Enhancing Motor Imagery EEG Signal Classification with Simplified GoogLeNet
2023 11th International Winter Conference on Brain-Computer Interface (BCI)(2023)
Abstract
The brain-computer interface is a novel tool for interaction between the brain and external devices, and is an important tool at the forefront of international brain science research. Motor imagery does not require external stimulation and is one of the most widely used BCI paradigms, and is now widely used to address the treatment of neurological impairments such as paralysis, stroke, and acromegaly. Electrophysiological source imaging is a technique based on the observation of electromagnetic signals from the cerebral cortex, reflecting the electrophysiological activity of brain nerve cells on the scalp surface of the brain. The wavelet transform, on the other hand, is commonly used for the processing of non-smooth signals and aims to extract excellent signal features. This paper combines these two algorithms as feature extraction methods. In recent years, deep learning has been increasingly combined with MI-EEG-based BCI. GoogLeNet is one of the most classical convolutional neural networks and has made a splash in ImageNet image recognition. However, using GoogLeNet to train the BCI public dataset BCI IV IIa and dataset HGD has shown problems such as long training time, overfitting, and gradient disappearance. In response, this paper proposes an improved and streamlined version of GoogLeNet for training based on this dataset to achieve an overall improvement in accuracy, training time, number of parameters, and other indicators. It is useful for the realistic application of MI-based BCI systems.
MoreTranslated text
Key words
brain computer interface,motor imagery,EEG,GoogLeNet.
AI Read Science
Must-Reading Tree
Example
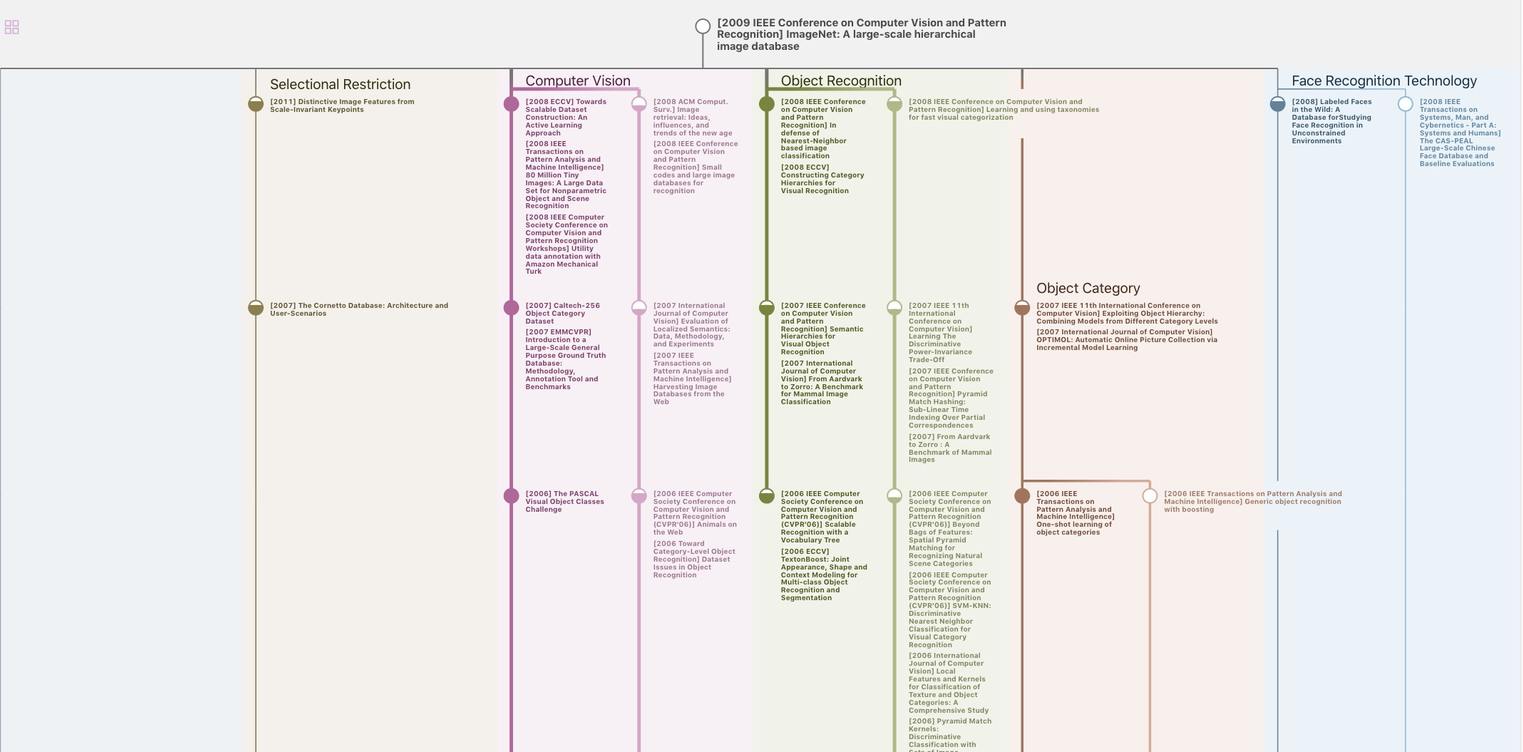
Generate MRT to find the research sequence of this paper
Chat Paper
Summary is being generated by the instructions you defined