UltraBD: Backdoor Attack against Automatic Speaker Verification Systems via Adversarial Ultrasound
2022 IEEE 28th International Conference on Parallel and Distributed Systems (ICPADS)(2023)
摘要
Automatic speaker verification (ASV) systems have been widely applied in voice user interfaces to conduct person identification and access control via voiceprints. A typical ASV system consists of three stages, i.e., training, enrollment, and verification. Previous work has revealed that the ASV system can be bypassed at the training stage by backdoor attacks and at the verification stage by adversarial example attacks. In this paper, we propose a new type of backdoor attack aimed at the enrollment stage via adversarial ultrasound, named UltraBD, which is highly imperceptible, synchronization-free, and content-independent. By simultaneously injecting the ultrasound backdoor examples when the legitimate user initiates the enrollment, the polluted voiceprints stored in the ASV systems grant access to both the legitimate user and the adversary with relatively high confidence. Despite the challenges, i.e., when, what, and how the legitimate user articulates at the enrollment stage can be remarkably unpredictable and various, we managed to launch UltraBD by augmenting the generation and optimization process of the ultrasound backdoor examples with the randomness of synchronous time and relative amplitude ratio. Furthermore, we optimize the modulation mechanism of adversarial ultrasound by tuning the baseband signal on limited signal frequency points to improve its robustness in the physical world setting. We validate UltraBD on two common datasets together with two open-source ASV models. Results show that UltraBD can be robust to various configurations, e.g., different speakers and utterance content. In sum, our attack calls attention to a new attack surface of ASV systems and sheds light on its fundamental mechanisms.
更多查看译文
关键词
backdoor attack,automatic speaker verification,adversarial ultrasound.
AI 理解论文
溯源树
样例
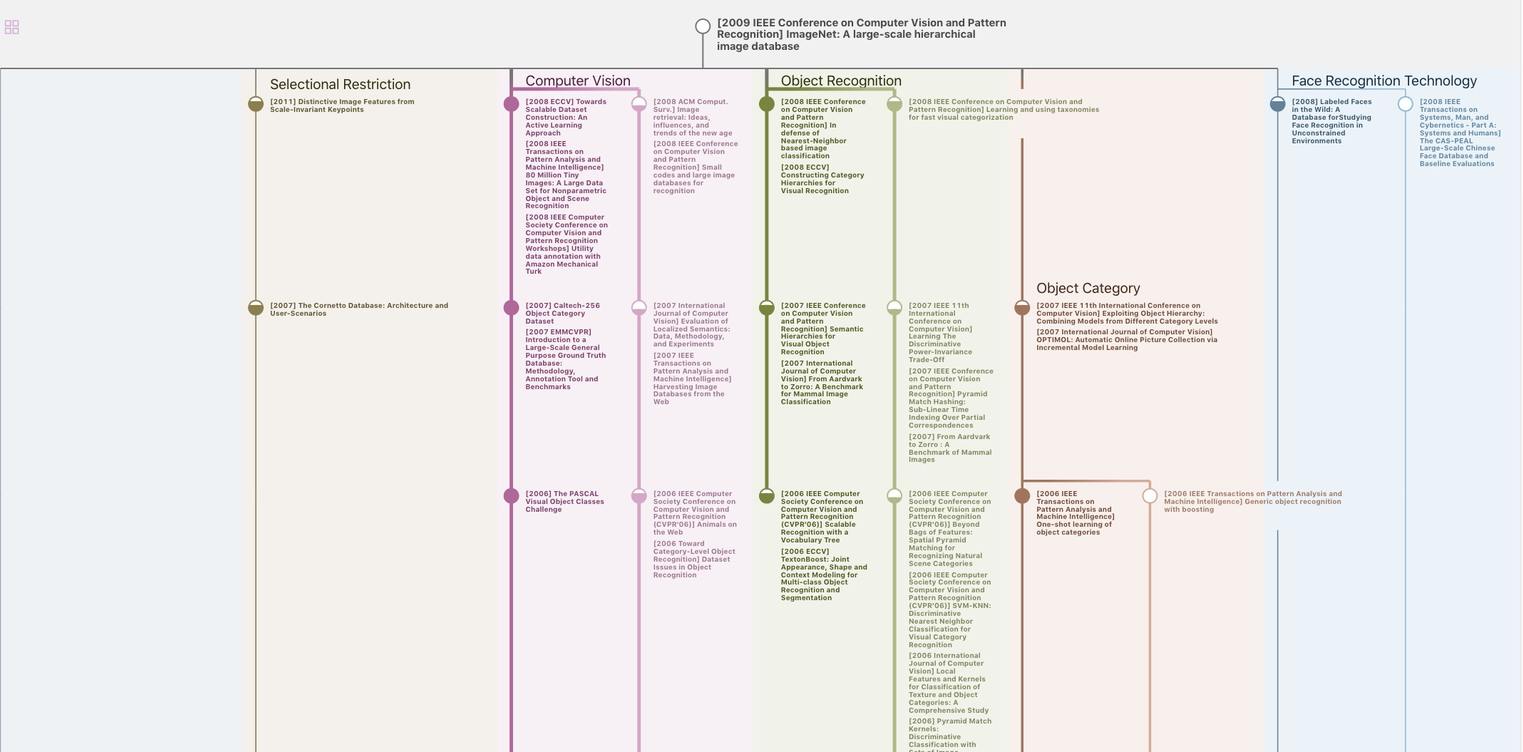
生成溯源树,研究论文发展脉络
Chat Paper
正在生成论文摘要