Multi-Source Ensemble Learning With Acoustic Spectrum Analysis for Fault Perception of Direct-Buried Transformer Substations
IEEE Transactions on Industry Applications(2023)
摘要
The tremendous growth of electrical loads results in the capacity increase and underground installation of power distribution infrastructures due to the shortage of urban land resources. However, the closed and narrow underground space of direct-buried transformer substation (DBTS) brings great challenges to its fault perception. This article proposes an adaptive acoustic spectrum analysis method with refined composite multiscale dispersion entropy to extract informative fault-related time-frequency vocal features using the singular-value thresholded variable mode decomposition for facilitating fault diagnosis of DBTS. Then, a time-series generative adversarial network based fault data augmentation model is presented to enrich the training dataset while preserving temporal dynamics of the original fault data for boosting the generalization ability of DBTS fault perception. Furthermore, a multi-source ensemble learning strategy is developed to integrate heterogeneous sub-classifiers based on the dynamic weighted fusion of electrical monitoring data and acoustic signals for promoting fault diagnosis accuracy of DBTS. Comparative studies have been investigated to demonstrate the superior performance of the proposed multi-source ensemble learning strategy in improving the fault diagnosis accuracy of DBTS.
更多查看译文
关键词
Acoustic analysis,fault perception,machine learning,power distribution system,transformer substation
AI 理解论文
溯源树
样例
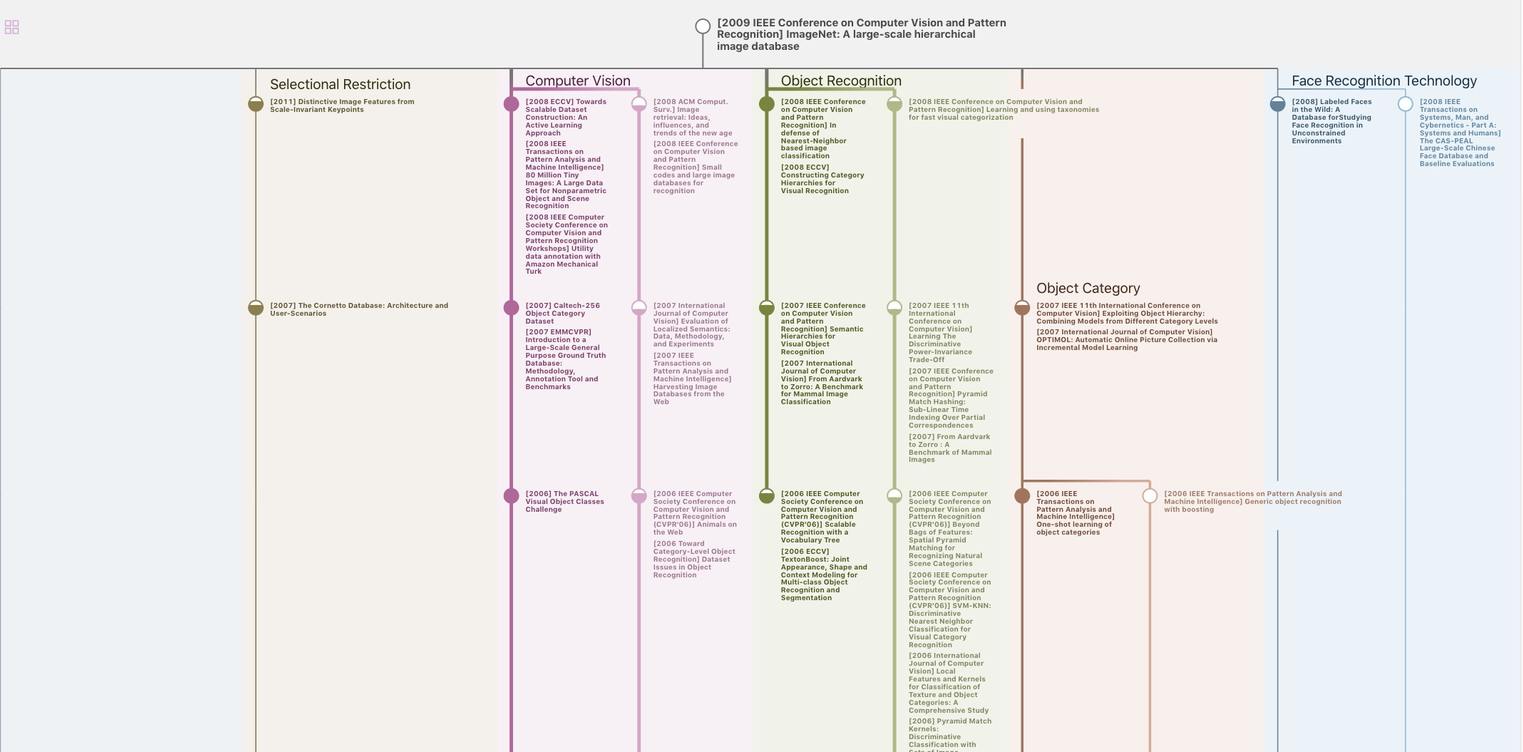
生成溯源树,研究论文发展脉络
Chat Paper
正在生成论文摘要