Self-Supervised Category-Level 6D Object Pose Estimation With Optical Flow Consistency
IEEE Robotics and Automation Letters(2023)
摘要
Category-level 6D object pose estimation aims at determining the pose of an object of a given category. Most current state-of-the-art methods require a significant amount of real training data to supervise their models. Moreover, annotating the 6D pose is very time consuming, error-prone, and it does not scale well to a large amount of object classes. Therefore, a handful of methods have recently been proposed to use unlabelled data to establish weak supervision. In this letter we propose a self-supervised method that leverages the 2D optical flow as a proxy for supervising the 6D pose. To this purpose, we estimate the 2D optical flow between consecutive frames based on the pose estimation. Then, we harness an off-the-shelf optical flow method to enable weak supervision using a 2D-3D optical flow based consistency loss. Experiments show that our approach for self-supervised learning yields state-of-the-art performance on the NOCS benchmark, and it reaches comparable results with some fully-supervised approaches.
更多查看译文
关键词
Object detection,segmentation and categorization,deep learning for visual perception
AI 理解论文
溯源树
样例
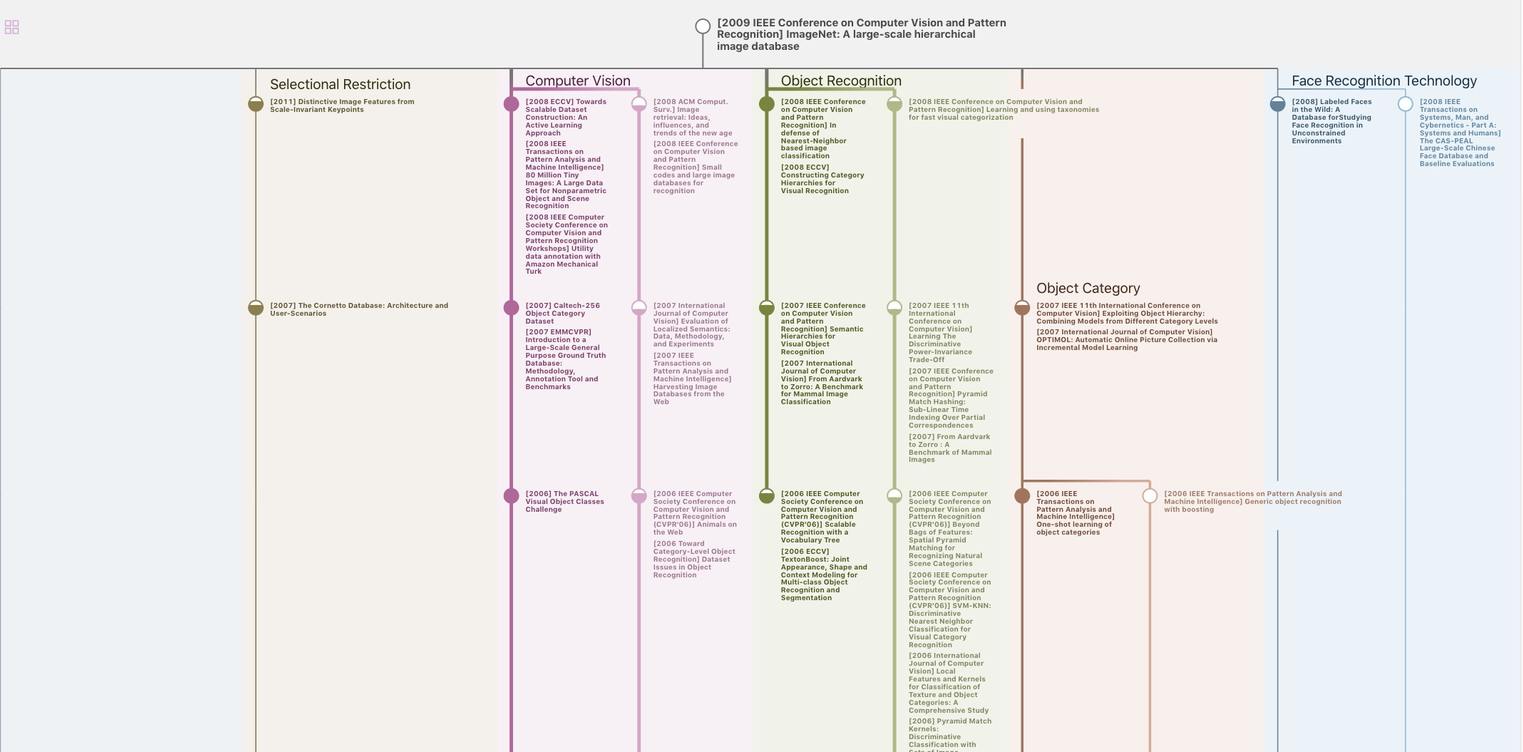
生成溯源树,研究论文发展脉络
Chat Paper
正在生成论文摘要