Canopy Scale High-Resolution Forest Biophysical Parameter (LAI, fAPAR, and fCover) Retrieval Through Machine Learning and Cloud Computation Approach
2023 International Conference on Machine Intelligence for GeoAnalytics and Remote Sensing (MIGARS)(2023)
Abstract
High-resolution Forest biophysical parameter estimation is crucial to understand forest structural and functional variability. Moreover, mapping high-resolution biophysical products is significant to capture accurate forest carbon fluxes and understand seasonal variability. The existing biophysical products are coarse in spatial resolution and unable to capture intra-annual variability. In this study, we proposed a random forest machine learning approach embedded in Google Earth Engine to retrieve three major forest biophysical parameters. The training samples were distributed in a 70:30 ratio for model training and validation. The outcome of the work shows promising results that hold a good agreement with SNAP-derived biophysical variables, whereas the agreement is moderate-to-poor for MODIS and VIIRS biophysical products. As shown in the model, NIR performs as the most sensitive band for forest biophysical estimation. We believe our proposed approach would significantly support the existing methods for improved high-resolution forest biophysical component estimation.
MoreTranslated text
Key words
Forest,LAI,Random Forest,Biophysical,India
AI Read Science
Must-Reading Tree
Example
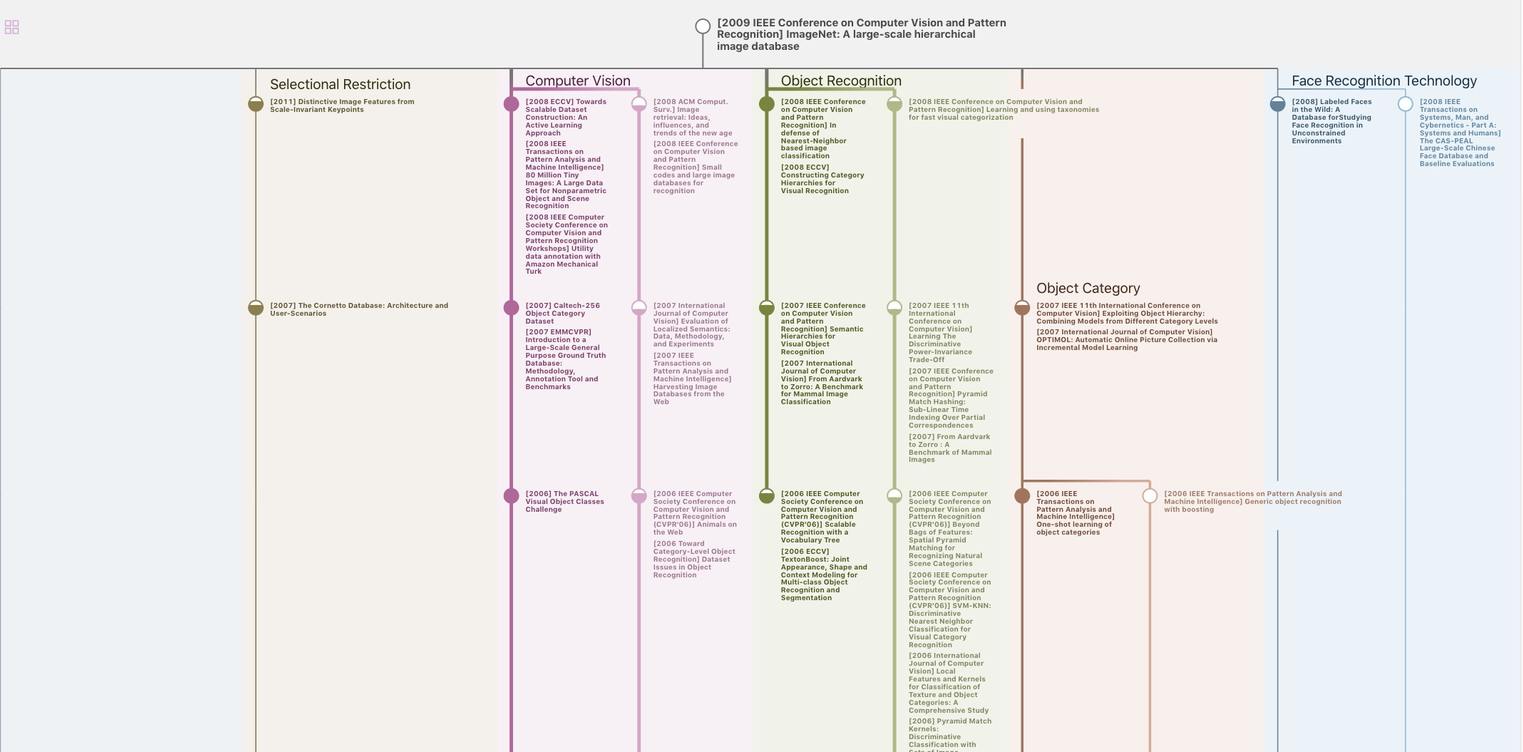
Generate MRT to find the research sequence of this paper
Chat Paper
Summary is being generated by the instructions you defined