DCENet: A dynamic correlation evolve network for short-term traffic prediction
Physica A: Statistical Mechanics and its Applications(2023)
Abstract
Graph neural networks (GNNs) have been extensively employed in traffic prediction tasks due to their excellent capturing capabilities of spatial dependence. However, the majority of GNN-based approaches tend to employ static graphs, whereas they evolve over time and vary dynamics in real-world traffic situations. It is challenging to capture the dynamic spatial-temporal evolution characteristics of traffic data. To address this problem, we propose a dynamic correlation evolve network (DCENet) for short-term traffic prediction. To be specific, we develop a dynamic correlation self-attention (DCSA) module, which captures dynamic node associations adaptively. In this way, the model acquires new node embedding features without explicitly constructing a new graph structure. Then, an evolution encoder-decoder (EED) module is built to learn the interactions of dynamic features and output future traffic states. The experiments are conducted on two real-world datasets, and the results show that the DCENet outperformers baseline models for most of the cases.(c) 2023 Elsevier B.V. All rights reserved.
MoreTranslated text
Key words
Traffic prediction,Dynamic correlations,Self-attention,Encoder-Decoder
AI Read Science
Must-Reading Tree
Example
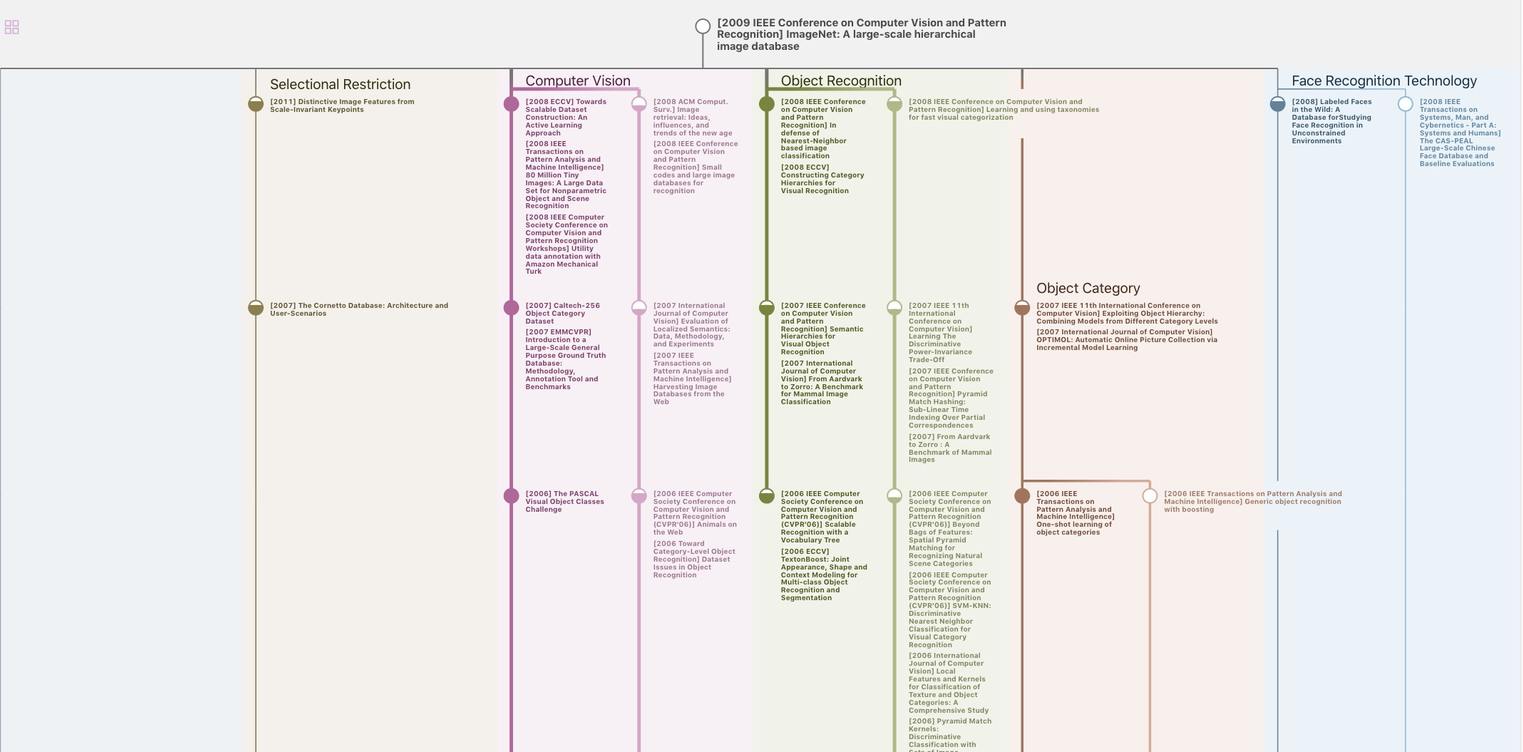
Generate MRT to find the research sequence of this paper
Chat Paper
Summary is being generated by the instructions you defined